书目名称 | Machine Learning in Medical Imaging |
副标题 | Second International |
编辑 | Kenji Suzuki,Fei Wang,Pingkun Yan |
视频video | |
概述 | State-of-the-art research.Fast-track conference proceedings.Unique visibility |
丛书名称 | Lecture Notes in Computer Science |
图书封面 | 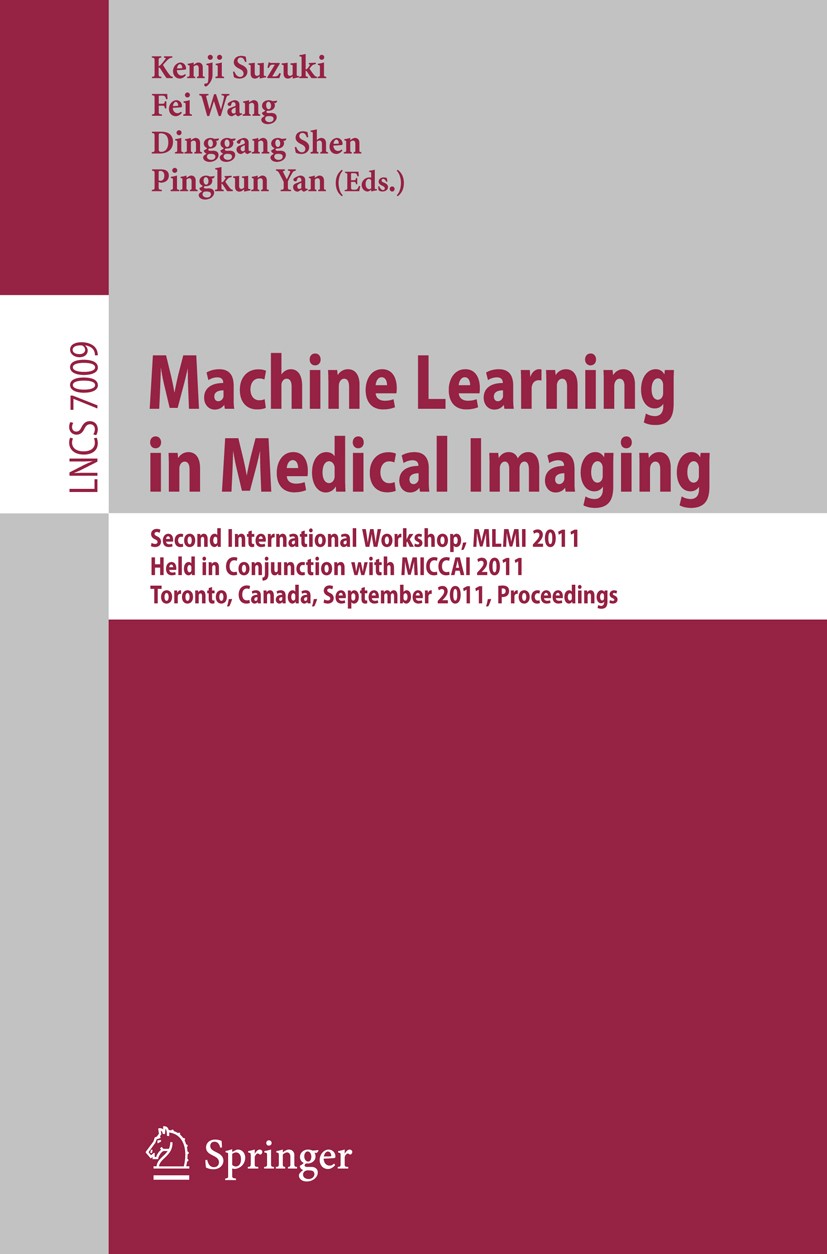 |
描述 | This book constitutes the refereed proceedings of the Second International Workshop on Machine Learning in Medical Imaging, MLMI 2011, held in conjunction with MICCAI 2011, in Toronto, Canada, in September 2011. The 44 revised full papers presented were carefully reviewed and selected from 74 submissions. The papers focus on major trends in machine learning in medical imaging aiming to identify new cutting-edge techniques and their use in medical imaging. |
出版日期 | Conference proceedings 2011 |
关键词 | artificial neural network; computer assisted surgery; graphical model; multi-modality; support vector ma |
版次 | 1 |
doi | https://doi.org/10.1007/978-3-642-24319-6 |
isbn_softcover | 978-3-642-24318-9 |
isbn_ebook | 978-3-642-24319-6Series ISSN 0302-9743 Series E-ISSN 1611-3349 |
issn_series | 0302-9743 |
copyright | Springer-Verlag GmbH Berlin Heidelberg 2011 |