书目名称 | Disruptive Technologies for Big Data and Cloud Applications |
副标题 | Proceedings of ICBDC |
编辑 | J. Dinesh Peter,Steven Lawrence Fernandes,Amir H. |
视频video | |
概述 | Gathers peer-reviewed proceedings of the International Conference on Big Data and Cloud Computing.Presents not only state of the art in cloud computing technologies and big data.Provides recent innova |
丛书名称 | Lecture Notes in Electrical Engineering |
图书封面 | 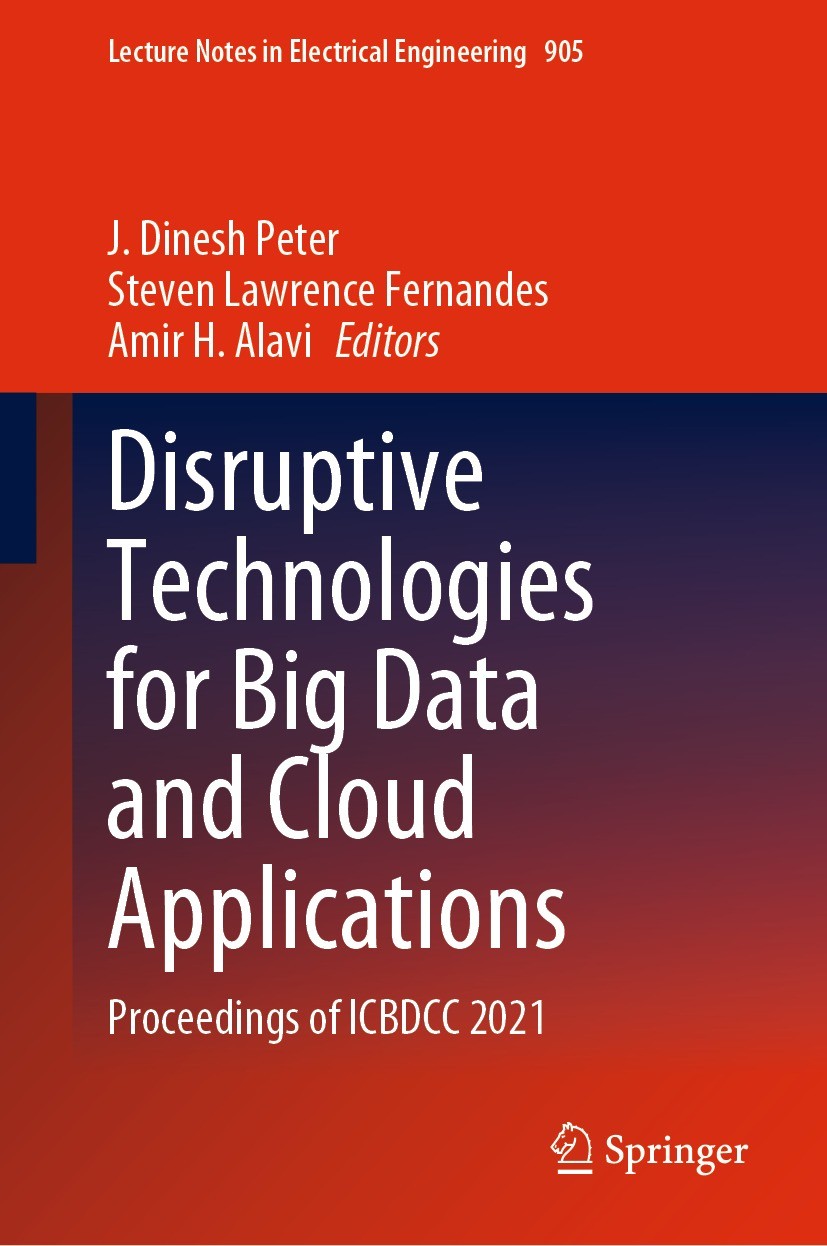 |
描述 | .This book provides a written record of the synergy that already exists among the research communities and represents a solid framework in the advancement of big data and cloud computing disciplines from which new interaction will result in the future. This book is a compendium of the International Conference on Big Data and Cloud Computing (ICBDCC 2021). It includes recent advances in big data analytics, cloud computing, the Internet of nano things, cloud security, data analytics in the cloud, smart cities and grids, etc. This book primarily focuses on the application of knowledge that promotes ideas for solving the problems of society through cutting-edge technologies. The articles featured in this book provide novel ideas that contribute to the growth of world-class research and development. The contents of this book are of interest to researchers and professionals alike.. |
出版日期 | Conference proceedings 2022 |
关键词 | Big Data; Data Analytics; Cloud Infrastructure for Big Data; Resource Scheduling; Data Models; Machine Le |
版次 | 1 |
doi | https://doi.org/10.1007/978-981-19-2177-3 |
isbn_ebook | 978-981-19-2177-3Series ISSN 1876-1100 Series E-ISSN 1876-1119 |
issn_series | 1876-1100 |
copyright | The Editor(s) (if applicable) and The Author(s), under exclusive license to Springer Nature Singapor |