书目名称 | Dependent Data in Social Sciences Research | 副标题 | Forms, Issues, and M | 编辑 | Mark Stemmler,Wolfgang Wiedermann,Francis L. Huang | 视频video | | 概述 | Presents new developments and applications for dependent data.Includs methods for the analysis of longitudinal data and corrections for degrees of freedom.Covers growth curve modeling, directional dep | 图书封面 | 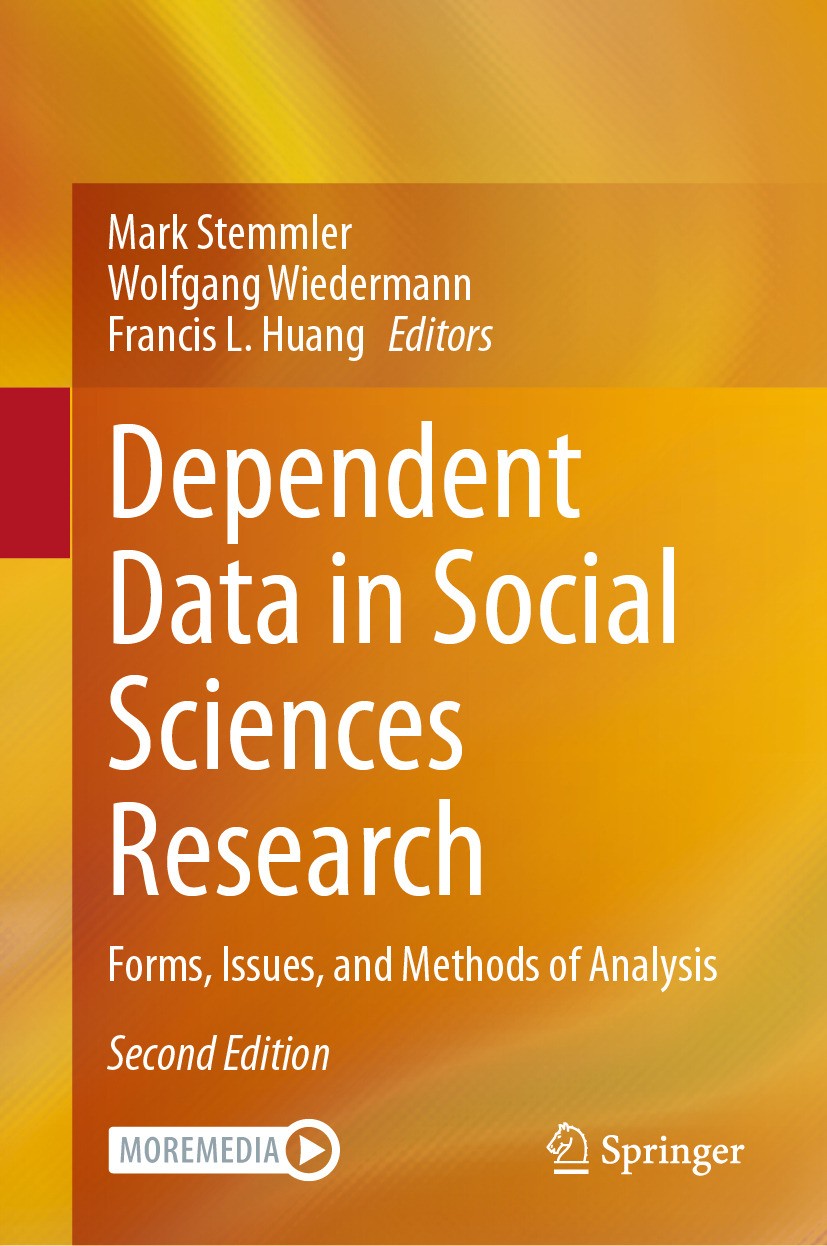 | 描述 | .This book covers the following subjects: growth curve modeling, directional dependence, dyadic data modeling, item response modeling (IRT), and other methods for the analysis of dependent data (e.g., approaches for modeling cross-section dependence, multidimensional scaling techniques, and mixed models). It presents contributions on handling data in which the postulate of independence in the data matrix is violated. When this postulate is violated and when the methods assuming independence are still applied, the estimated parameters are likely to be biased, and statistical decisions are very likely to be incorrect. Problems associated with dependence in data have been known for a long time, and led to the development of tailored methods for the analysis of dependent data in various areas of statistical analysis. These include, for example, methods for the analysis of longitudinal data, corrections for dependency, and corrections for degrees of freedom. .Researchers and graduate students in the social and behavioral sciences, education, econometrics, and medicine will find this up-to-date overview of modern statistical approaches for dealing with problems related to dependent data | 出版日期 | Book 2024Latest edition | 关键词 | analysis of longitudinal panel count data; close proximity data; clustered or paired data; corrections | 版次 | 2 | doi | https://doi.org/10.1007/978-3-031-56318-8 | isbn_softcover | 978-3-031-56320-1 | isbn_ebook | 978-3-031-56318-8 | copyright | The Editor(s) (if applicable) and The Author(s), under exclusive license to Springer Nature Switzerl |
The information of publication is updating
|
|