书目名称 | Deep Learning Theory and Applications |
副标题 | 5th International Co |
编辑 | Ana Fred,Allel Hadjali,Carlo Sansone |
视频video | |
丛书名称 | Communications in Computer and Information Science |
图书封面 | 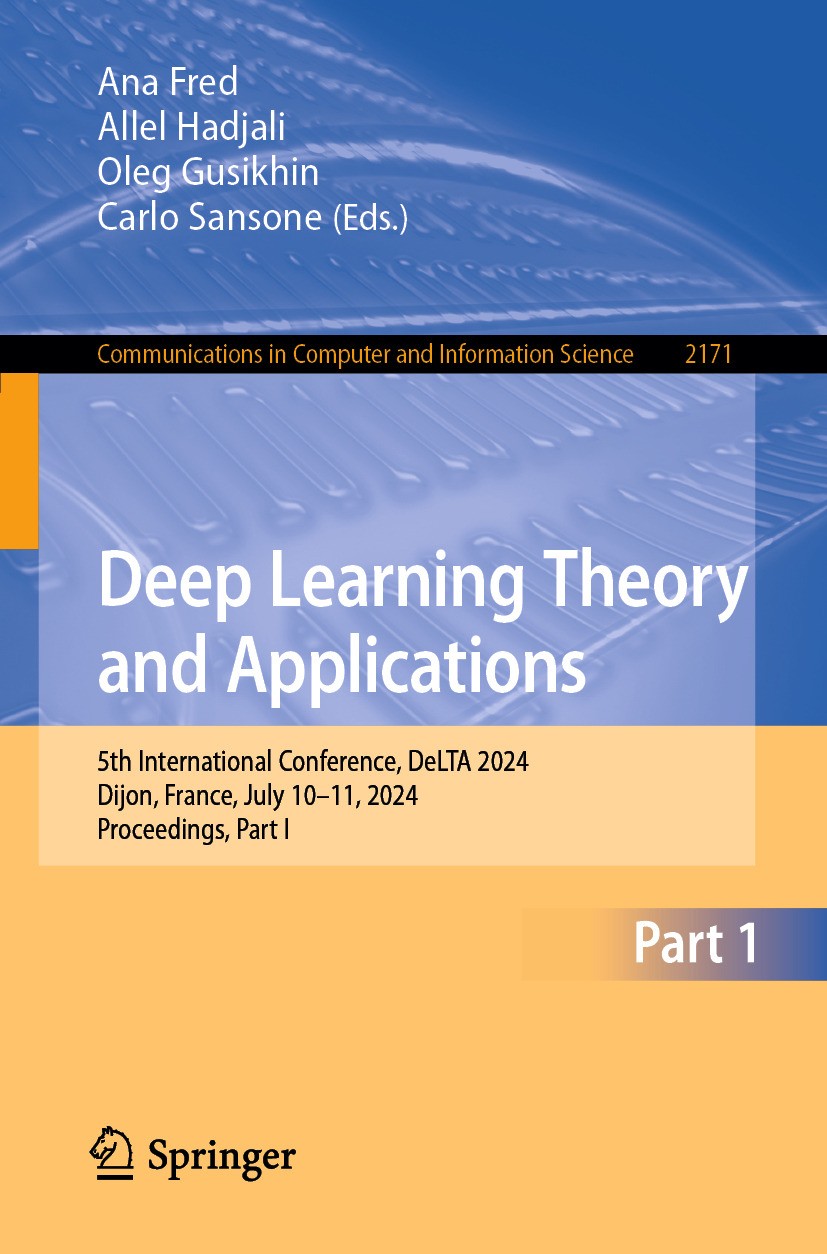 |
描述 | .The two-volume set CCIS 2171 and 2172 constitutes the refereed best papers from the 5th International Conference on Deep Learning Theory and Applications, DeLTA 2024, which took place in Dijon, France, during July 10-11, 2024. ..The 44 papers included in these proceedings were carefully reviewed and selected from a total of 70 submissions. They focus on topics such as deep learning and big data analytics; machine-learning and artificial intelligence, etc. . |
出版日期 | Conference proceedings 2024 |
关键词 | Models and Algorithms; machine learning; Big Data Analytics; Computer Vision Applications; Natural Langu |
版次 | 1 |
doi | https://doi.org/10.1007/978-3-031-66694-0 |
isbn_softcover | 978-3-031-66693-3 |
isbn_ebook | 978-3-031-66694-0Series ISSN 1865-0929 Series E-ISSN 1865-0937 |
issn_series | 1865-0929 |
copyright | The Editor(s) (if applicable) and The Author(s), under exclusive license to Springer Nature Switzerl |