书目名称 | Deep Learning and Physics |
编辑 | Akinori Tanaka,Akio Tomiya,Koji Hashimoto |
视频video | |
概述 | Is the first machine learning textbook written by physicists so that physicists and undergraduates can learn easily.Presents applications to physics problems written so that readers can soon imagine h |
丛书名称 | Mathematical Physics Studies |
图书封面 | 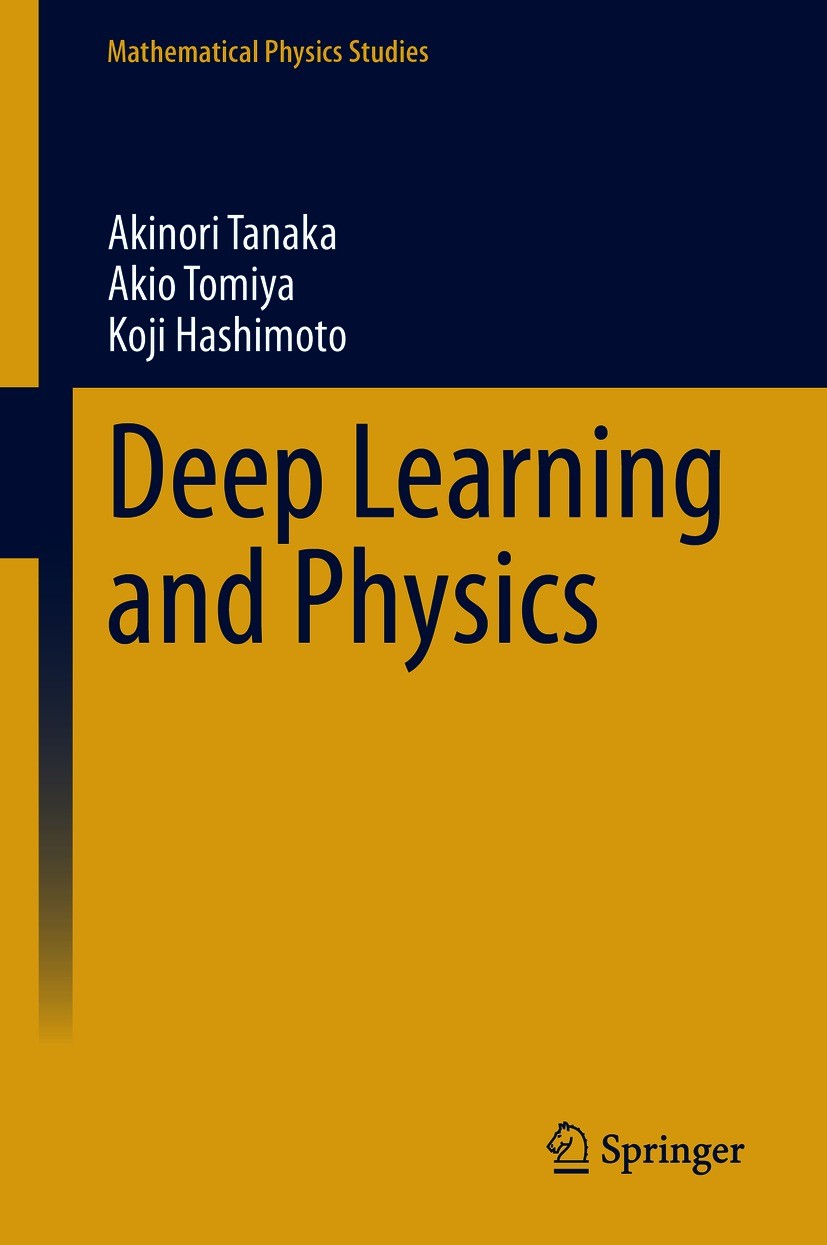 |
描述 | What is deep learning for those who study physics? Is it completely different from physics? Or is it similar? .In recent years, machine learning, including deep learning, has begun to be used in various physics studies. Why is that? Is knowing physics useful in machine learning? Conversely, is knowing machine learning useful in physics? .This book is devoted to answers of these questions. Starting with basic ideas of physics, neural networks are derived naturally. And you can learn the concepts of deep learning through the words of physics..In fact, the foundation of machine learning can be attributed to physical concepts. Hamiltonians that determine physical systems characterize various machine learning structures. Statistical physics given by Hamiltonians defines machine learning by neural networks. Furthermore, solving inverse problems in physics through machine learning and generalization essentially providesprogress and even revolutions in physics. For these reasons, in recent years interdisciplinary research in machine learning and physics has been expanding dramatically. .This book is written for anyone who wants to learn, understand, and apply the relationship between deep |
出版日期 | Book 2021 |
关键词 | Deep learning; Physics; Neural network; Applications to theoretical physics; Machine learning |
版次 | 1 |
doi | https://doi.org/10.1007/978-981-33-6108-9 |
isbn_softcover | 978-981-33-6110-2 |
isbn_ebook | 978-981-33-6108-9Series ISSN 0921-3767 Series E-ISSN 2352-3905 |
issn_series | 0921-3767 |
copyright | The Editor(s) (if applicable) and The Author(s), under exclusive licence to Springer Nature Singapor |