书目名称 | Deep Neural Networks in a Mathematical Framework | 编辑 | Anthony L. Caterini,Dong Eui Chang | 视频video | | 丛书名称 | SpringerBriefs in Computer Science | 图书封面 | 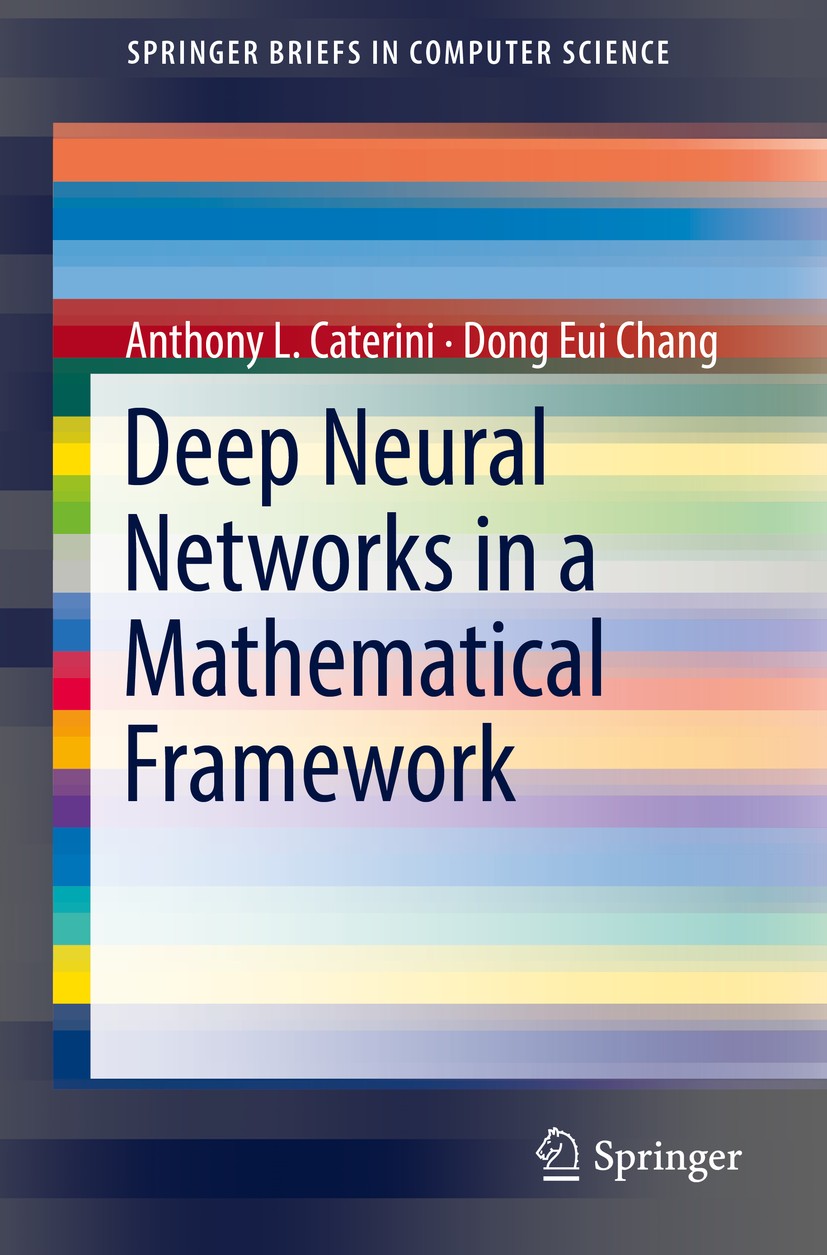 | 描述 | .This SpringerBrief describes how to build a rigorous end-to-end mathematical framework for deep neural networks. The authors provide tools to represent and describe neural networks, casting previous results in the field in a more natural light. In particular, the authors derive gradient descent algorithms in a unified way for several neural network structures, including multilayer perceptrons, convolutional neural networks, deep autoencoders and recurrent neural networks. Furthermore, the authors developed framework is both more concise and mathematically intuitive than previous representations of neural networks..This SpringerBrief is one step towards unlocking the .black box .of Deep Learning. The authors believe that this framework will help catalyze further discoveries regarding the mathematical properties of neural networks.This SpringerBrief is accessible not only to researchers, professionals and students working and studying in the field of deep learning, but alsoto those outside of the neutral network community.. | 出版日期 | Book 2018 | 关键词 | deep learning; machine learning; neural networks; multilayer perceptron; convolutional neural networks; r | 版次 | 1 | doi | https://doi.org/10.1007/978-3-319-75304-1 | isbn_softcover | 978-3-319-75303-4 | isbn_ebook | 978-3-319-75304-1Series ISSN 2191-5768 Series E-ISSN 2191-5776 | issn_series | 2191-5768 | copyright | The Author(s) 2018 |
The information of publication is updating
|
|