书目名称 | Machine Learning and Knowledge Discovery in Databases. Research Track | 副标题 | European Conference, | 编辑 | Albert Bifet,Jesse Davis,Indrė Žliobaitė | 视频video | | 丛书名称 | Lecture Notes in Computer Science | 图书封面 | 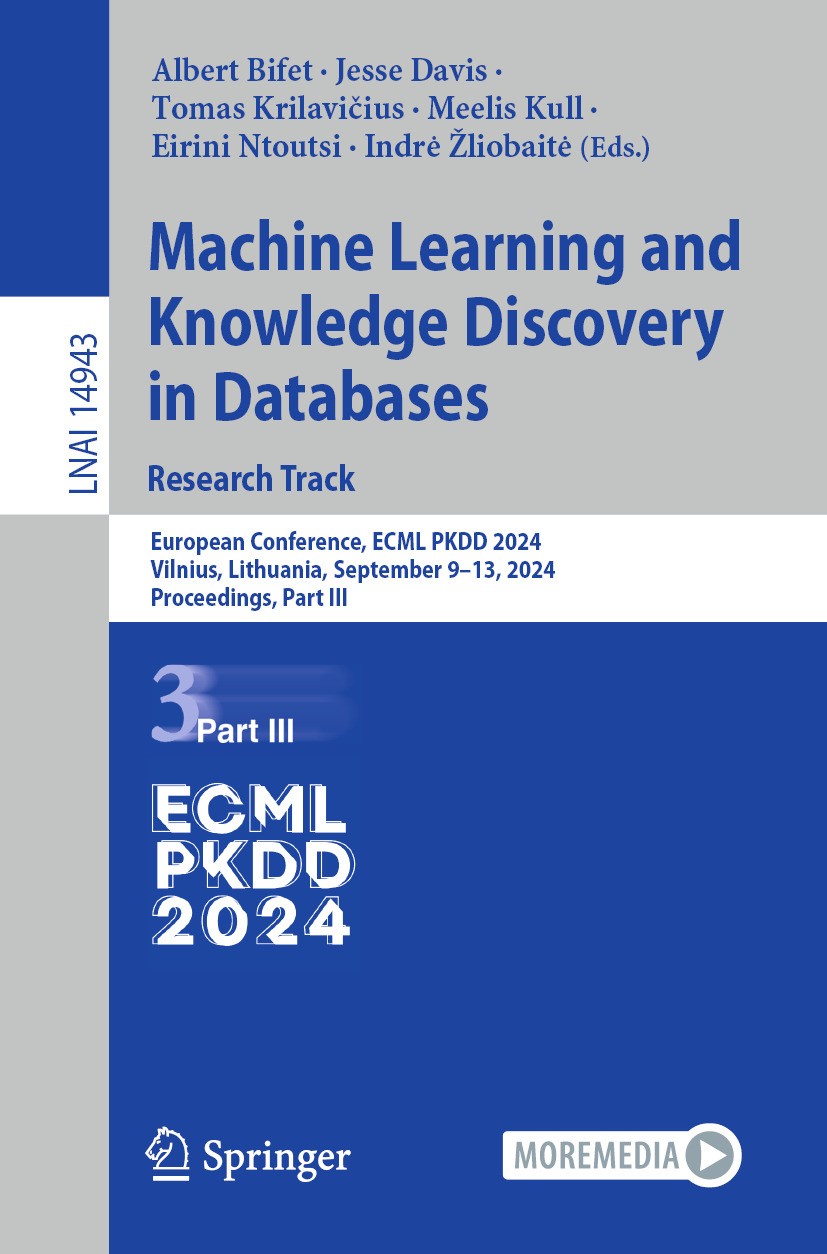 | 描述 | .This multi-volume set, LNAI 14941 to LNAI 14950, constitutes the refereed proceedings of the European Conference on Machine Learning and Knowledge Discovery in Databases, ECML PKDD 2024, held in Vilnius, Lithuania, in September 2024... ..The papers presented in these proceedings are from the following three conference tracks: -..Research Track:. The 202 full papers presented here, from this track, were carefully reviewed and selected from 826 submissions. These papers are present in the following volumes: Part I, II, III, IV, V, VI, VII, VIII... ..Demo Track: .The 14 papers presented here, from this track, were selected from 30 submissions. These papers are present in the following volume: Part VIII... ..Applied Data Science Track: .The 56 full papers presented here, from this track, were carefully reviewed and selected from 224 submissions. These papers are present in the following volumes: Part IX and Part X.. | 出版日期 | Conference proceedings 2024 | 关键词 | artificial intelligence; computer security; computer systems; computer vision; computational modelling; d | 版次 | 1 | doi | https://doi.org/10.1007/978-3-031-70352-2 | isbn_softcover | 978-3-031-70351-5 | isbn_ebook | 978-3-031-70352-2Series ISSN 0302-9743 Series E-ISSN 1611-3349 | issn_series | 0302-9743 | copyright | The Editor(s) (if applicable) and The Author(s), under exclusive license to Springer Nature Switzerl |
The information of publication is updating
|
|