书目名称 | Independent Component Analysis and Signal Separation | 副标题 | 7th International Co | 编辑 | Mike E. Davies,Christopher J. James,Mark D Plumble | 视频video | | 丛书名称 | Lecture Notes in Computer Science | 图书封面 | 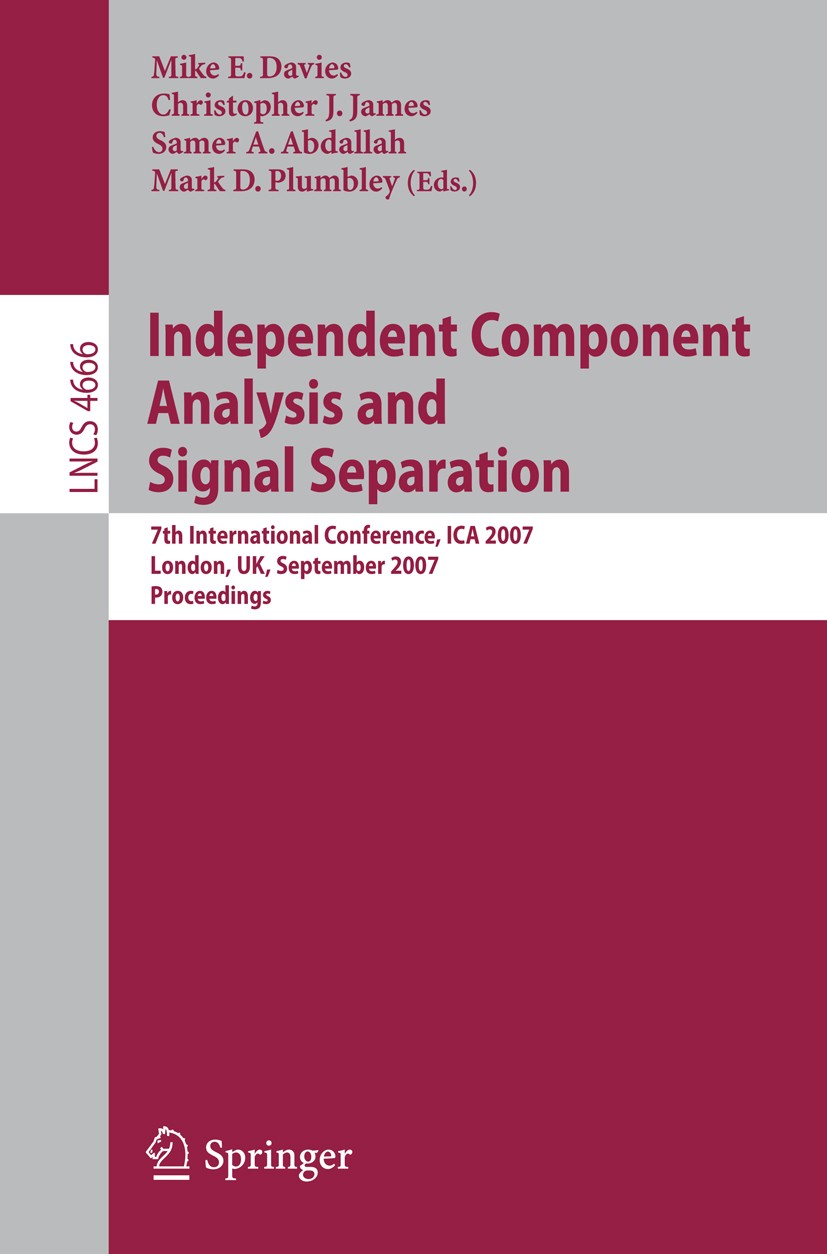 | 描述 | This volume contains the papers presented at the 7th International Conference on Independent Component Analysis (ICA) and Source Separation held in L- don, 9–12 September 2007, at Queen Mary, University of London. Independent Component Analysis and Signal Separation is one of the most exciting current areas of research in statistical signal processing and unsup- vised machine learning. The area has received attention from several research communities including machine learning, neural networks, statistical signal p- cessing and Bayesian modeling. Independent Component Analysis and Signal Separation has applications at the intersection of many science and engineering disciplinesconcernedwithunderstandingandextractingusefulinformationfrom data as diverse as neuronal activity and brain images, bioinformatics, com- nications, the World Wide Web, audio, video, sensor signals, or time series. This year’s event was organized by the EPSRC-funded UK ICA Research Network (www.icarn.org). There was also a minor change to the conference title this year with the exclusion of the word‘blind’. The motivation for this was the increasing number of interesting submissions using non-blind or semi-bli | 出版日期 | Conference proceedings 2007 | 关键词 | DOM; Estimator; Information; Minimum; Minimum Description Length; algorithms; audio segmentation; auditory | 版次 | 1 | doi | https://doi.org/10.1007/978-3-540-74494-8 | isbn_softcover | 978-3-540-74493-1 | isbn_ebook | 978-3-540-74494-8Series ISSN 0302-9743 Series E-ISSN 1611-3349 | issn_series | 0302-9743 | copyright | Springer-Verlag Berlin Heidelberg 2007 |
The information of publication is updating
|
|