书目名称 | Dimensionality Reduction in Data Science | 编辑 | Max Garzon,Ching-Chi Yang,Lih-Yuan Deng | 视频video | | 概述 | Presents‘a comprehensive review of datasets and solutions methods, with worked out applications to important problems that can be scaled to other data science problems and big datasets.An Ariadne’s th | 图书封面 | 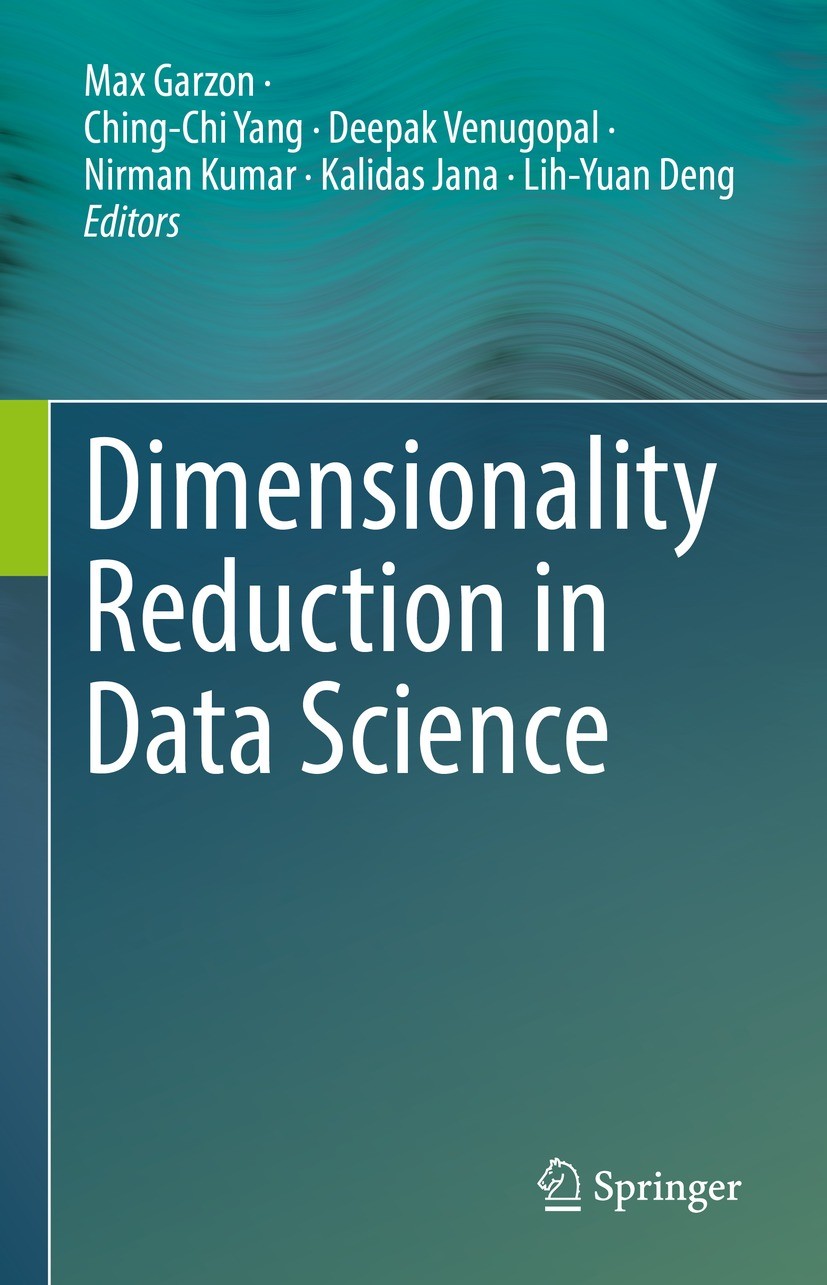 | 描述 | This book provides a practical and fairly comprehensive review of Data Science through the lens of dimensionality reduction, as well as hands-on techniques to tackle problems with data collected in the real world. State-of-the-art results and solutions from statistics, computer science and mathematics are explained from the point of view of a practitioner in any domain science, such as biology, cyber security, chemistry, sports science and many others. Quantitative and qualitative assessment methods are described to implement and validate the solutions back in the real world where the problems originated..The ability to generate, gather and store volumes of data in the order of tera- and exo bytes daily has far outpaced our ability to derive useful information with available computational resources for many domains..This book focuses on data science and problem definition, data cleansing, feature selection and extraction,statistical, geometric, information-theoretic, biomolecular and machine learning methods for dimensionality reduction of big datasets and problem solving, as well as a comparative assessment of solutions in a real-world setting..This book targets professionals work | 出版日期 | Book 2022 | 关键词 | Classification/Prediction; Cross-validation; Data science platforms; Data visualization; Deep learning/B | 版次 | 1 | doi | https://doi.org/10.1007/978-3-031-05371-9 | isbn_softcover | 978-3-031-05373-3 | isbn_ebook | 978-3-031-05371-9 | copyright | The Editor(s) (if applicable) and The Author(s), under exclusive license to Springer Nature Switzerl |
The information of publication is updating
|
|