书目名称 | Information-Driven Machine Learning | 副标题 | Data Science as an E | 编辑 | Gerald Friedland | 视频video | | 概述 | Tackles the ‘why‘ questions of data science and deep learning.Interdisciplinary approach to model engineering.Information measurements for MLOps, Data drift, bias | 图书封面 | 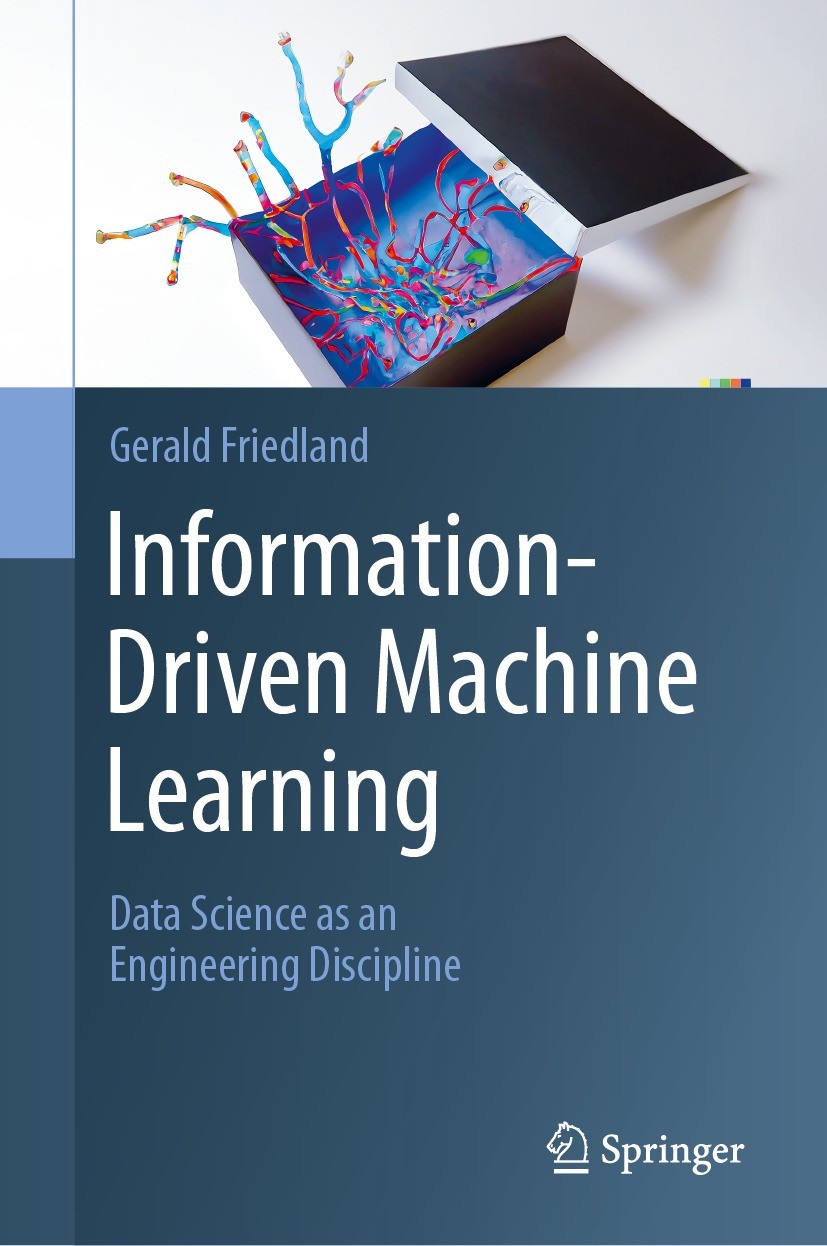 | 描述 | .This groundbreaking book transcends traditional machine learning approaches by introducing information measurement methodologies that revolutionize the field...Stemming from a UC Berkeley seminar on experimental design for machine learning tasks, these techniques aim to overcome the ‘black box‘ approach of machine learning by reducing conjectures such as magic numbers (hyper-parameters) or model-type bias. Information-based machine learning enables data quality measurements, a priori task complexity estimations, and reproducible design of data science experiments. The benefits include significant size reduction, increased explainability, and enhanced resilience of models, all contributing to advancing the discipline‘s robustness and credibility...While bridging the gap between machine learning and disciplines such as physics, information theory, and computer engineering, this textbook maintains an accessible and comprehensive style, making complex topics digestible fora broad readership. .Information-Driven Machine Learning. explores the synergistic harmony among these disciplines to enhance our understanding of data science modeling. Instead of solely focusing on the "how," this | 出版日期 | Textbook 2024 | 关键词 | machine learning experiments; information theory; information measurements; decision trees; neural netwo | 版次 | 1 | doi | https://doi.org/10.1007/978-3-031-39477-5 | isbn_softcover | 978-3-031-39479-9 | isbn_ebook | 978-3-031-39477-5 | copyright | The Editor(s) (if applicable) and The Author(s), under exclusive license to Springer Nature Switzerl |
The information of publication is updating
|
|