书目名称 | Distributed Machine Learning with PySpark | 副标题 | Migrating Effortless | 编辑 | Abdelaziz Testas | 视频video | | 概述 | Covers migrating from Pandas, Scikit-Learn to PySpark, from single-node to large-scale computing.Explains deploying ML models to production with Scikit-Learn and PySpark.Explains how to use PySpark fo | 图书封面 | 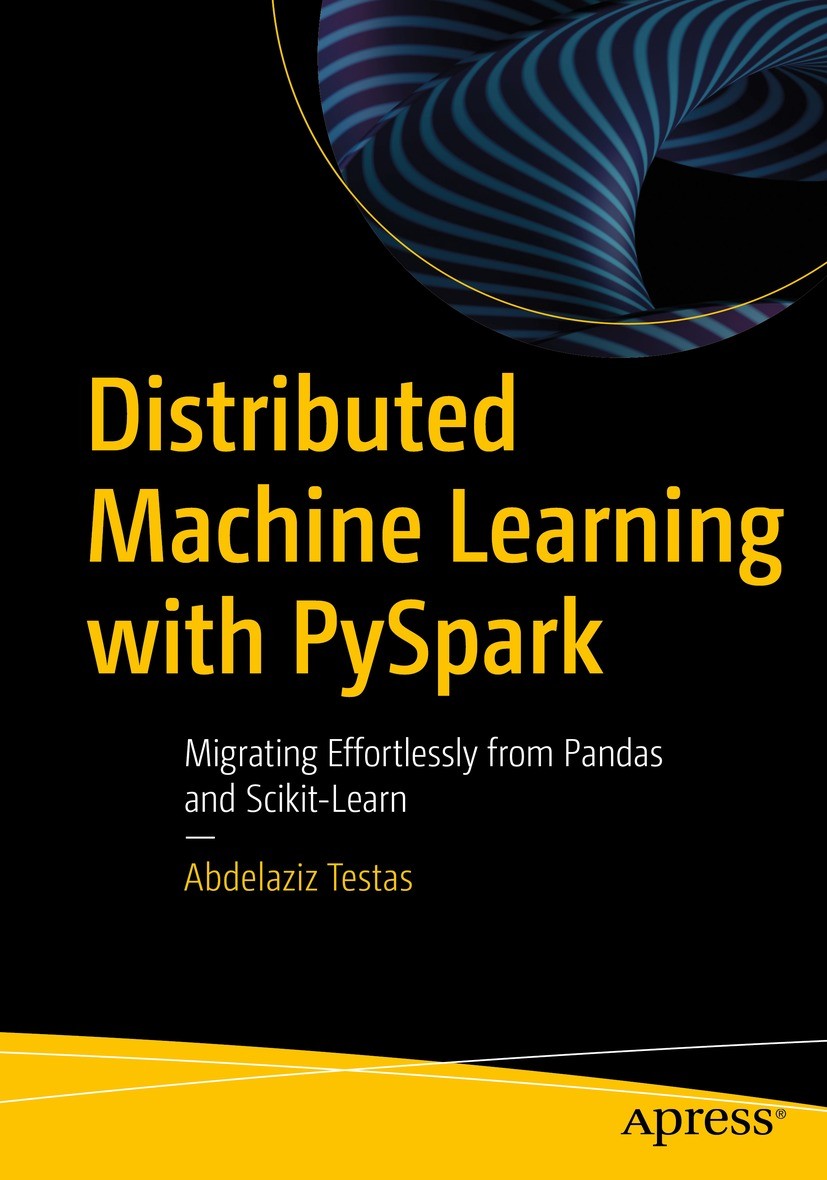 | 描述 | .Migrate from pandas and scikit-learn to PySpark to handle vast amounts of data and achieve faster data processing time. This book will show you how to make this transition by adapting your skills and leveraging the similarities in syntax, functionality, and interoperability between these tools...Distributed Machine Learning with PySpark. offers a roadmap to data scientists considering transitioning from small data libraries (pandas/scikit-learn) to big data processing and machine learning with PySpark. You will learn to translate Python code from pandas/scikit-learn to PySpark to preprocess large volumes of data and build, train, test, and evaluate popular machine learning algorithms such as linear and logistic regression, decision trees, random forests, support vector machines, Naïve Bayes, and neural networks...After completing this book, you will understand the foundational concepts of data preparation and machine learning and will have the skills necessary toapply these methods using PySpark, the industry standard for building scalable ML data pipelines...What You Will Learn..Master the fundamentals of supervised learning, unsupervised learning, NLP, and recommender systems.Un | 出版日期 | Book 2023 | 关键词 | Python; Scalable machine learning; Large-Scale machine learning; Machine Learning; PySpark; Scikit-learn; | 版次 | 1 | doi | https://doi.org/10.1007/978-1-4842-9751-3 | isbn_softcover | 978-1-4842-9750-6 | isbn_ebook | 978-1-4842-9751-3 | copyright | Abdelaziz Testas 2023 |
The information of publication is updating
|
|