书目名称 | Elliptically Symmetric Distributions in Signal Processing and Machine Learning | 编辑 | Jean-Pierre Delmas,Mohammed Nabil El Korso,Frédéri | 视频video | | 概述 | Avoids the need to make assumptions about Gaussian distributions in data.Provides a general, flexible method of signal processing analysis.Is helpful in a variety of practical applications | 图书封面 | 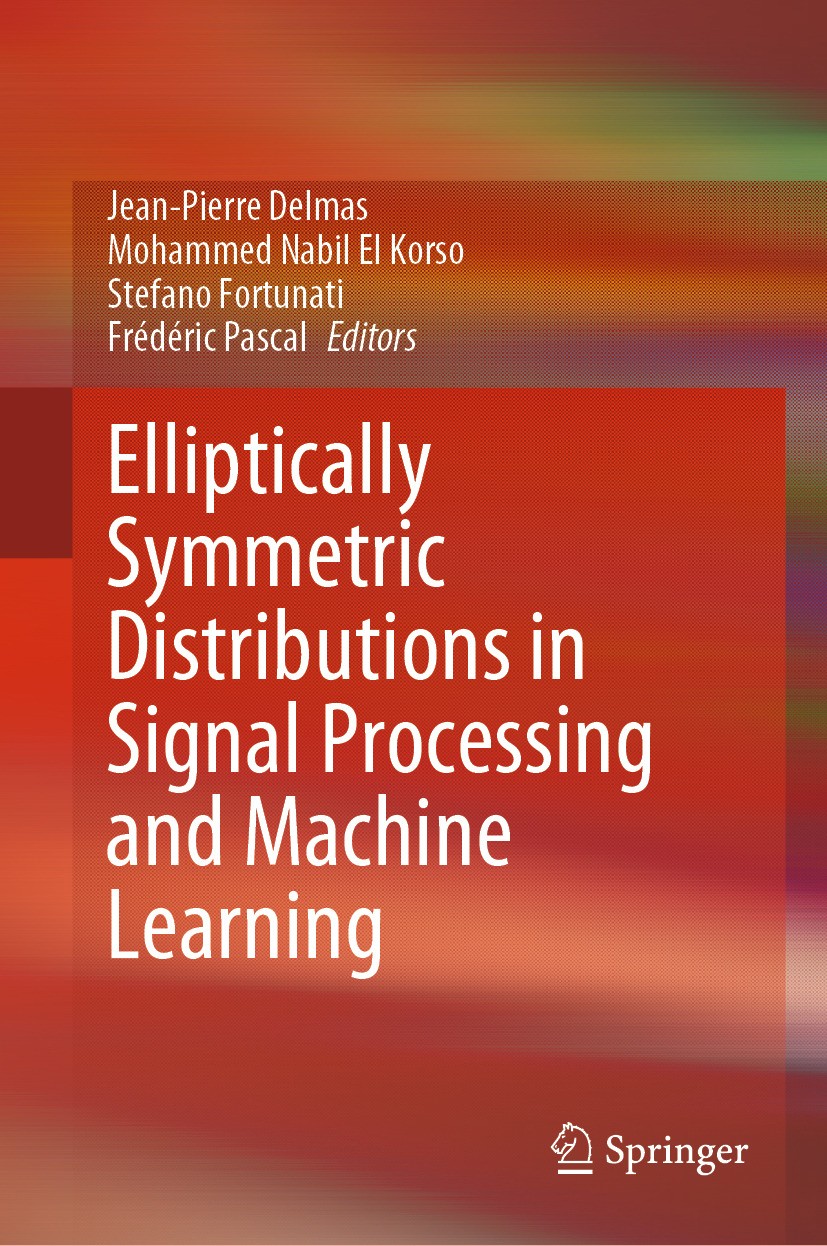 | 描述 | .This book constitutes a review of recent developments in the theory and practical exploitation of the elliptical model for measured data in both classical and emerging areas of signal processing. It develops techniques usable in (among other areas): graph learning, robust clustering, linear shrinkage, information geometry, subspace-based algorithm design, and semiparametric and misspecified estimation.. .The various contributions combine to show how the goal of inferring information from a set of acquired data, recurrent in statistical signal processing, can be achieved, even when the common practical assumption of Gaussian distribution in the data is not valid. The elliptical model propounded maintains the performance of its inference procedures even when that assumption fails. The elliptical distribution, being fully characterized by its location vector, its scatter/covariance matrix and its so-called density generator, used to describe the impulsiveness of the data, is sufficiently flexible to model heterogeneous applications.. . .This book is of interest to any graduate students and academic researchers wishing to acquaint themselves with the latest research in an area of risi | 出版日期 | Book 2024 | 关键词 | Graph Learning; Linear Shrinkage; Missing Data; Robust Statistics; Elliptical Distribution; Information G | 版次 | 1 | doi | https://doi.org/10.1007/978-3-031-52116-4 | isbn_softcover | 978-3-031-52118-8 | isbn_ebook | 978-3-031-52116-4 | copyright | The Editor(s) (if applicable) and The Author(s), under exclusive license to Springer Nature Switzerl |
The information of publication is updating
|
|