书目名称 | Federated Learning |
副标题 | Privacy and Incentiv |
编辑 | Qiang Yang,Lixin Fan,Han Yu |
视频video | |
概述 | Provides a comprehensive and self-contained introduction to Federated Learning.Popular topic for GDPR.Covers learning, implementation and practice of Federated Learning |
丛书名称 | Lecture Notes in Computer Science |
图书封面 | 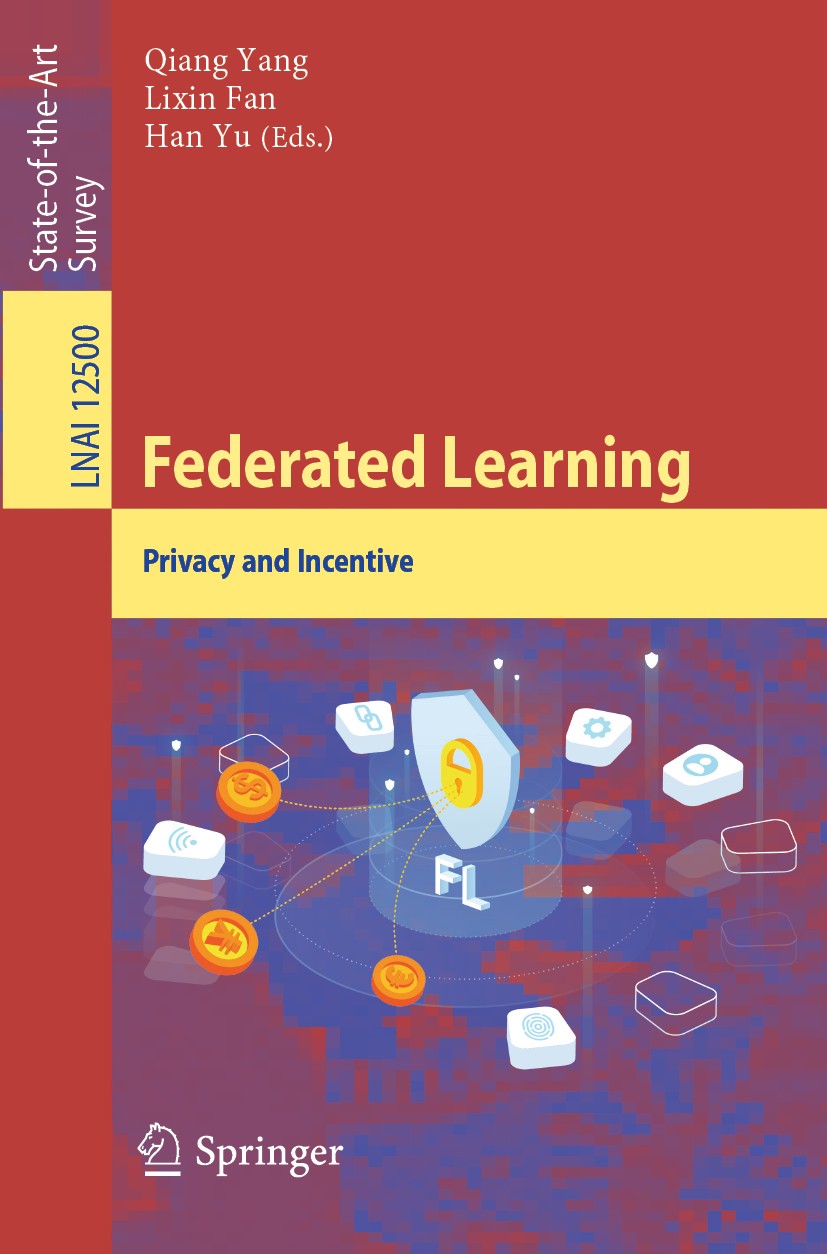 |
描述 | .This book provides a comprehensive and self-contained introduction to federated learning, ranging from the basic knowledge and theories to various key applications. ..Privacy and incentive issues are the focus of this book. It is timely as federated learning is becoming popular after the release of the General Data Protection Regulation (GDPR). Since federated learning aims to enable a machine model to be collaboratively trained without each party exposing private data to others. This setting adheres to regulatory requirements of data privacy protection such as GDPR...This book contains three main parts. Firstly, it introduces different privacy-preserving methods for protecting a federated learning model against different types of attacks such as data leakage and/or data poisoning. Secondly, the book presents incentive mechanisms which aim to encourage individuals to participate in the federated learning ecosystems. Last but not least, this book also describes how federated learning can be applied in industry and business to address data silo and privacy-preserving problems. The book is intended for readers from both the academia and the industry, who would like to learn about fed |
出版日期 | Book 2020 |
关键词 | distributed machine learning; privacy preserving; machine learning; adversarial learning; artificial int |
版次 | 1 |
doi | https://doi.org/10.1007/978-3-030-63076-8 |
isbn_softcover | 978-3-030-63075-1 |
isbn_ebook | 978-3-030-63076-8Series ISSN 0302-9743 Series E-ISSN 1611-3349 |
issn_series | 0302-9743 |
copyright | Springer Nature Switzerland AG 2020 |