书目名称 | Discovery Science | 副标题 | 16th International C | 编辑 | Johannes Fürnkranz,Eyke Hüllermeier,Tomoyuki Higuc | 视频video | | 概述 | Conference proceedings of the International Conference on Discovery Science, DS 2013 | 丛书名称 | Lecture Notes in Computer Science | 图书封面 | 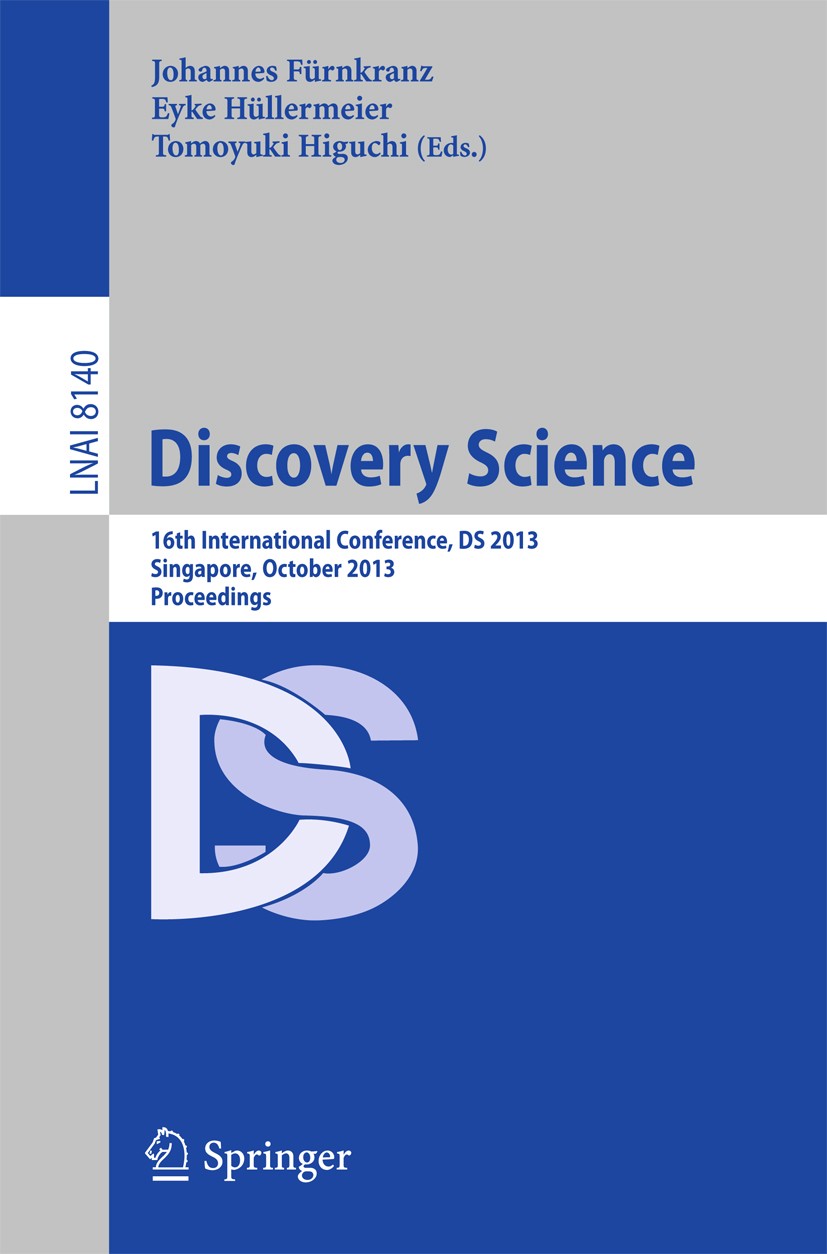 | 描述 | This book constitutes the proceedings of the 16th International Conference on Discovery Science, DS 2013, held in Singapore in October 2013, and co-located with the International Conference on Algorithmic Learning Theory, ALT 2013. The 23 papers presented in this volume were carefully reviewed and selected from 52 submissions. They cover recent advances in the development and analysis of methods of automatic scientific knowledge discovery, machine learning, intelligent data analysis, and their application to knowledge discovery. | 出版日期 | Conference proceedings 2013 | 关键词 | constraint-based clustering; domain ontology; hypernetworks; semantic data mining; structure learning; al | 版次 | 1 | doi | https://doi.org/10.1007/978-3-642-40897-7 | isbn_softcover | 978-3-642-40896-0 | isbn_ebook | 978-3-642-40897-7Series ISSN 0302-9743 Series E-ISSN 1611-3349 | issn_series | 0302-9743 | copyright | Springer-Verlag Berlin Heidelberg 2013 |
The information of publication is updating
|
|