书目名称 | Extreme Value Theory-Based Methods for Visual Recognition | 编辑 | Walter J. Scheirer | 视频video | | 丛书名称 | Synthesis Lectures on Computer Vision | 图书封面 | 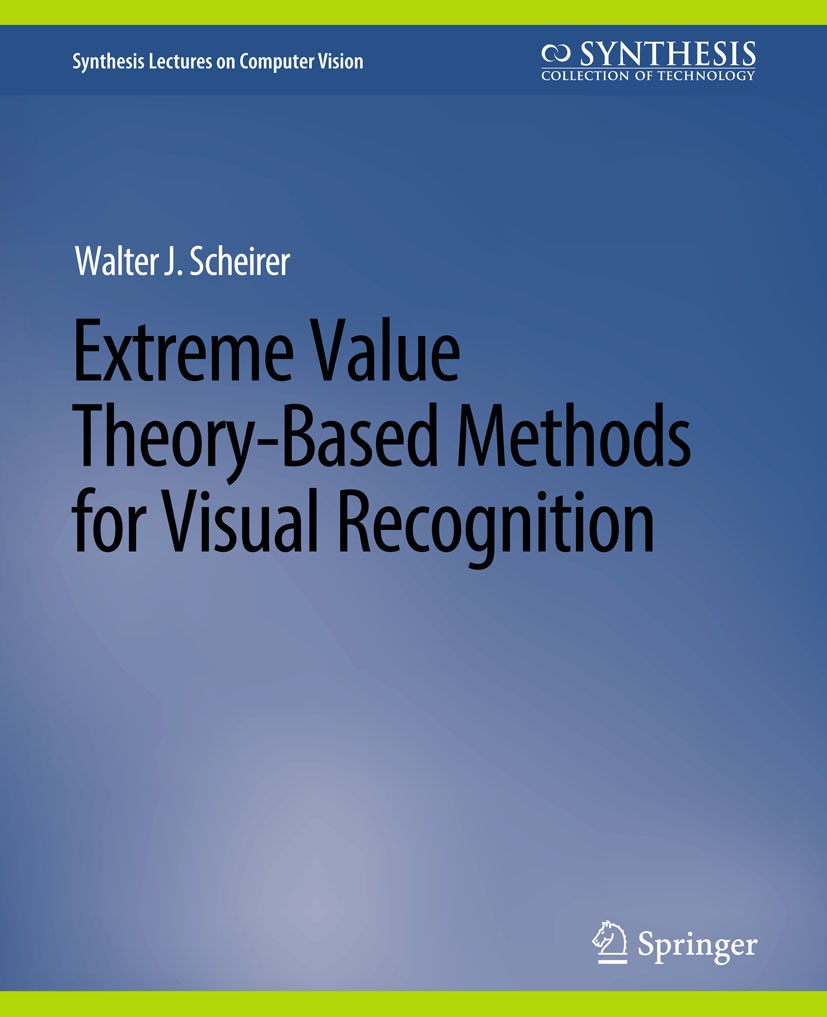 | 描述 | A common feature of many approaches to modeling sensory statistics is an emphasis on capturing the "average." From early representations in the brain, to highly abstracted class categories in machine learning for classification tasks, central-tendency models based on the Gaussian distribution are a seemingly natural and obvious choice for modeling sensory data. However, insights from neuroscience, psychology, and computer vision suggest an alternate strategy: preferentially focusing representational resources on the extremes of the distribution of sensory inputs. The notion of treating extrema near a decision boundary as features is not necessarily new, but a comprehensive statistical theory of recognition based on extrema is only now just emerging in the computer vision literature. This book begins by introducing the statistical Extreme Value Theory (EVT) for visual recognition. In contrast to central-tendency modeling, it is hypothesized that distributions near decision boundaries form a more powerful model for recognition tasks by focusing coding resources on data that are arguably the most diagnostic features. EVT has several important properties: strong statistical grounding, | 出版日期 | Book 2017 | 版次 | 1 | doi | https://doi.org/10.1007/978-3-031-01817-6 | isbn_softcover | 978-3-031-00689-0 | isbn_ebook | 978-3-031-01817-6Series ISSN 2153-1056 Series E-ISSN 2153-1064 | issn_series | 2153-1056 | copyright | Springer Nature Switzerland AG 2017 |
The information of publication is updating
|
|