书目名称 | Learning from Imbalanced Data Sets | 编辑 | Alberto Fernández,Salvador García,Francisco Herrer | 视频video | | 概述 | Offers a comprehensive review of imbalanced learning widely used worldwide in many real applications, such as fraud detection, disease diagnosis, etc.Provides the user with the required background and | 图书封面 | 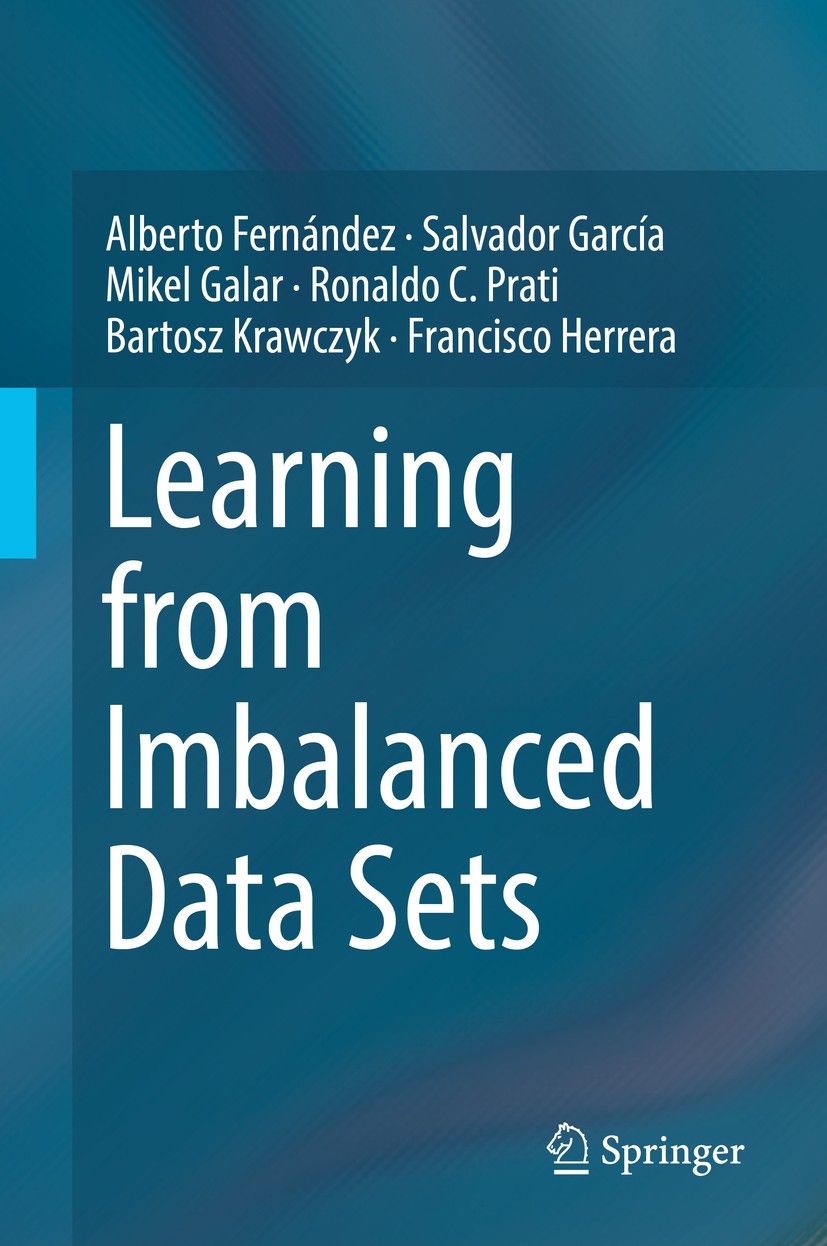 | 描述 | .This book provides a general and comprehensible overview of imbalanced learning. It contains a formal description of a problem, and focuses on its main features, and the most relevant proposed solutions. Additionally, it considers the different scenarios in Data Science for which the imbalanced classification can create a real challenge. .This book stresses the gap with standard classification tasks by reviewing the case studies and ad-hoc performance metrics that are applied in this area. It also covers the different approaches that have been traditionally applied to address the binary skewed class distribution. Specifically, it reviews cost-sensitive learning, data-level preprocessing methods and algorithm-level solutions, taking also into account those ensemble-learning solutions that embed any of the former alternatives. Furthermore, it focuses on the extension of the problem for multi-class problems, where the former classical methods are no longer to be applied in a straightforward way..This book also focuses on the data intrinsic characteristics that are the main causes which, added to the uneven class distribution, truly hinders the performance of classification algori | 出版日期 | Book 2018 | 关键词 | Machine learning; Data mining; Classification; Imbalanced data; Data preprocessing; Ensemble learning; Cos | 版次 | 1 | doi | https://doi.org/10.1007/978-3-319-98074-4 | isbn_softcover | 978-3-030-07446-3 | isbn_ebook | 978-3-319-98074-4 | copyright | Springer Nature Switzerland AG 2018 |
The information of publication is updating
|
|