书目名称 | Machine Learning in Social Networks | 副标题 | Embedding Nodes, Edg | 编辑 | Manasvi Aggarwal,M.N. Murty | 视频video | | 概述 | Highlights the understanding of complex systems in different domains including health, education, agriculture, and transportation.Combines both conventional machine learning (ML) and deep learning (DL | 丛书名称 | SpringerBriefs in Applied Sciences and Technology | 图书封面 | 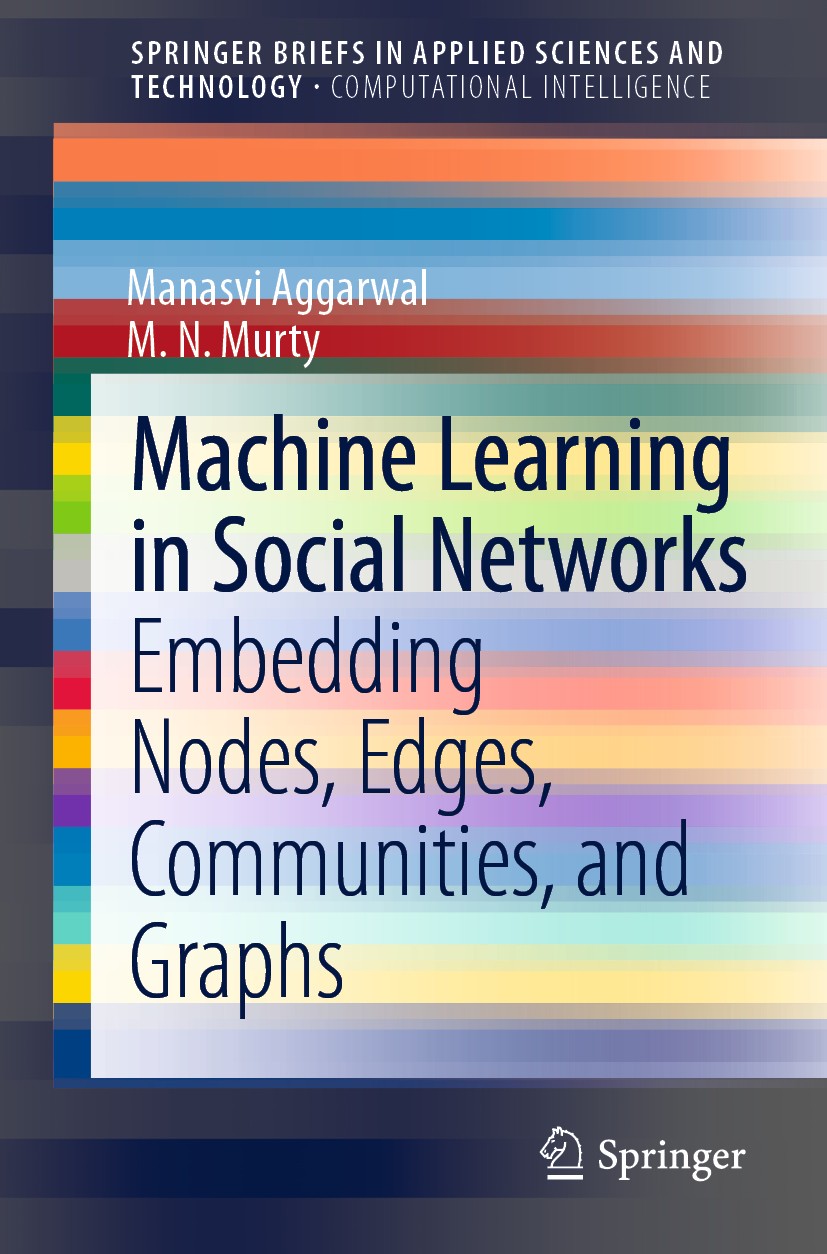 | 描述 | .This book deals with network representation learning. It deals with embedding nodes, edges, subgraphs and graphs. There is a growing interest in understanding complex systems in different domains including health, education, agriculture and transportation. Such complex systems are analyzed by modeling, using networks that are aptly called complex networks. Networks are becoming ubiquitous as they can represent many real-world relational data, for instance, information networks, molecular structures, telecommunication networks and protein–protein interaction networks. Analysis of these networks provides advantages in many fields such as recommendation (recommending friends in a social network), biological field (deducing connections between proteins for treating new diseases) and community detection (grouping users of a social network according to their interests) by leveraging the latent information of networks. An active and important area ofcurrent interest is to come out with algorithms that learn features by embedding nodes or (sub)graphs into a vector space. These tasks come under the broad umbrella of representation learning. A representation learning model learns a mapping | 出版日期 | Book 2021 | 关键词 | Network embedding; Deep Learning (DL); Neural Networks; Network representation learning; Embedded graphs | 版次 | 1 | doi | https://doi.org/10.1007/978-981-33-4022-0 | isbn_softcover | 978-981-33-4021-3 | isbn_ebook | 978-981-33-4022-0Series ISSN 2191-530X Series E-ISSN 2191-5318 | issn_series | 2191-530X | copyright | The Author(s), under exclusive license to Springer Nature Singapore Pte Ltd. 2021 |
The information of publication is updating
|
|