书目名称 | Differential Privacy and Applications |
编辑 | Tianqing Zhu,Gang Li,Philip S. Yu |
视频video | |
概述 | Presents differential privacy in a more comprehensive style.Provides detailed coverage on differential privacy in the perspective of engineering rather than computing theory.Includes examples on vario |
丛书名称 | Advances in Information Security |
图书封面 | 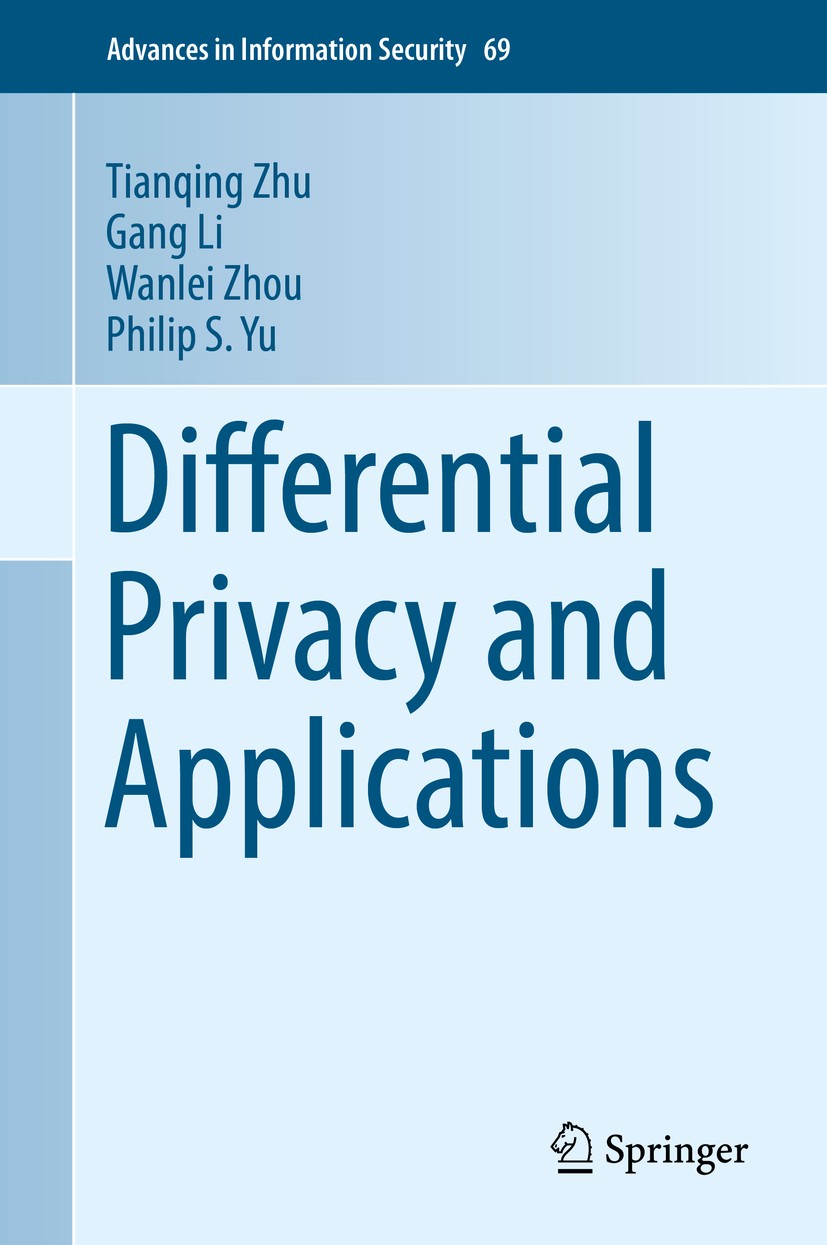 |
描述 | .This book focuses on differential privacy and its application with an emphasis on technical and application aspects. This book also presents the most recent research on differential privacy with a theory perspective. It provides an approachable strategy for researchers and engineers to implement differential privacy in real world applications..Early chapters are focused on two major directions, differentially private data publishing and differentially private data analysis. Data publishing focuses on how to modify the original dataset or the queries with the guarantee of differential privacy. Privacy data analysis concentrates on how to modify the data analysis algorithm to satisfy differential privacy, while retaining a high mining accuracy. The authors also introduce several applications in real world applications, including recommender systems and location privacy. .Advanced level students in computer science and engineering, as well as researchers and professionals working in privacy preserving, data mining, machine learning and data analysis will find this book useful as a reference. Engineers in database, network security, social networks and web services will also find this |
出版日期 | Book 2017 |
关键词 | data analysis; data mining; data release; differential policy; location privacy; machine learning; privacy |
版次 | 1 |
doi | https://doi.org/10.1007/978-3-319-62004-6 |
isbn_softcover | 978-3-319-87211-7 |
isbn_ebook | 978-3-319-62004-6Series ISSN 1568-2633 Series E-ISSN 2512-2193 |
issn_series | 1568-2633 |
copyright | Springer International Publishing AG 2017 |