书目名称 | Machine Learning and Knowledge Discovery in Databases |
副标题 | European Conference, |
编辑 | Hendrik Blockeel,Kristian Kersting,Filip Železný |
视频video | |
概述 | State-of-the-art research.Up-to-date results.Unique visibility |
丛书名称 | Lecture Notes in Computer Science |
图书封面 | 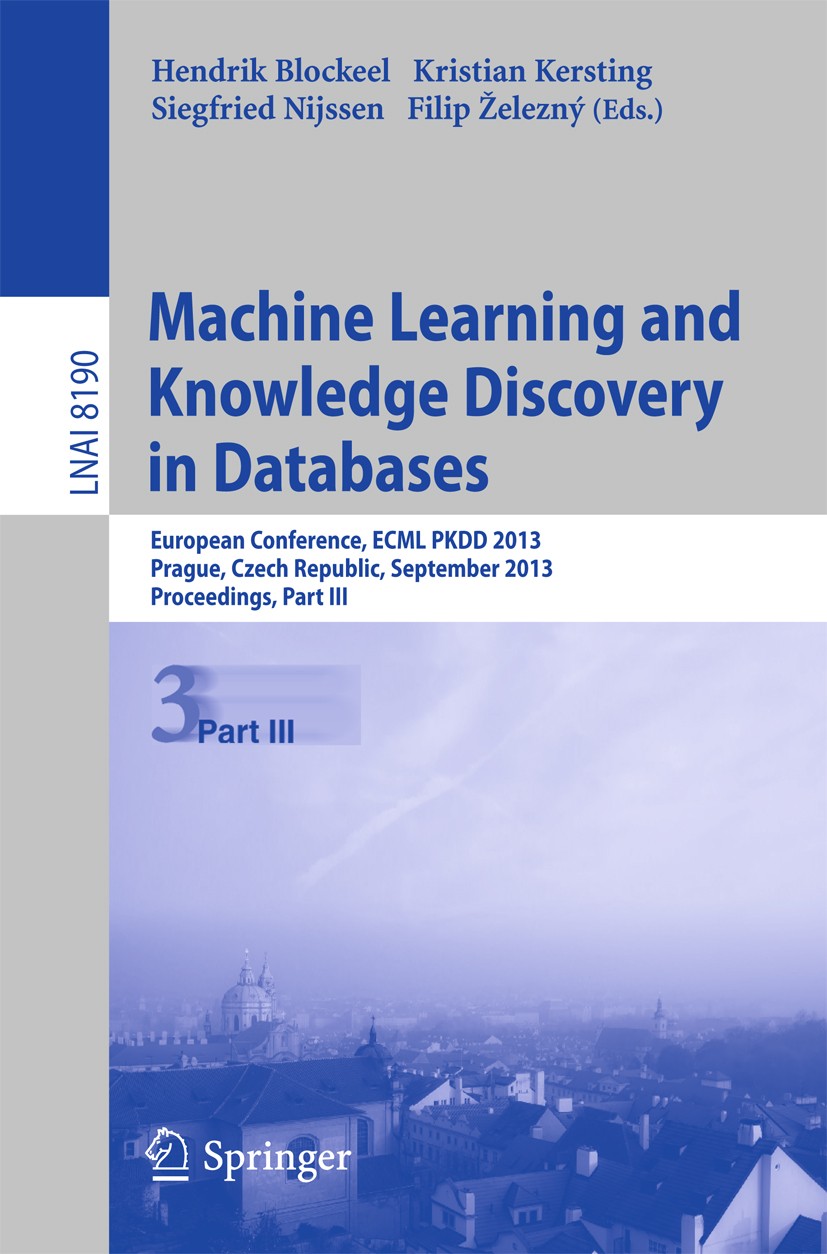 |
描述 | This three-volume set LNAI 8188, 8189 and 8190 constitutes the refereed proceedings of the European Conference on Machine Learning and Knowledge Discovery in Databases, ECML PKDD 2013, held in Prague, Czech Republic, in September 2013. The 111 revised research papers presented together with 5 invited talks were carefully reviewed and selected from 447 submissions. The papers are organized in topical sections on reinforcement learning; Markov decision processes; active learning and optimization; learning from sequences; time series and spatio-temporal data; data streams; graphs and networks; social network analysis; natural language processing and information extraction; ranking and recommender systems; matrix and tensor analysis; structured output prediction, multi-label and multi-task learning; transfer learning; bayesian learning; graphical models; nearest-neighbor methods; ensembles; statistical learning; semi-supervised learning; unsupervised learning; subgroup discovery, outlier detection and anomaly detection; privacy and security; evaluation; applications; and medical applications. |
出版日期 | Conference proceedings 2013 |
关键词 | bayesian network; data mining; graph-based methods; parallel optimization; social responsibility |
版次 | 1 |
doi | https://doi.org/10.1007/978-3-642-40994-3 |
isbn_softcover | 978-3-642-40993-6 |
isbn_ebook | 978-3-642-40994-3Series ISSN 0302-9743 Series E-ISSN 1611-3349 |
issn_series | 0302-9743 |
copyright | Springer-Verlag Berlin Heidelberg 2013 |