书目名称 | Learning with Partially Labeled and Interdependent Data |
编辑 | Massih-Reza Amini,Nicolas Usunier |
视频video | |
概述 | Presents an overview of statistical learning theory.Analyzes two machine learning frameworks, semi-supervised learning with partially labeled data and learning with interdependent data.Outlines how th |
图书封面 | 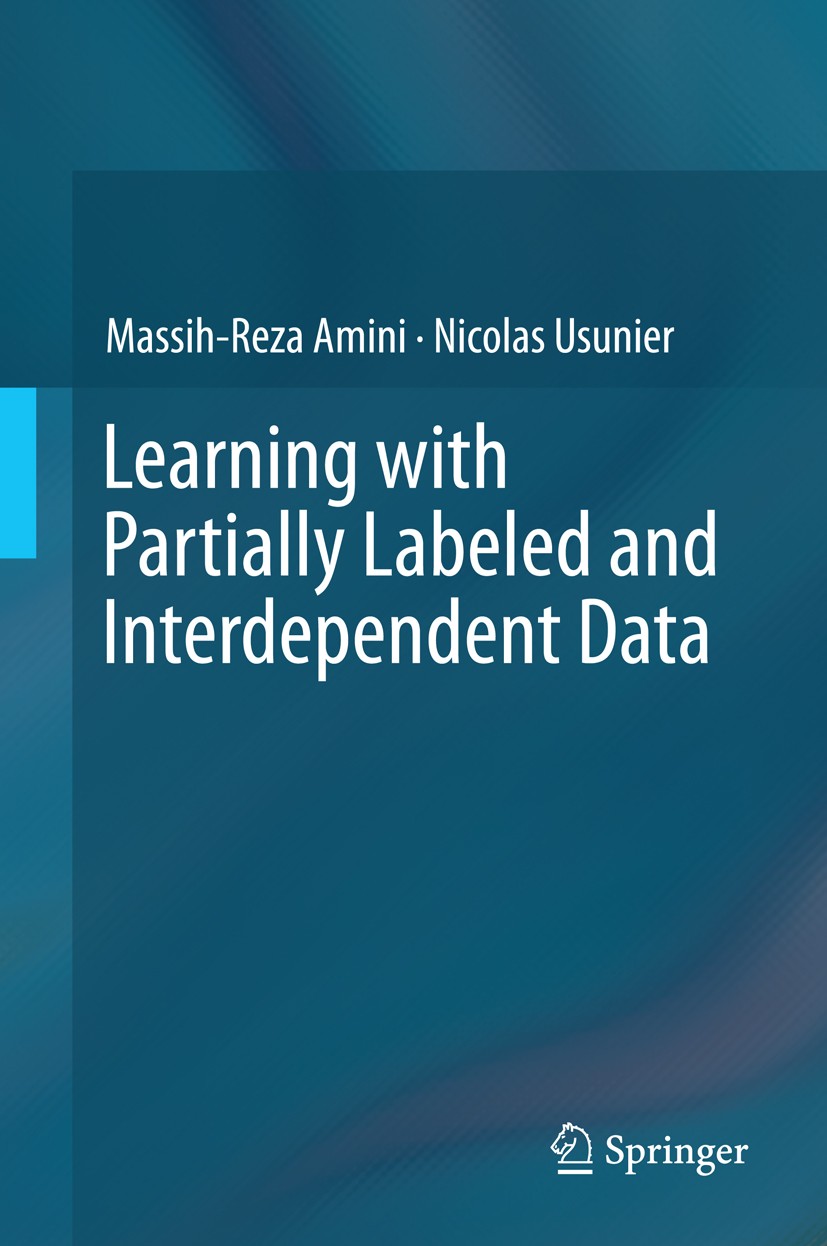 |
描述 | .This book develops two key machine learning principles: the semi-supervised paradigm and learning with interdependent data. It reveals new applications, primarily web related, that transgress the classical machine learning framework through learning with interdependent data. .The book traces how the semi-supervised paradigm and the learning to rank paradigm emerged from new web applications, leading to a massive production of heterogeneous textual data. It explains how semi-supervised learning techniques are widely used, but only allow a limited analysis of the information content and thus do not meet the demands of many web-related tasks..Later chapters deal with the development of learning methods for ranking entities in a large collection with respect to precise information needed. In some cases, learning a ranking function can be reduced to learning a classification function over the pairs of examples. The book proves that this task can be efficiently tackled in a new framework: learning with interdependent data..Researchers and professionals in machine learning will find these new perspectives and solutions valuable. Learning with Partially Labeled and Interdependent Data is |
出版日期 | Book 2015 |
关键词 | learning to rank; learning with interdependent data; learning with partially labeled data; machine lear |
版次 | 1 |
doi | https://doi.org/10.1007/978-3-319-15726-9 |
isbn_softcover | 978-3-319-35390-6 |
isbn_ebook | 978-3-319-15726-9 |
copyright | Springer International Publishing Switzerland 2015 |