书目名称 | Deep Learning and Data Labeling for Medical Applications |
副标题 | First International |
编辑 | Gustavo Carneiro,Diana Mateus,Julien Cornebise |
视频video | |
概述 | Includes supplementary material: |
丛书名称 | Lecture Notes in Computer Science |
图书封面 | 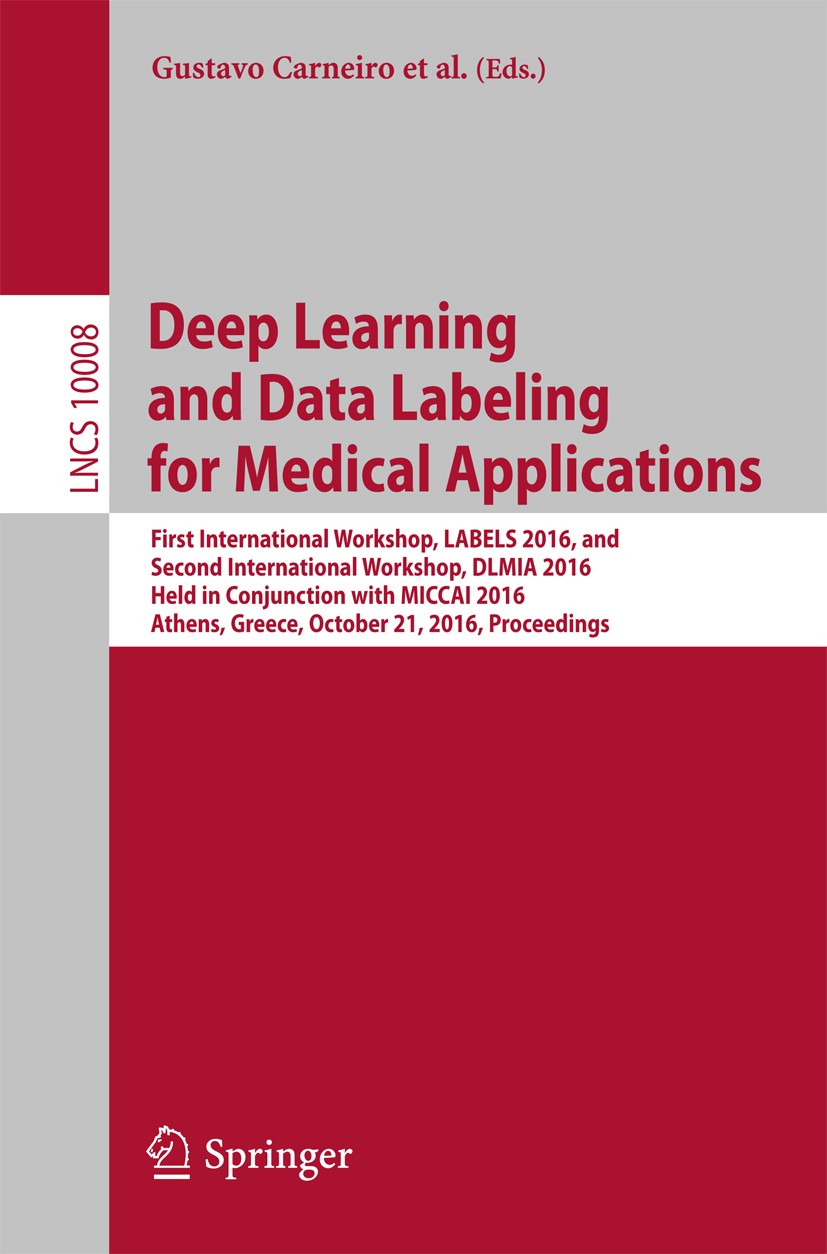 |
描述 | This book constitutes the refereed proceedings of two workshops held at the 19th International Conference on Medical Image Computing and Computer-Assisted Intervention, MICCAI 2016, in Athens, Greece, in October 2016: the First Workshop on Large-Scale Annotation of Biomedical Data and Expert Label Synthesis, LABELS 2016, and the Second International Workshop on Deep Learning in Medical Image Analysis, DLMIA 2016. The 28 revised regular papers presented in this book were carefully reviewed and selected from a total of 52 submissions. The 7 papers selected for LABELS deal with topics from the following fields: crowd-sourcing methods; active learning; transfer learning; semi-supervised learning; and modeling of label uncertainty.The 21 papers selected for DLMIA span a wide range of topics such as image description; medical imaging-based diagnosis; medical signal-based diagnosis; medical image reconstruction and model selection using deep learning techniques; meta-heuristic techniques for fine-tuning parameter in deep learning-based architectures; and applications based on deep learning techniques.. |
出版日期 | Conference proceedings 2016 |
关键词 | active learning; deep learning; human-computer interaction; label uncertainty; medical image analysis; an |
版次 | 1 |
doi | https://doi.org/10.1007/978-3-319-46976-8 |
isbn_softcover | 978-3-319-46975-1 |
isbn_ebook | 978-3-319-46976-8Series ISSN 0302-9743 Series E-ISSN 1611-3349 |
issn_series | 0302-9743 |
copyright | Springer International Publishing AG 2016 |