书目名称 | Lifelong Machine Learning, Second Edition | 编辑 | Zhiyuan Chen,Bing Liu | 视频video | http://file.papertrans.cn/586/585945/585945.mp4 | 丛书名称 | Synthesis Lectures on Artificial Intelligence and Machine Learning | 图书封面 | 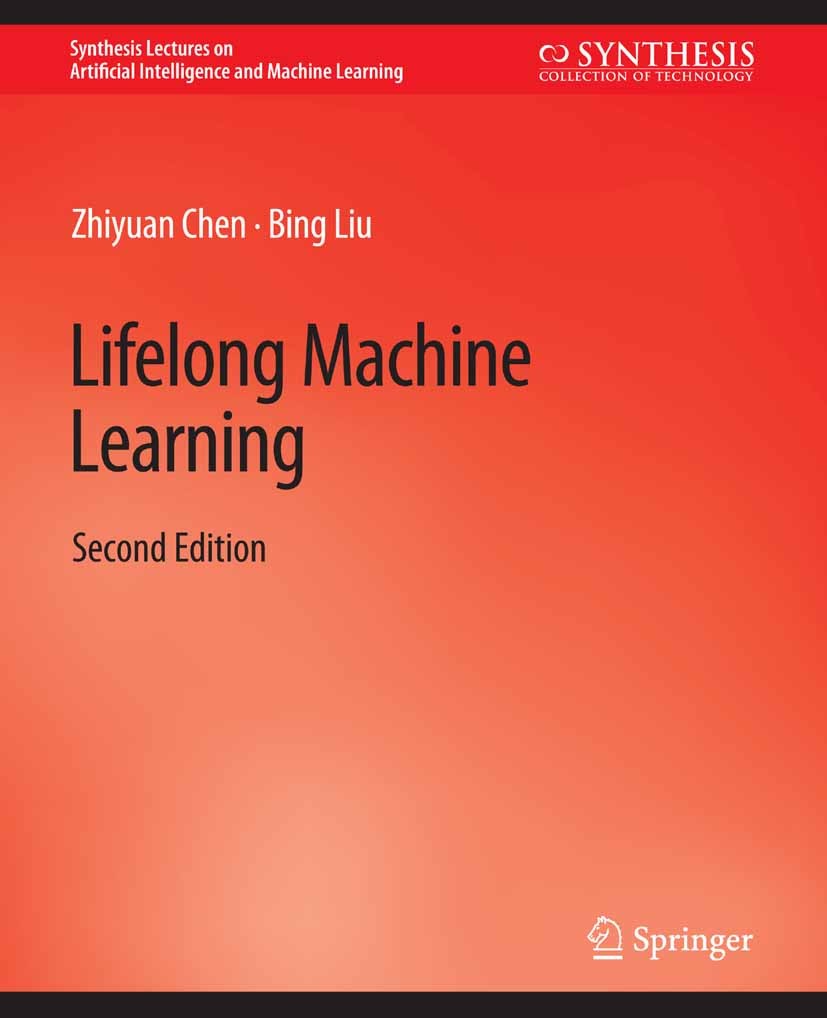 | 描述 | .Lifelong Machine Learning, Second Edition. is an introduction to an advanced machine learning paradigm that continuously learns by accumulating past knowledge that it then uses in future learning and problem solving. In contrast, the current dominant machine learning paradigm learns in isolation: given a training dataset, it runs a machine learning algorithm on the dataset to produce a model that is then used in its intended application. It makes no attempt to retain the learned knowledge and use it in subsequent learning. Unlike this isolated system, humans learn effectively with only a few examples precisely because our learning is very knowledge-driven: the knowledge learned in the past helps us learn new things with little data or effort. Lifelong learning aims to emulate this capability, because without it, an AI system cannot be considered truly intelligent...Research in lifelong learning has developed significantly in the relatively short time since the first edition of this book was published. The purpose of this second edition is to expand the definition of lifelong learning, update the content of several chapters, and add a new chapter about continual learning in deep ne | 出版日期 | Book 2018Latest edition | 版次 | 2 | doi | https://doi.org/10.1007/978-3-031-01581-6 | isbn_softcover | 978-3-031-00453-7 | isbn_ebook | 978-3-031-01581-6Series ISSN 1939-4608 Series E-ISSN 1939-4616 | issn_series | 1939-4608 | copyright | Springer Nature Switzerland AG 2018 |
The information of publication is updating
书目名称Lifelong Machine Learning, Second Edition影响因子(影响力) 
书目名称Lifelong Machine Learning, Second Edition影响因子(影响力)学科排名 
书目名称Lifelong Machine Learning, Second Edition网络公开度 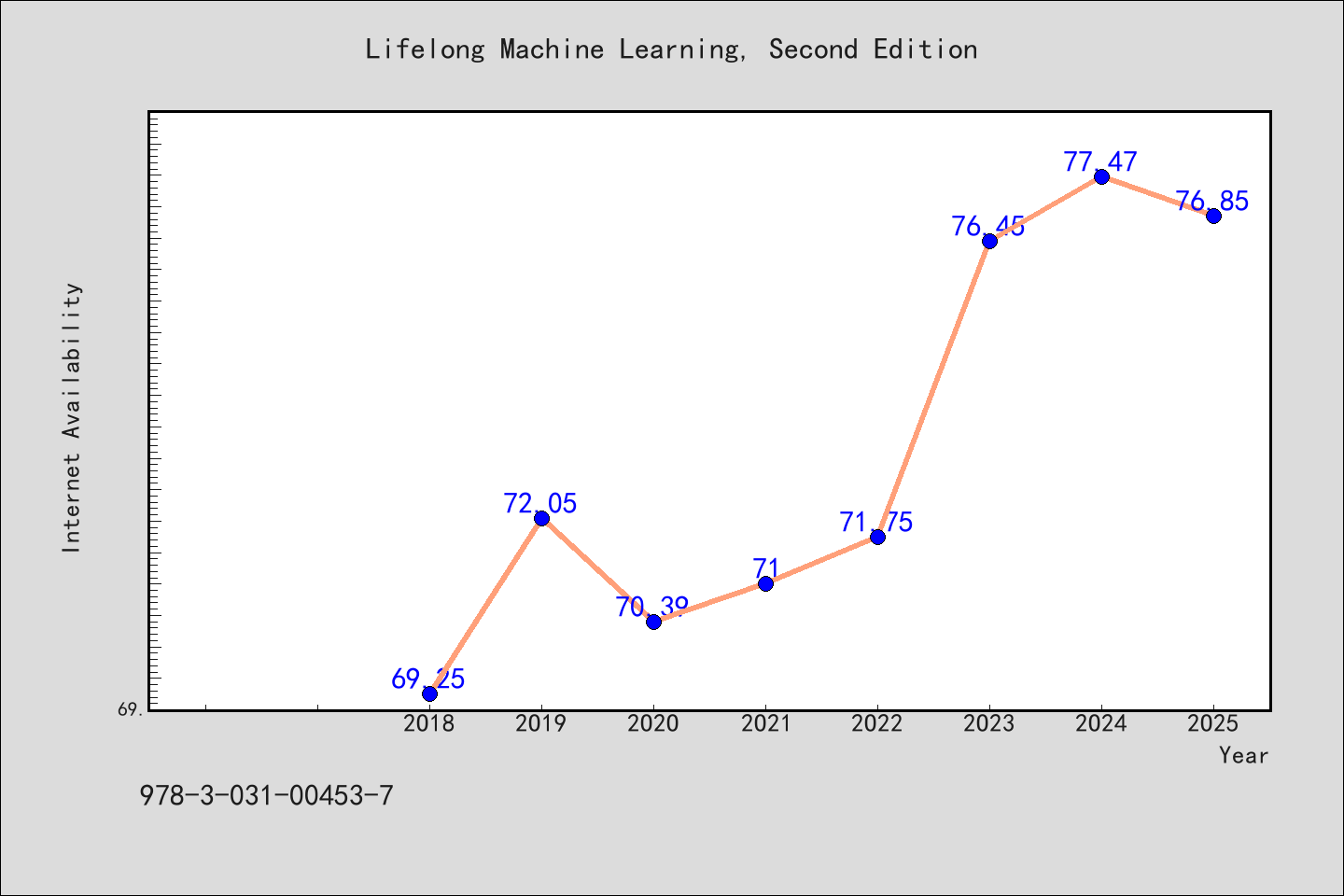
书目名称Lifelong Machine Learning, Second Edition网络公开度学科排名 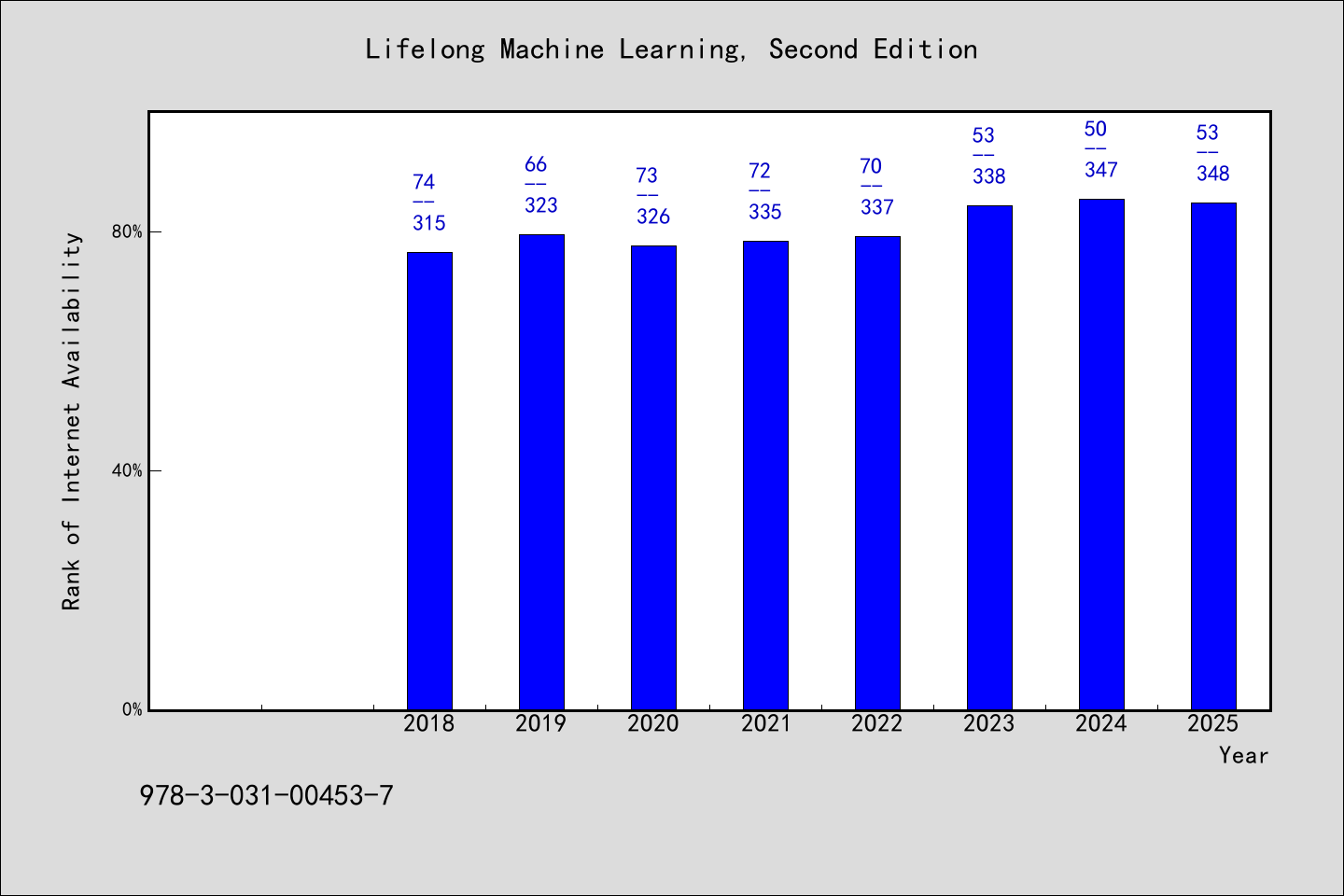
书目名称Lifelong Machine Learning, Second Edition被引频次 
书目名称Lifelong Machine Learning, Second Edition被引频次学科排名 
书目名称Lifelong Machine Learning, Second Edition年度引用 
书目名称Lifelong Machine Learning, Second Edition年度引用学科排名 
书目名称Lifelong Machine Learning, Second Edition读者反馈 
书目名称Lifelong Machine Learning, Second Edition读者反馈学科排名 
|
|
|