书目名称 | Machine Learning Applications in Electronic Design Automation | 编辑 | Haoxing Ren,Jiang Hu | 视频video | http://file.papertrans.cn/621/620382/620382.mp4 | 概述 | Serves as a single-source reference to key machine learning (ML) applications and methods in digital.Covers classical ML methods, as well as deep learning models such as convolutional neural networks | 图书封面 | 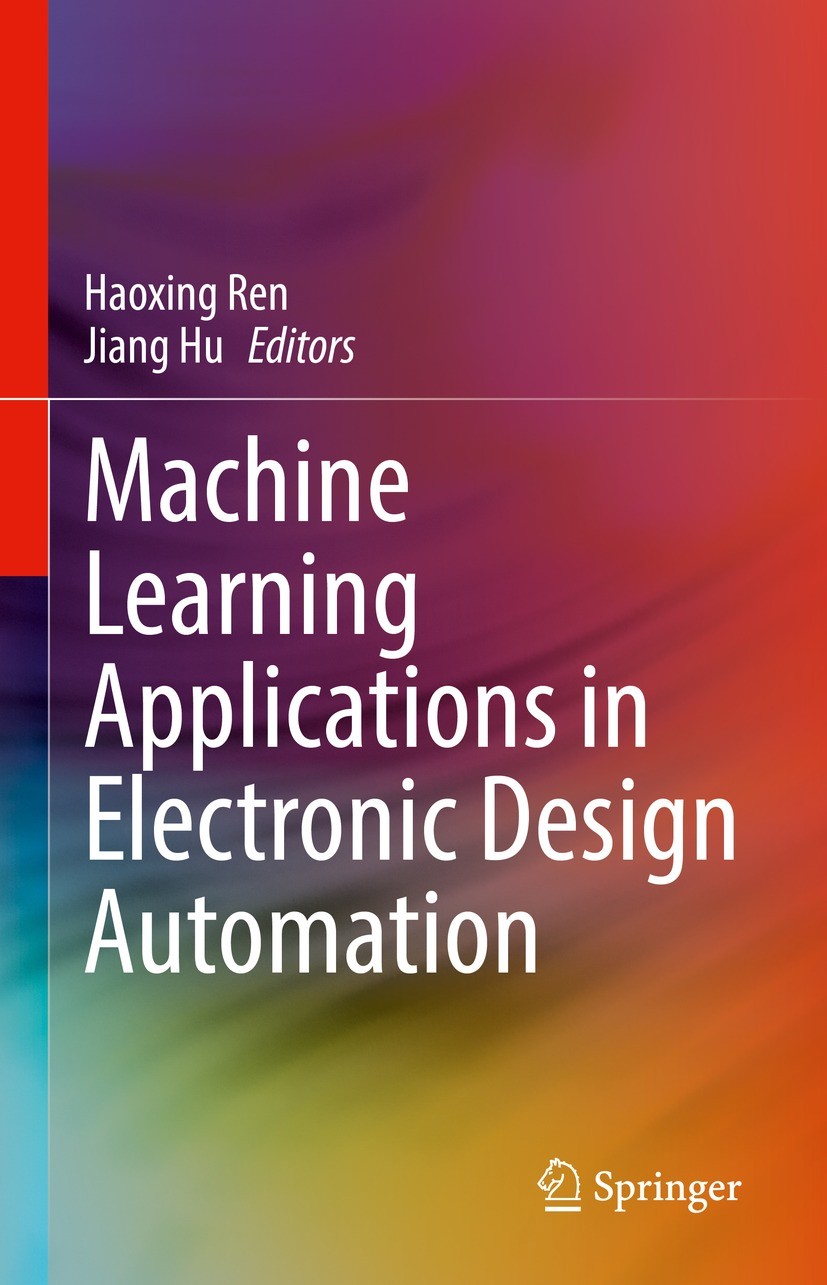 | 描述 | .This book serves as a single-source reference to key machine learning (ML) applications and methods in digital and analog design and verification. Experts from academia and industry cover a wide range of the latest research on ML applications in electronic design automation (EDA), including analysis and optimization of digital design, analysis and optimization of analog design, as well as functional verification, FPGA and system level designs, design for manufacturing (DFM), and design space exploration. The authors also cover key ML methods such as classical ML, deep learning models such as convolutional neural networks (CNNs), graph neural networks (GNNs), generative adversarial networks (GANs) and optimization methods such as reinforcement learning (RL) and Bayesian optimization (BO). All of these topics are valuable to chip designers and EDA developers and researchers working in digital and analog designs and verification. . | 出版日期 | Book 2022 | 关键词 | Deep learning for EDA; convolutional neural networks; graph neural networks; generative adversarial net | 版次 | 1 | doi | https://doi.org/10.1007/978-3-031-13074-8 | isbn_softcover | 978-3-031-13076-2 | isbn_ebook | 978-3-031-13074-8 | copyright | The Editor(s) (if applicable) and The Author(s), under exclusive license to Springer Nature Switzerl |
The information of publication is updating
|
|