书目名称 | Embedded Deep Learning | 副标题 | Algorithms, Architec | 编辑 | Bert Moons,Daniel Bankman,Marian Verhelst | 视频video | | 概述 | Gives a wide overview of a series of effective solutions for energy-efficient neural networks on battery constrained wearable devices.Discusses the optimization of neural networks for embedded deploym | 图书封面 | 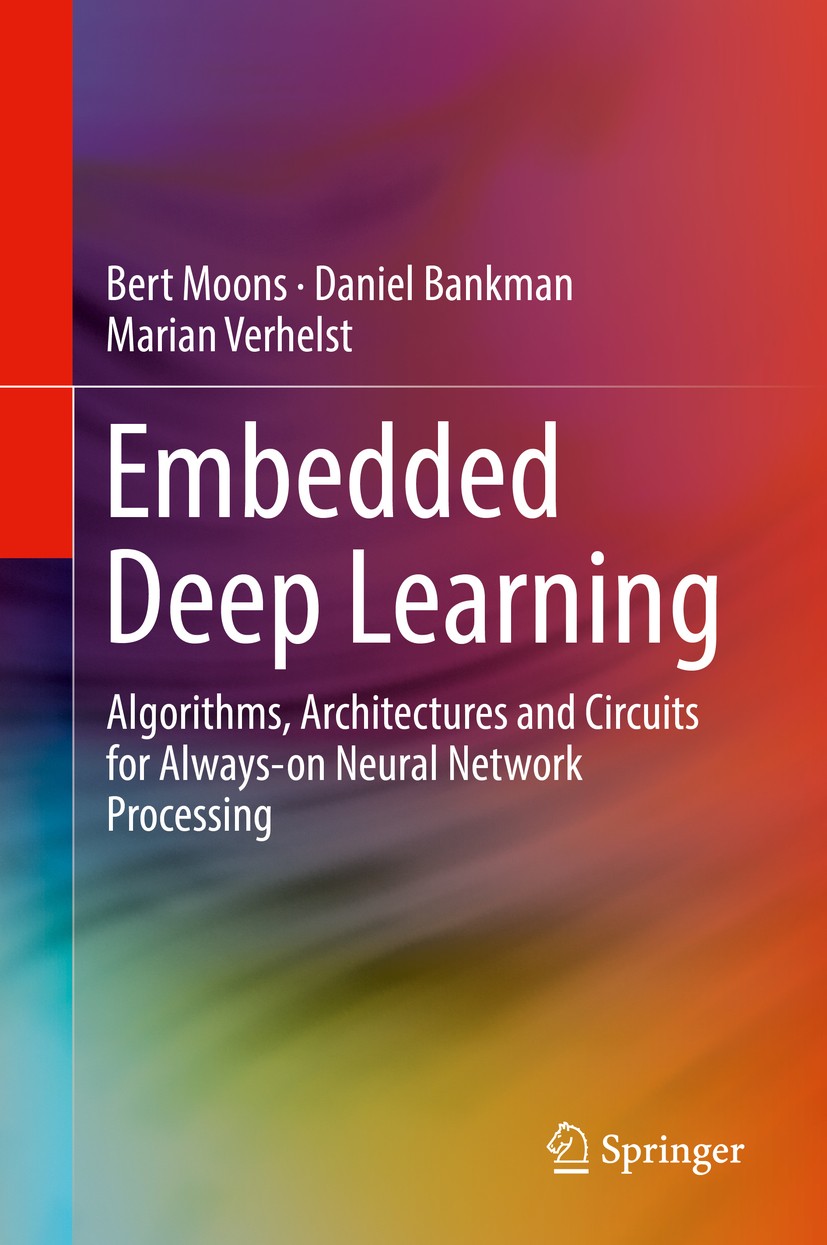 | 描述 | .This book covers algorithmic and hardware implementation techniques to enable embedded deep learning. The authors describe synergetic design approaches on the application-, algorithmic-, computer architecture-, and circuit-level that will help in achieving the goal of reducing the computational cost of deep learning algorithms. The impact of these techniques is displayed in four silicon prototypes for embedded deep learning..Gives a wide overview of a series of effective solutions for energy-efficient neural networks on battery constrained wearable devices;.Discusses the optimization of neural networks for embedded deployment on all levels of the design hierarchy – applications, algorithms, hardware architectures, and circuits – supported by real silicon prototypes;.Elaborates on how to design efficient Convolutional Neural Network processors, exploiting parallelism and data-reuse, sparse operations, and low-precision computations;.Supports the introduced theory and design concepts by four real silicon prototypes. The physical realization’s implementation and achieved performances are discussed elaborately to illustrated and highlight the introduced cross-layer design concepts.. | 出版日期 | Book 2019 | 关键词 | Deep Learning for Computer Architects; Embedded Deep Neural Networks; optimization of a neural network | 版次 | 1 | doi | https://doi.org/10.1007/978-3-319-99223-5 | isbn_softcover | 978-3-030-07577-4 | isbn_ebook | 978-3-319-99223-5 | copyright | Springer Nature Switzerland AG 2019 |
The information of publication is updating
|
|