书目名称 | Machine Learning Models and Algorithms for Big Data Classification | 副标题 | Thinking with Exampl | 编辑 | Shan Suthaharan | 视频video | http://file.papertrans.cn/621/620410/620410.mp4 | 概述 | Addresses a new and hot field of Big Data Science and Engineering.Offers new Machine Learning techniques and solutions.Provides solutions to overcome Big Data classification problems that industries, | 丛书名称 | Integrated Series in Information Systems | 图书封面 | 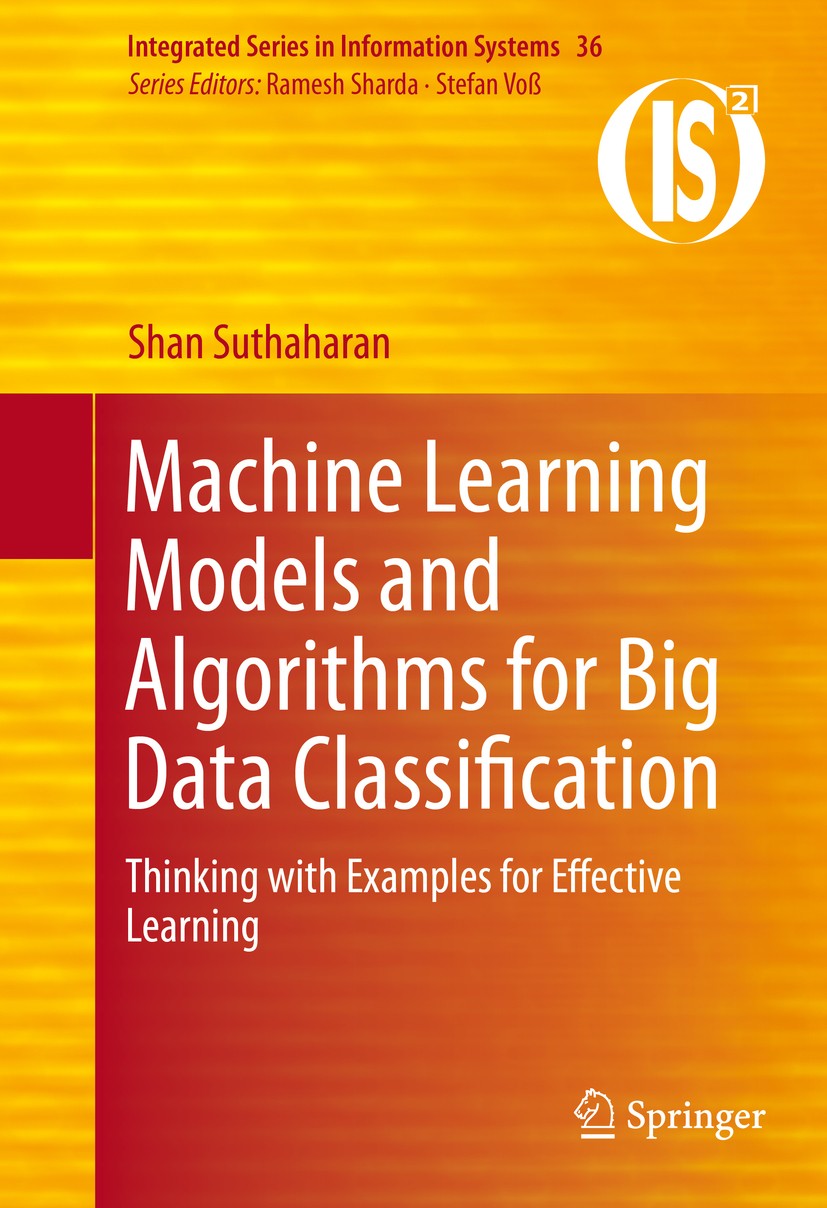 | 描述 | .This book presents machine learning models and algorithms to address big data classification problems. Existing machine learning techniques like the decision tree (a hierarchical approach), random forest (an ensemble hierarchical approach), and deep learning (a layered approach) are highly suitable for the system that can handle such problems. This book helps readers, especially students and newcomers to the field of big data and machine learning, to gain a quick understanding of the techniques and technologies; therefore, the theory, examples, and programs (Matlab and R) presented in this book have been simplified, hardcoded, repeated, or spaced for improvements. They provide vehicles to test and understand the complicated concepts of various topics in the field. It is expected that the readers adopt these programs to experiment with the examples, and then modify or write their own programs toward advancing their knowledge for solving more complex and challenging problems. .The presentation format of this book focuses on simplicity, readability, and dependability so that both undergraduate and graduate students as well as new researchers, developers, and practitioners in this fie | 出版日期 | Book 2016 | 关键词 | Big Data; Classification; Data Visualization; Machine Learning; Supervised Learning; Unit Circle Machine | 版次 | 1 | doi | https://doi.org/10.1007/978-1-4899-7641-3 | isbn_softcover | 978-1-4899-7852-3 | isbn_ebook | 978-1-4899-7641-3Series ISSN 1571-0270 Series E-ISSN 2197-7968 | issn_series | 1571-0270 | copyright | Springer Science+Business Media New York 2016 |
The information of publication is updating
|
|