书目名称 | Distributed, Collaborative, and Federated Learning, and Affordable AI and Healthcare for Resource Di | 副标题 | Third MICCAI Worksho | 编辑 | Shadi Albarqouni,Spyridon Bakas,Daguang Xu | 视频video | | 丛书名称 | Lecture Notes in Computer Science | 图书封面 | 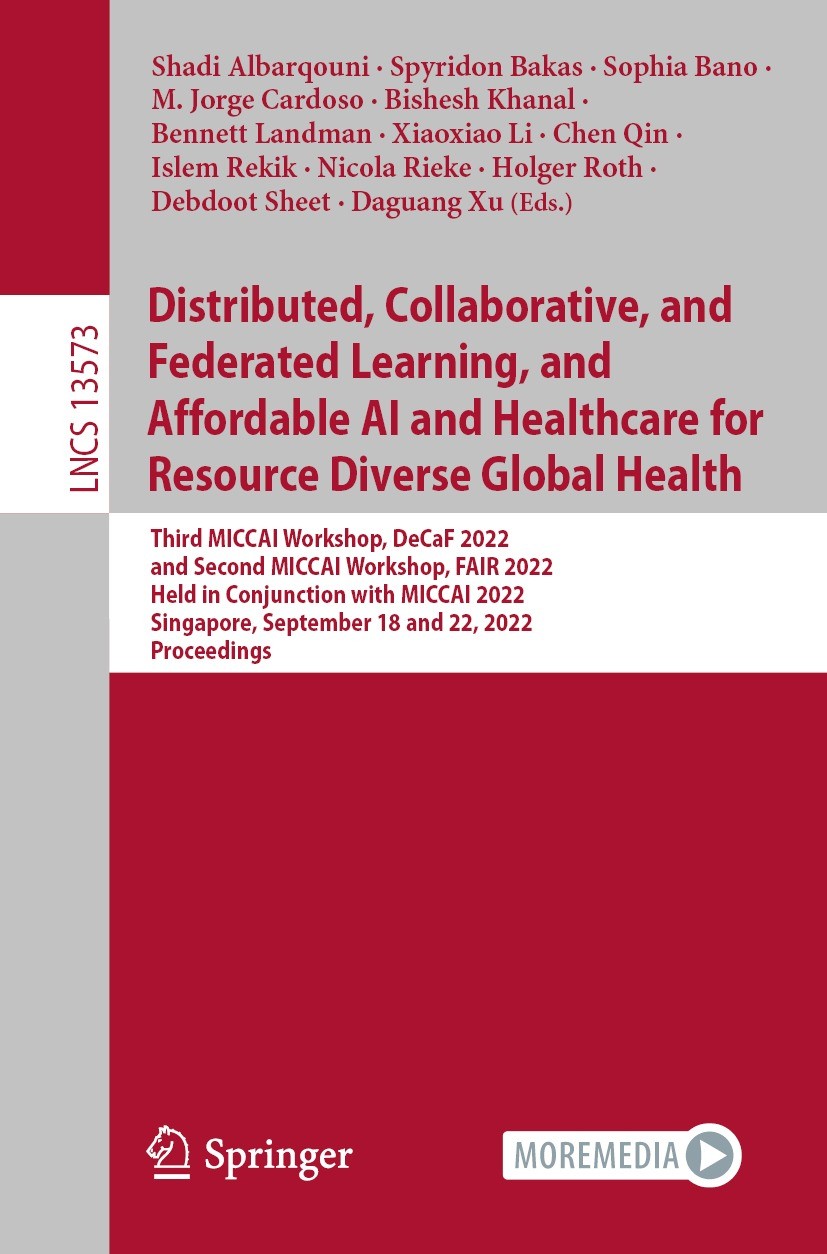 | 描述 | This book constitutes the refereed proceedings of the Third MICCAI Workshop on Distributed, Collaborative, and Federated Learning, DeCaF 2022, and the Second MICCAI Workshop on Affordable AI and Healthcare, FAIR 2022, held in conjunction with MICCAI 2022, in Singapore in September 2022. FAIR 2022 was held as a hybrid event..DeCaF 2022 accepted 14 papers from the 18 submissions received. The workshop aims at creating a scientific discussion focusing on the comparison, evaluation, and discussion of methodological advancement and practical ideas about machine learning applied to problems where data cannot be stored in centralized databases or where information privacy is a priority...For FAIR 2022, 4 papers from 9 submissions were accepted for publication. The topics of the accepted submissions focus on deep ultrasound segmentation, portable OCT image quality enhancement, self-attention deep networks and knowledge distillation in low-regime setting.. | 出版日期 | Conference proceedings 2022 | 关键词 | artificial intelligence; bioinformatics; computer networks; computer vision; cryptography; data mining; da | 版次 | 1 | doi | https://doi.org/10.1007/978-3-031-18523-6 | isbn_softcover | 978-3-031-18522-9 | isbn_ebook | 978-3-031-18523-6Series ISSN 0302-9743 Series E-ISSN 1611-3349 | issn_series | 0302-9743 | copyright | The Editor(s) (if applicable) and The Author(s), under exclusive license to Springer Nature Switzerl |
The information of publication is updating
书目名称Distributed, Collaborative, and Federated Learning, and Affordable AI and Healthcare for Resource Di影响因子(影响力) 
书目名称Distributed, Collaborative, and Federated Learning, and Affordable AI and Healthcare for Resource Di影响因子(影响力)学科排名 
书目名称Distributed, Collaborative, and Federated Learning, and Affordable AI and Healthcare for Resource Di网络公开度 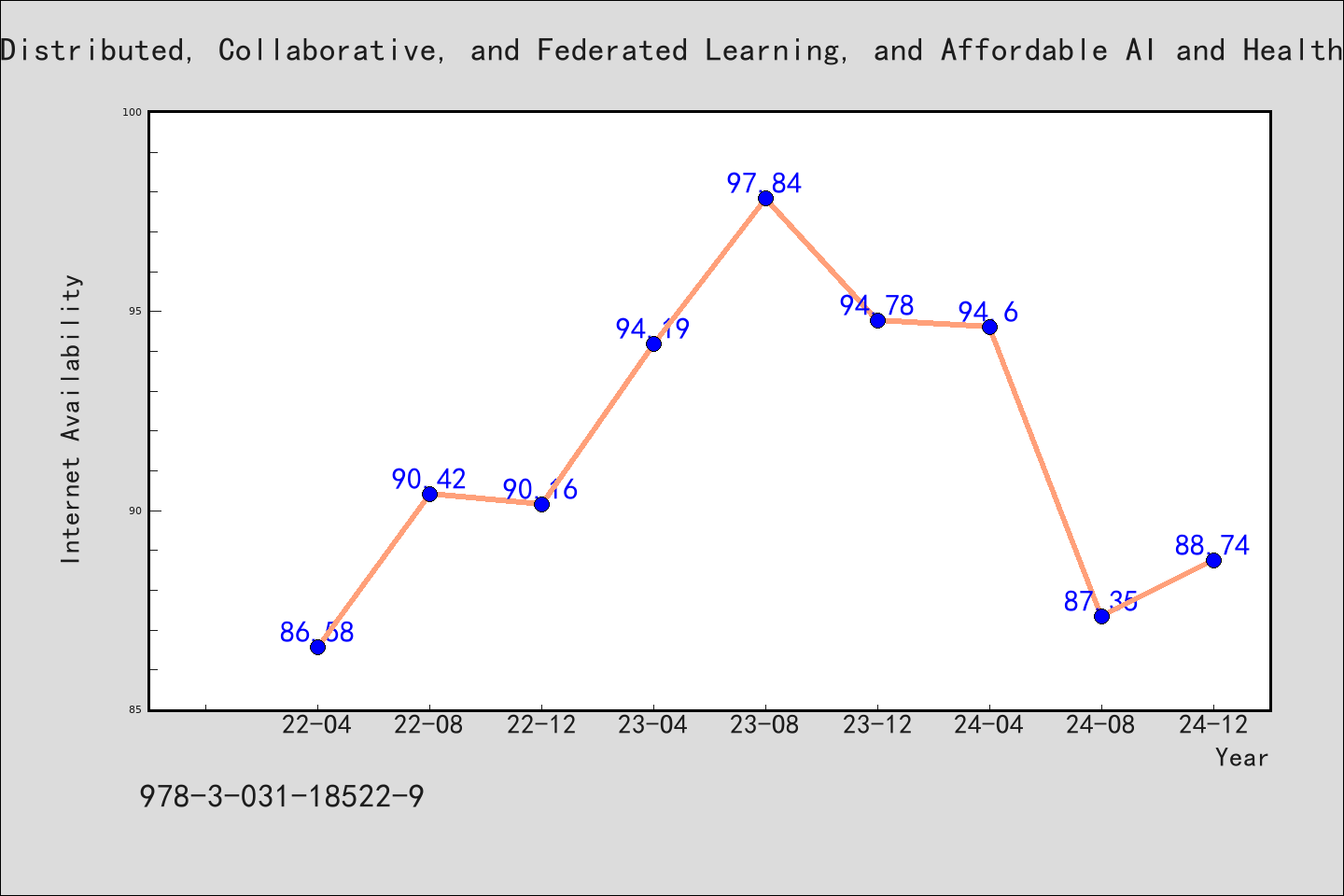
书目名称Distributed, Collaborative, and Federated Learning, and Affordable AI and Healthcare for Resource Di网络公开度学科排名 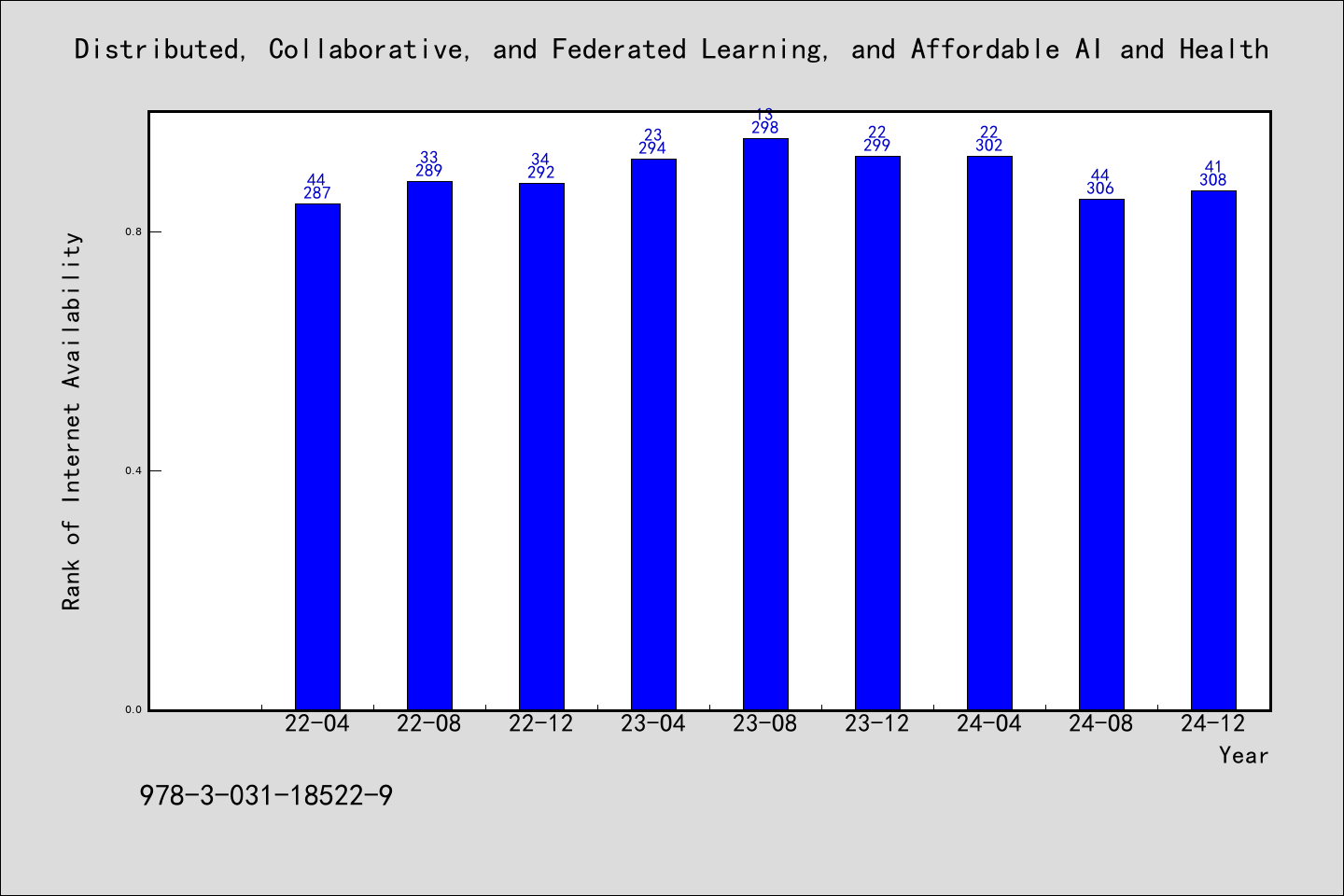
书目名称Distributed, Collaborative, and Federated Learning, and Affordable AI and Healthcare for Resource Di被引频次 
书目名称Distributed, Collaborative, and Federated Learning, and Affordable AI and Healthcare for Resource Di被引频次学科排名 
书目名称Distributed, Collaborative, and Federated Learning, and Affordable AI and Healthcare for Resource Di年度引用 
书目名称Distributed, Collaborative, and Federated Learning, and Affordable AI and Healthcare for Resource Di年度引用学科排名 
书目名称Distributed, Collaborative, and Federated Learning, and Affordable AI and Healthcare for Resource Di读者反馈 
书目名称Distributed, Collaborative, and Federated Learning, and Affordable AI and Healthcare for Resource Di读者反馈学科排名 
|
|
|