书目名称 | Machine Learning in Document Analysis and Recognition |
编辑 | Simone Marinai,Hiromichi Fujisawa |
视频video | |
概述 | Presents applications and learning algorithms for Document Image Analysis and Recognition (DIAR).Identifies good practices for the use of learning strategies in DIAR.Includes supplementary material: |
丛书名称 | Studies in Computational Intelligence |
图书封面 | 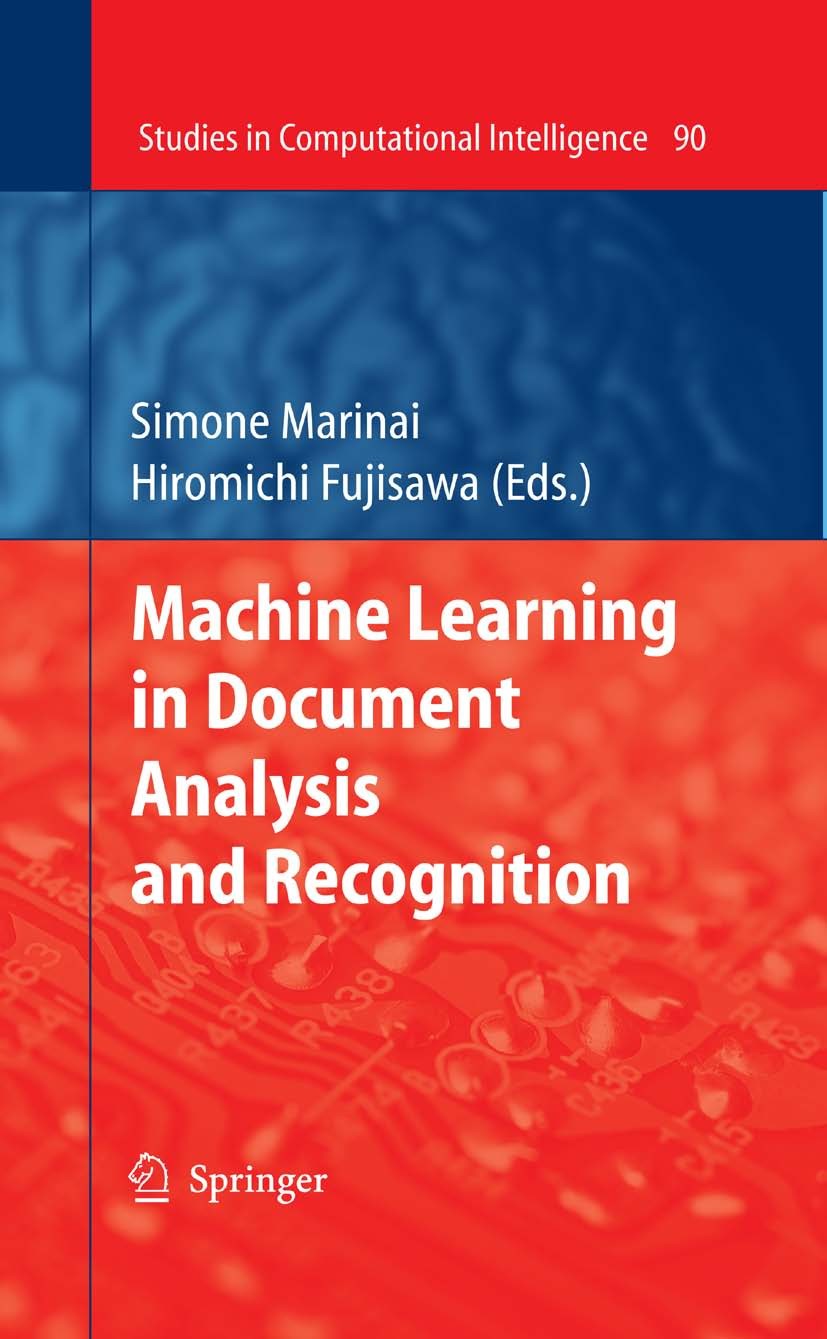 |
描述 | The objective of Document Analysis and Recognition (DAR) is to recognize the text and graphicalcomponents of a document and to extract information. With ?rst papers dating back to the 1960’s, DAR is a mature but still gr- ing research?eld with consolidated and known techniques. Optical Character Recognition (OCR) engines are some of the most widely recognized pr- ucts of the research in this ?eld, while broader DAR techniques are nowadays studied and applied to other industrial and o?ce automation systems. In the machine learning community, one of the most widely known - search problems addressed in DAR is recognition of unconstrained handwr- ten characters which has been frequently used in the past as a benchmark for evaluating machine learning algorithms, especially supervised classi?ers. However, developing a DAR system is a complex engineering task that involves the integration of multiple techniques into an organic framework. A reader may feel that the use of machine learning algorithms is not approp- ate for other DAR tasks than character recognition. On the contrary, such algorithms have been massively used for nearly all the tasks in DAR. With large emphasis being devoted t |
出版日期 | Book 2008 |
关键词 | Document Image Analysis and Recognition (DIAR); Learning Strategies; algorithm; algorithms; calculus; cla |
版次 | 1 |
doi | https://doi.org/10.1007/978-3-540-76280-5 |
isbn_softcover | 978-3-642-09511-5 |
isbn_ebook | 978-3-540-76280-5Series ISSN 1860-949X Series E-ISSN 1860-9503 |
issn_series | 1860-949X |
copyright | Springer-Verlag Berlin Heidelberg 2008 |