书目名称 | Machine Learning for Dynamic Software Analysis: Potentials and Limits |
副标题 | International Dagstu |
编辑 | Amel Bennaceur,Reiner Hähnle,Karl Meinke |
视频video | |
概述 | Written by international experts.Presents the state of the art and suggests new directions and collaborations for future research.Gives an overview of the machine learning techniques that can be used |
丛书名称 | Lecture Notes in Computer Science |
图书封面 | 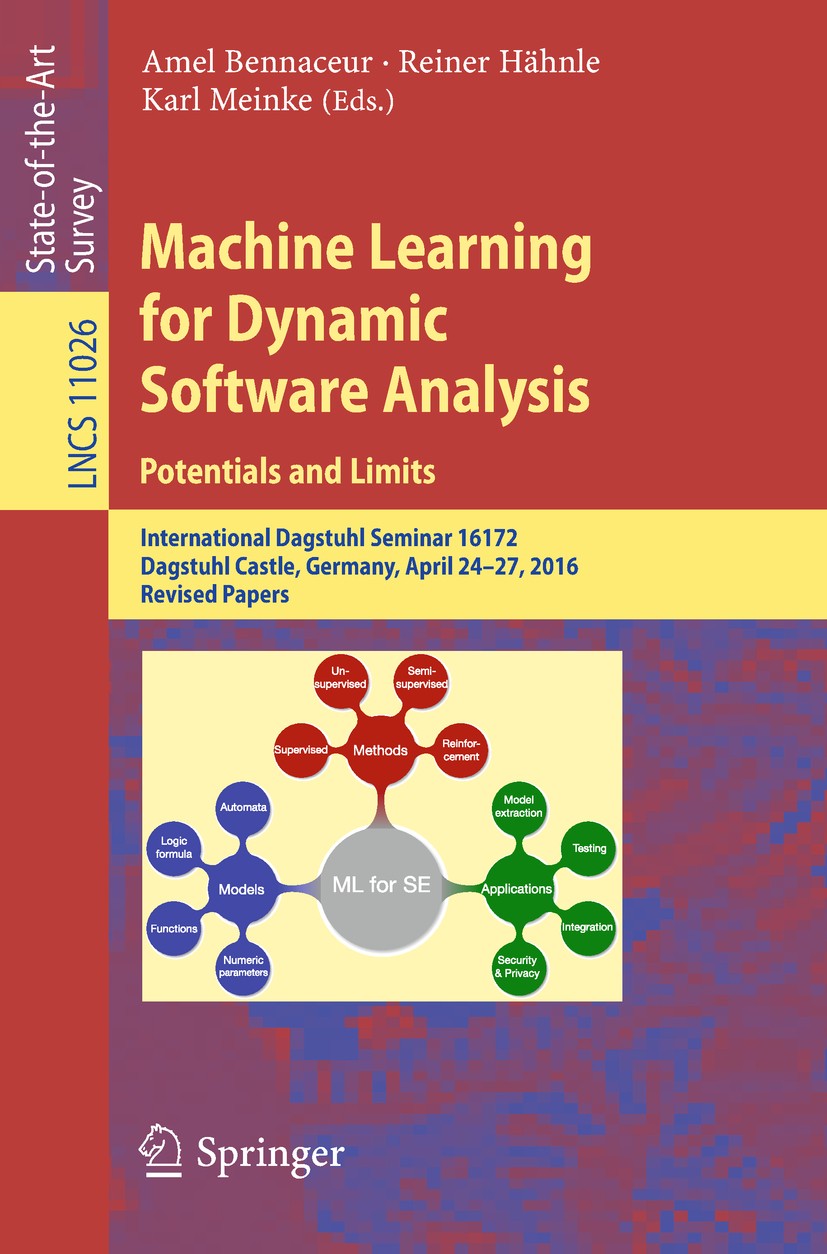 |
描述 | Machine learning of software artefacts is an emerging area of interaction between the machine learning and software analysis communities. Increased productivity in software engineering relies on the creation of new adaptive, scalable tools that can analyse large and continuously changing software systems. These require new software analysis techniques based on machine learning, such as learning-based software testing, invariant generation or code synthesis. Machine learning is a powerful paradigm that provides novel approaches to automating the generation of models and other essential software artifacts. This volume originates from a Dagstuhl Seminar entitled "Machine Learning for Dynamic Software Analysis: Potentials and Limits” held in April 2016. The seminar focused on fostering a spirit of collaboration in order to share insights and to expand and strengthen the cross-fertilisation between the machine learning and software analysis communities. The book provides an overview of the machine learning techniques that can be used for software analysis and presents example applications of their use. Besides an introductory chapter, the book is structured into three parts: testing |
出版日期 | Book 2018 |
关键词 | Active learning; Artificial intelligence; Automated static analysis; Computing methodologies; Dynamic an |
版次 | 1 |
doi | https://doi.org/10.1007/978-3-319-96562-8 |
isbn_softcover | 978-3-319-96561-1 |
isbn_ebook | 978-3-319-96562-8Series ISSN 0302-9743 Series E-ISSN 1611-3349 |
issn_series | 0302-9743 |
copyright | Springer Nature Switzerland AG 2018 |