书目名称 | Deep Reinforcement Learning with Python | 副标题 | With PyTorch, Tensor | 编辑 | Nimish Sanghi | 视频video | | 概述 | Explains deep reinforcement learning implementation using TensorFlow, PyTorch and OpenAI Gym..Covers deep reinforcement implementation using CNN and deep q-networks.Explains deep-q learning and policy | 图书封面 | 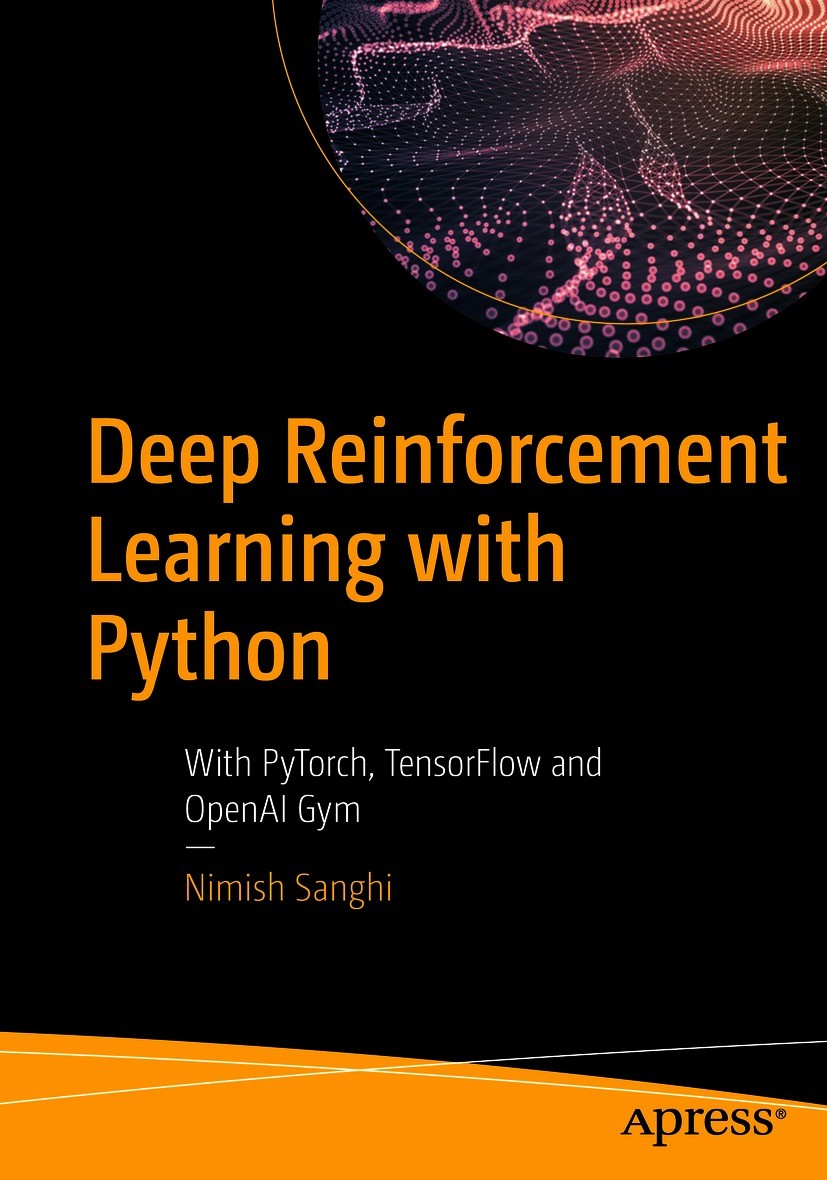 | 描述 | Deep reinforcement learning is a fast-growing discipline that is making a significant impact in fields of autonomous vehicles, robotics, healthcare, finance, and many more. This book covers deep reinforcement learning using deep-q learning and policy gradient models with coding exercise..You‘ll begin by reviewing the Markov decision processes, Bellman equations, and dynamic programming that form the core concepts and foundation of deep reinforcement learning. Next, you‘ll study model-free learning followed by function approximation using neural networks and deep learning. This is followed by various deep reinforcement learning algorithms such as deep q-networks, various flavors of actor-critic methods, and other policy-based methods. .You‘ll also look at exploration vs exploitation dilemma, a key consideration in reinforcement learning algorithms, along with Monte Carlo tree search (MCTS), which played a key role inthe success of AlphaGo. The final chapters conclude with deep reinforcement learning implementation using popular deep learning frameworks such as TensorFlow and PyTorch. In the end, you‘ll understand deep reinforcement learning along with deep q networks and policy grad | 出版日期 | Book 20211st edition | 关键词 | Artificial Intelligence; Deep Reinforcement Learning; PyTorch; Neural Networks; Robotics; Autonomous Vehi | 版次 | 1 | doi | https://doi.org/10.1007/978-1-4842-6809-4 | isbn_ebook | 978-1-4842-6809-4 | copyright | Nimish Sanghi 2021 |
The information of publication is updating
|
|