书目名称 | Deep Learning Foundations | 编辑 | Taeho Jo | 视频video | | 概述 | Provides a conceptual understanding of deep learning algorithms.Presents ways of modifying existing machine learning algorithms into deep learning algorithms for further analysis.Details how deep lear | 图书封面 | 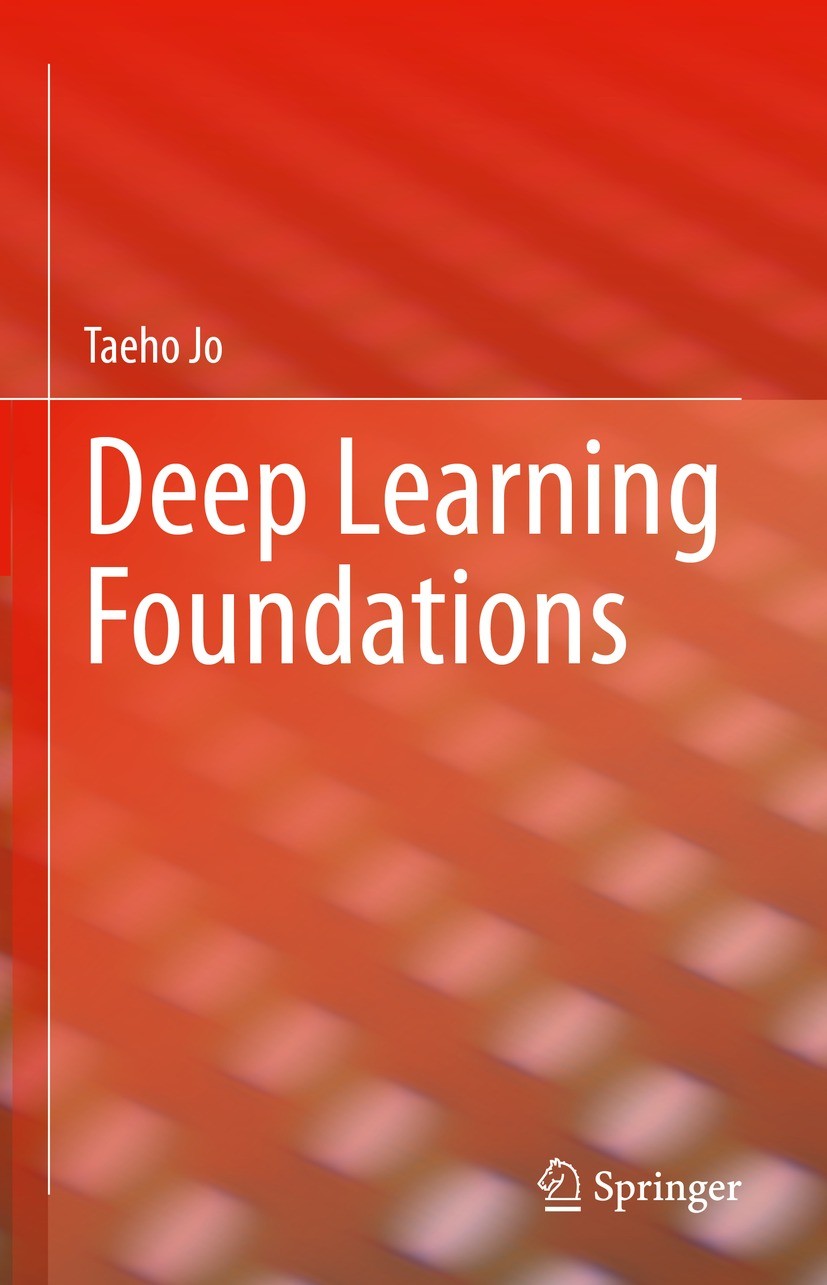 | 描述 | This book provides a conceptual understanding of deep learning algorithms. The book consists of the four parts: foundations, deep machine learning, deep neural networks, and textual deep learning. The first part provides traditional supervised learning, traditional unsupervised learning, and ensemble learning, as the preparation for studying deep learning algorithms. The second part deals with modification of existing machine learning algorithms into deep learning algorithms. The book’s third part deals with deep neural networks, such as Multiple Perceptron, Recurrent Networks, Restricted Boltzmann Machine, and Convolutionary Neural Networks. The last part provides deep learning techniques that are specialized for text mining tasks. The book is relevant for researchers, academics, students, and professionals in machine learning. | 出版日期 | Book 2023 | 关键词 | Deep Learning; Deep K nearest Neighbor; Deep Naïve Bayes; Deep Support Vector Machine; Multiple Layer Pe | 版次 | 1 | doi | https://doi.org/10.1007/978-3-031-32879-4 | isbn_softcover | 978-3-031-32881-7 | isbn_ebook | 978-3-031-32879-4 | copyright | The Editor(s) (if applicable) and The Author(s), under exclusive license to Springer Nature Switzerl |
The information of publication is updating
|
|