书目名称 | Machine Learning and Knowledge Discovery in Databases. Research Track | 副标题 | European Conference, | 编辑 | Nuria Oliver,Fernando Pérez-Cruz,Jose A. Lozano | 视频video | | 丛书名称 | Lecture Notes in Computer Science | 图书封面 | 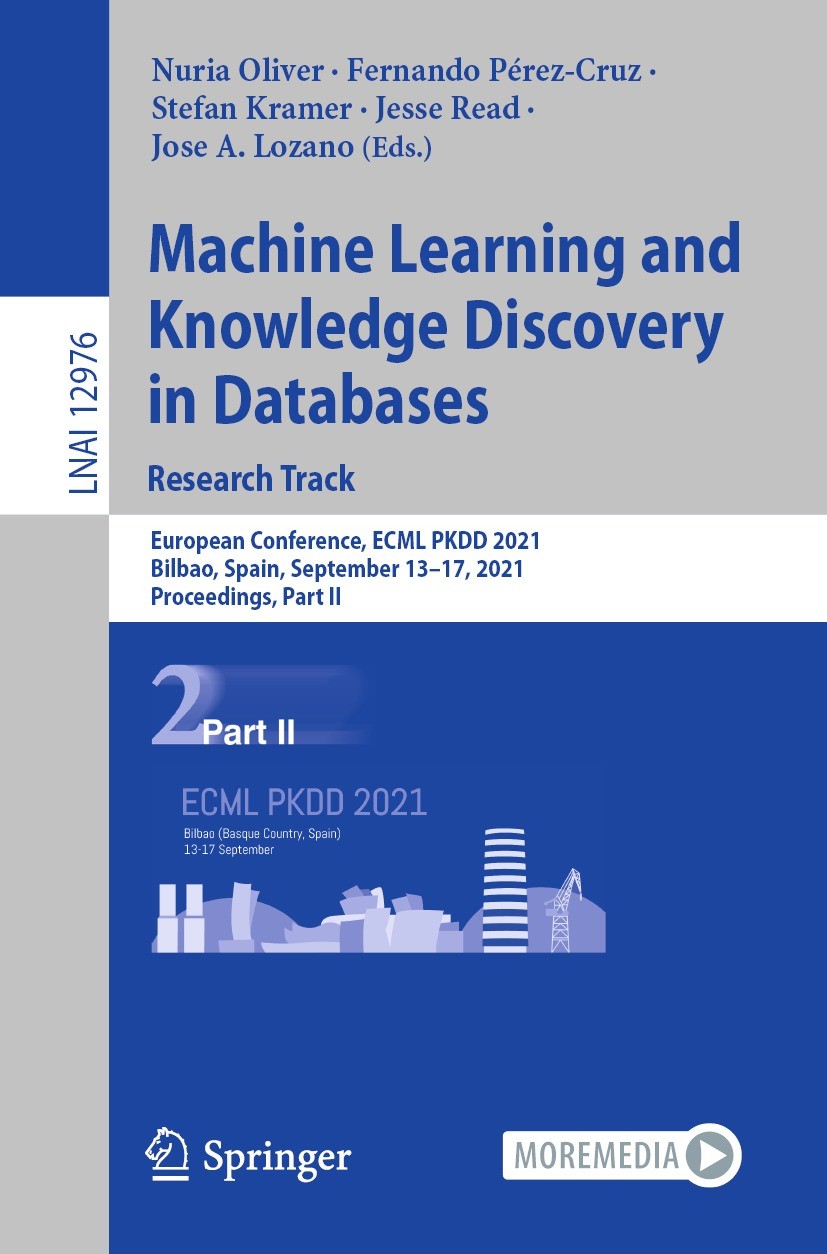 | 描述 | .The multi-volume set LNAI 12975 until 12979 constitutes the refereed proceedings of the European Conference on Machine Learning and Knowledge Discovery in Databases, ECML PKDD 2021, which was held during September 13-17, 2021. The conference was originally planned to take place in Bilbao, Spain, but changed to an online event due to the COVID-19 pandemic. .The 210 full papers presented in these proceedings were carefully reviewed and selected from a total of 869 submissions...The volumes are organized in topical sections as follows:..Research Track:..Part I:. Online learning; reinforcement learning; time series, streams, and sequence models; transfer and multi-task learning; semi-supervised and few-shot learning; learning algorithms and applications...Part II:. Generative models; algorithms and learning theory; graphs and networks; interpretation, explainability, transparency, safety...Part III: .Generative models; search and optimization; supervised learning; text mining and natural language processing; image processing, computer vision and visual analytics...Applied Data Science Track:..Part IV:. Anomaly detection and malware; spatio-temporal data; e-commerce and finance; health | 出版日期 | Conference proceedings 2021 | 关键词 | applied computing; communication systems; computer graphics; computer networks; computer security; comput | 版次 | 1 | doi | https://doi.org/10.1007/978-3-030-86520-7 | isbn_softcover | 978-3-030-86519-1 | isbn_ebook | 978-3-030-86520-7Series ISSN 0302-9743 Series E-ISSN 1611-3349 | issn_series | 0302-9743 | copyright | Springer Nature Switzerland AG 2021 |
The information of publication is updating
|
|