书目名称 | Machine Learning for Computer Scientists and Data Analysts | 副标题 | From an Applied Pers | 编辑 | Setareh Rafatirad,Houman Homayoun,Sai Manoj Puduko | 视频video | | 概述 | Describes traditional as well as advanced machine learning algorithms.Enables students to learn which algorithm is most appropriate for the data being handled.Includes numerous, practical case-studies | 图书封面 | 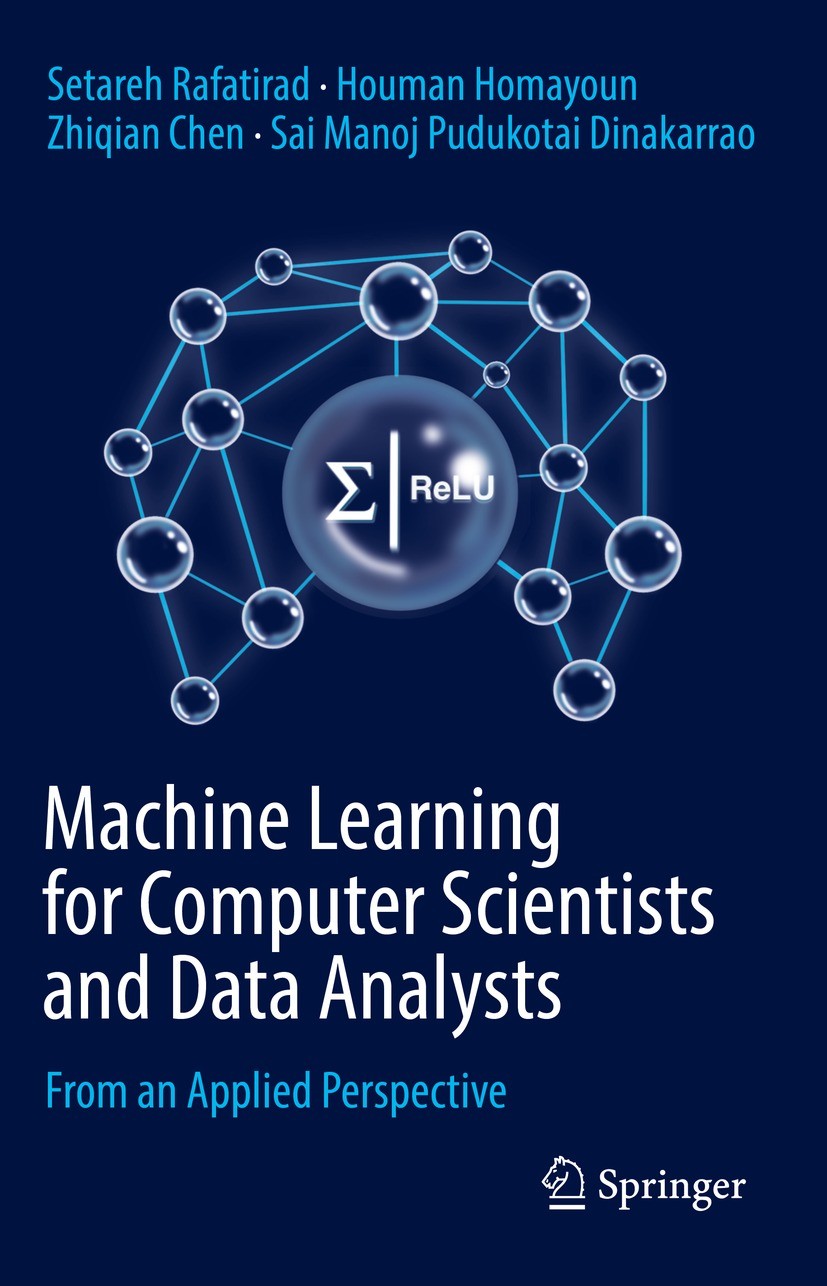 | 描述 | .This textbook introduces readers to the theoretical aspects of machine learning (ML) algorithms, starting from simple neuron basics, through complex neural networks, including generative adversarial neural networks and graph convolution networks. Most importantly, this book helps readers to understand the concepts of ML algorithms and enables them to develop the skills necessary to choose an apt ML algorithm for a problem they wish to solve. In addition, this book includes numerous case studies, ranging from simple time-series forecasting to object recognition and recommender systems using massive databases. Lastly, this book also provides practical implementation examples and assignments for the readers to practice and improve their programming capabilities for the ML applications.. | 出版日期 | Textbook 2022 | 关键词 | Machine Learning Textbook; Deep Learning Textbook; AI Textbook; Machine Learning in Big Data; Machine Le | 版次 | 1 | doi | https://doi.org/10.1007/978-3-030-96756-7 | isbn_softcover | 978-3-030-96758-1 | isbn_ebook | 978-3-030-96756-7 | copyright | The Editor(s) (if applicable) and The Author(s), under exclusive license to Springer Nature Switzerl |
The information of publication is updating
|
|