书目名称 | Knowledge Representation and Organization in Machine Learning |
编辑 | Katharina Morik |
视频video | |
丛书名称 | Lecture Notes in Computer Science |
图书封面 | 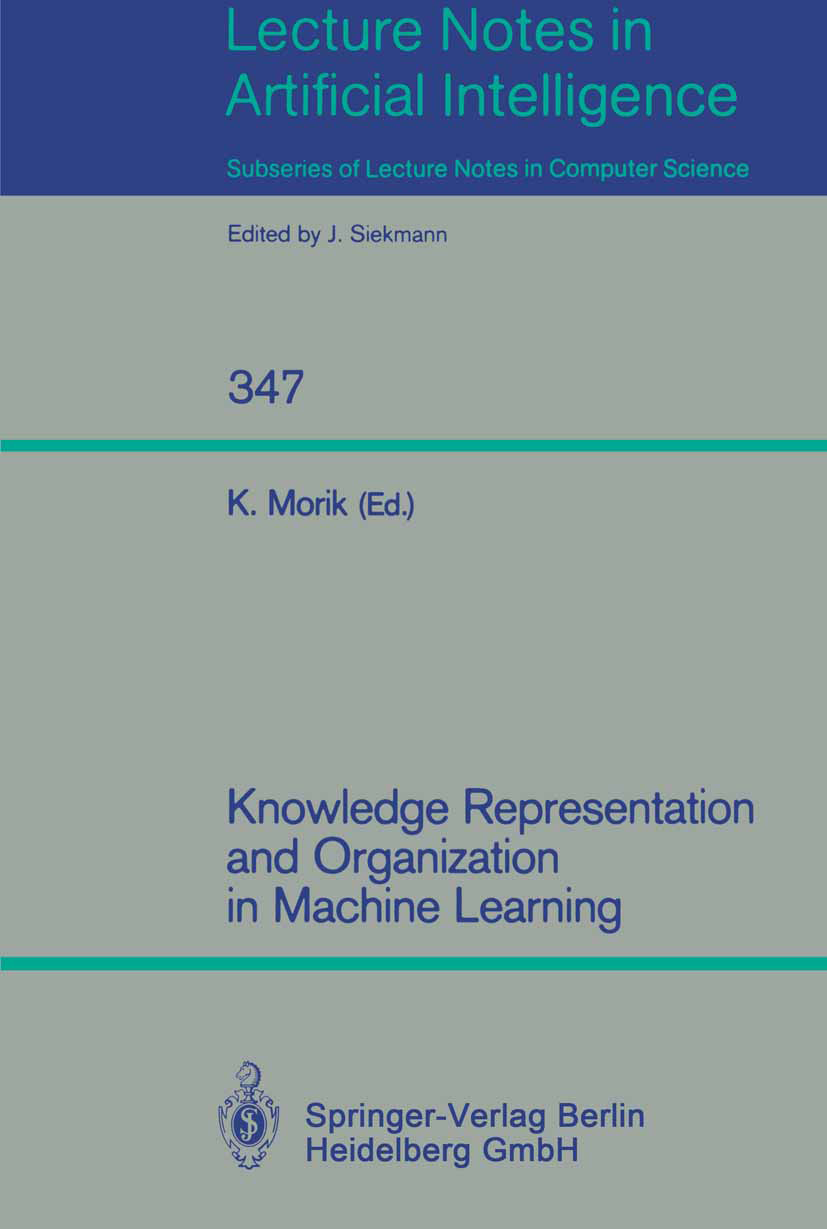 |
描述 | Machine learning has become a rapidly growing field of Artificial Intelligence. Since the First International Workshop on Machine Learning in 1980, the number of scientists working in the field has been increasing steadily. This situation allows for specialization within the field. There are two types of specialization: on subfields or, orthogonal to them, on special subjects of interest. This book follows the thematic orientation. It contains research papers, each of which throws light upon the relation between knowledge representation, knowledge acquisition and machine learning from a different angle. Building up appropriate representations is considered to be the main concern of knowledge acquisition for knowledge-based systems throughout the book. Here machine learning is presented as a tool for building up such representations. But machine learning itself also states new representational problems. This book gives an easy-to-understand insight into a new field with its problems and the solutions it offers. Thus it will be of good use to both experts and newcomers to the subject. |
出版日期 | Book 1989 |
关键词 | artificial intelligence; expert system; inference engine; intelligence; knowledge base; knowledge represe |
版次 | 1 |
doi | https://doi.org/10.1007/BFb0017213 |
isbn_softcover | 978-3-540-50768-0 |
isbn_ebook | 978-3-540-46081-7Series ISSN 0302-9743 Series E-ISSN 1611-3349 |
issn_series | 0302-9743 |
copyright | Springer-Verlag Berlin Heidelberg 1989 |