书目名称 | Deep Learning for Hyperspectral Image Analysis and Classification |
编辑 | Linmi Tao,Atif Mughees |
视频video | |
概述 | Proposes adaptive-boundary adjustment-based noise detection and group-wise band categorization with unsupervised spectral-spatial adaptive band-noise factor-based formulation.Presents unsupervised spe |
丛书名称 | Engineering Applications of Computational Methods |
图书封面 | 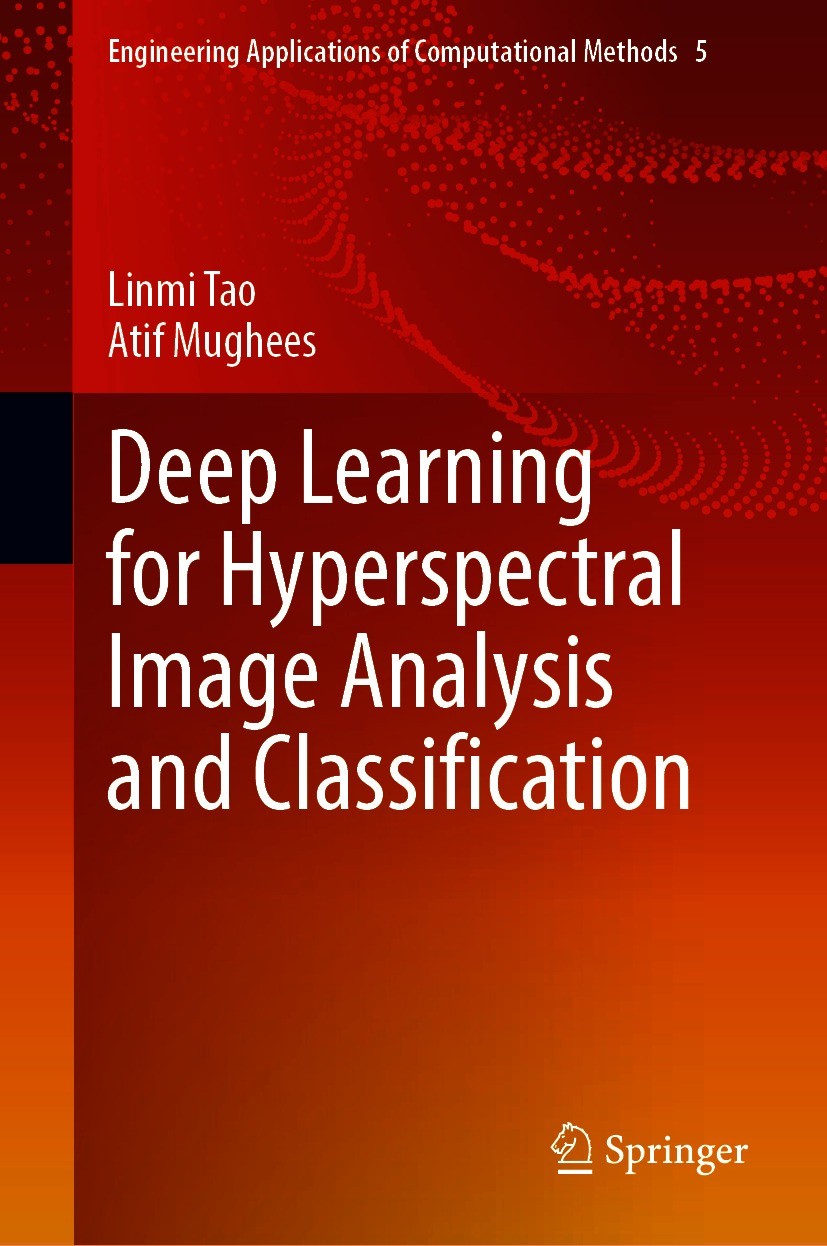 |
描述 | .This book focuses on deep learning-based methods for hyperspectral image (HSI) analysis. Unsupervised spectral-spatial adaptive band-noise factor-based formulation is devised for HSI noise detection and band categorization. The method to characterize the bands along with the noise estimation of HSIs will benefit subsequent remote sensing techniques significantly...This book develops on two fronts: On the one hand, it is aimed at domain professionals who want to have an updated overview of how hyperspectral acquisition techniques can combine with deep learning architectures to solve specific tasks in different application fields. On the other hand, the authors want to target the machine learning and computer vision experts by giving them a picture of how deep learning technologies are applied to hyperspectral data from a multidisciplinary perspective. The presence of these two viewpoints and the inclusion of application fields of remote sensing by deep learning are theoriginal contributions of this review, which also highlights some potentialities and critical issues related to the observed development trends.. |
出版日期 | Book 2021 |
关键词 | Remote sensing; Hyperspectral image analysis; Deep learning; Stacked auto-encoder; Deep belief network; S |
版次 | 1 |
doi | https://doi.org/10.1007/978-981-33-4420-4 |
isbn_softcover | 978-981-33-4422-8 |
isbn_ebook | 978-981-33-4420-4Series ISSN 2662-3366 Series E-ISSN 2662-3374 |
issn_series | 2662-3366 |
copyright | The Editor(s) (if applicable) and The Author(s), under exclusive license to Springer Nature Singapor |