书目名称 | Machine Learning for Cyber-Physical Systems |
副标题 | Selected papers from |
编辑 | Oliver Niggemann,Jürgen Beyerer,Christian Kühnert |
视频video | |
概述 | Includes the full proceedings of the 2023 ML4CPS – Machine Learning for Cyber-Physical Systems Conference.Presents recent and new advances in automated machine learning methods.Combines machine learni |
丛书名称 | Technologien für die intelligente Automation |
图书封面 | 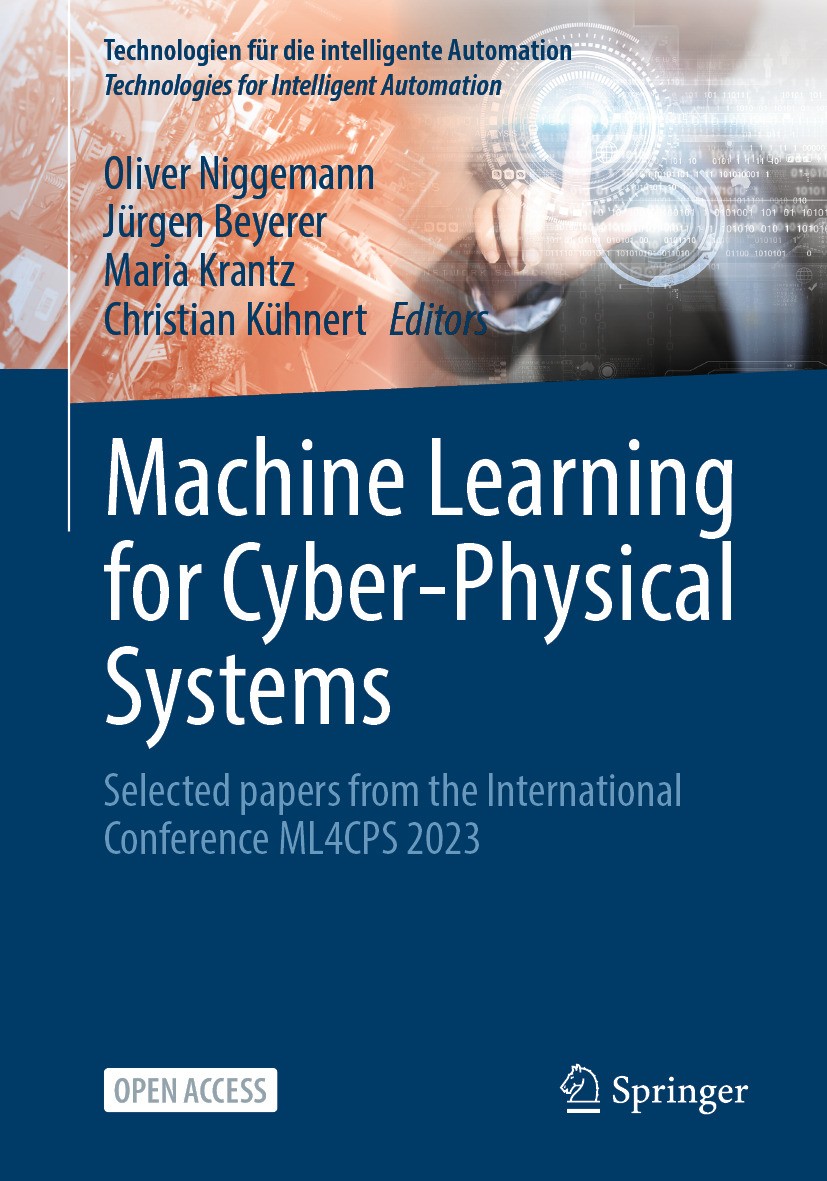 |
描述 | .This open access proceedings presents new approaches to Machine Learning for Cyber-Physical Systems, experiences and visions. It contains some selected papers from the international Conference ML4CPS – Machine Learning for Cyber-Physical Systems, which was held in Hamburg (Germany), March 29th to 31st, 2023. .Cyber-physical systems are characterized by their ability to adapt and to learn: They analyze their environment and, based on observations, they learn patterns, correlations and predictive models. Typical applications are condition monitoring, predictive maintenance, image processing and diagnosis. Machine Learning is the key technology for these developments..This is an open access book.. |
出版日期 | Conference proceedings‘‘‘‘‘‘‘‘ 2024 |
关键词 | Cyber-physical systems; Neural networks; Computer Science; Network architecture; Automatic validation; Ma |
版次 | 1 |
doi | https://doi.org/10.1007/978-3-031-47062-2 |
isbn_softcover | 978-3-031-47061-5 |
isbn_ebook | 978-3-031-47062-2Series ISSN 2522-8579 Series E-ISSN 2522-8587 |
issn_series | 2522-8579 |
copyright | The Editor(s) (if applicable) and The Author(s) 2024 |