书目名称 | Deep Learning in Multi-step Prediction of Chaotic Dynamics |
副标题 | From Deterministic M |
编辑 | Matteo Sangiorgio,Fabio Dercole,Giorgio Guariso |
视频video | |
丛书名称 | SpringerBriefs in Applied Sciences and Technology |
图书封面 | 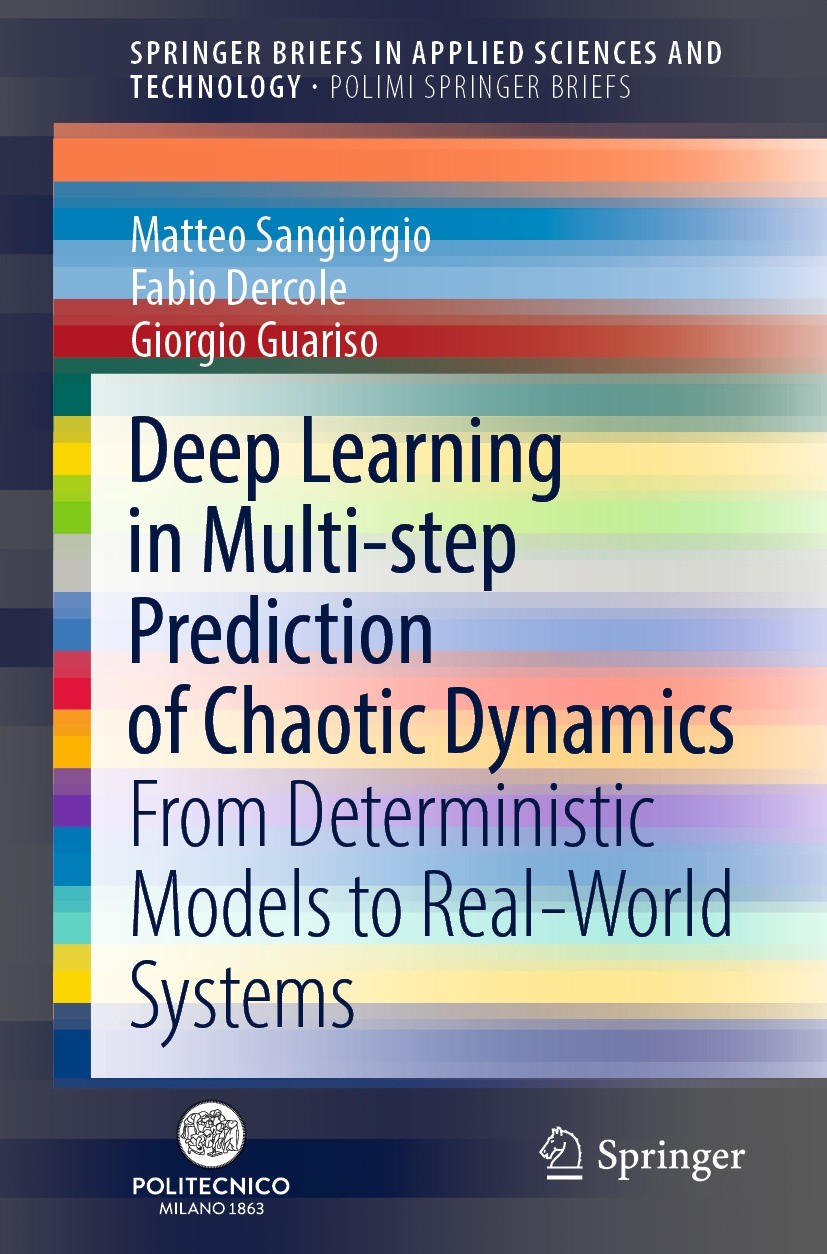 |
描述 | .The book represents the first attempt to systematically deal with the use of deep neural networks to forecast chaotic time series. Differently from most of the current literature, it implements a multi-step approach, i.e., the forecast of an entire interval of future values. This is relevant for many applications, such as model predictive control, that requires predicting the values for the whole receding horizon. Going progressively from deterministic models with different degrees of complexity and chaoticity to noisy systems and then to real-world cases, the book compares the performances of various neural network architectures (feed-forward and recurrent). It also introduces an innovative and powerful approach for training recurrent structures specific for sequence-to-sequence tasks. The book also presents one of the first attempts in the context of environmental time series forecasting of applying transfer-learning techniques such as domain adaptation.. |
出版日期 | Book 2021 |
关键词 | Chaotic attractors; Neural network training; Recurrent neural networks; Henon systems; Exposure bias; env |
版次 | 1 |
doi | https://doi.org/10.1007/978-3-030-94482-7 |
isbn_softcover | 978-3-030-94481-0 |
isbn_ebook | 978-3-030-94482-7Series ISSN 2191-530X Series E-ISSN 2191-5318 |
issn_series | 2191-530X |
copyright | The Author(s), under exclusive license to Springer Nature Switzerland AG 2021 |