书目名称 | Data-Driven Prediction for Industrial Processes and Their Applications |
编辑 | Jun Zhao,Wei Wang,Chunyang Sheng |
视频video | |
概述 | Features data-driven modeling algorithms for different industrial prediction requirements.Discusses multi-scale (short, median, long) prediction, multi-type prediction (time series and factor-based), |
丛书名称 | Information Fusion and Data Science |
图书封面 | 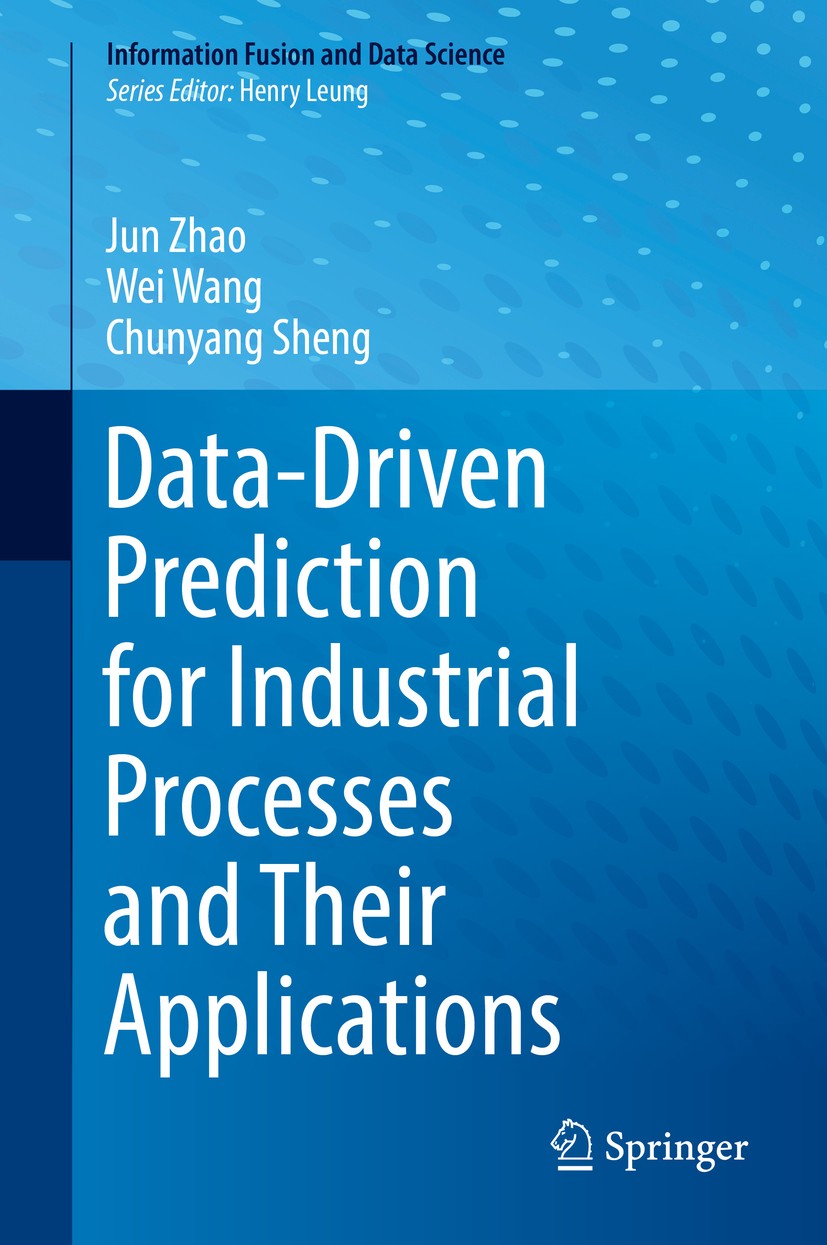 |
描述 | This book presents modeling methods and algorithms for data-driven prediction and forecasting of practical industrial process by employing machine learning and statistics methodologies. Related case studies, especially on energy systems in the steel industry are also addressed and analyzed. The case studies in this volume are entirely rooted in both classical data-driven prediction problems and industrial practice requirements. Detailed figures and tables demonstrate the effectiveness and generalization of the methods addressed, and the classifications of the addressed prediction problems come from practical industrial demands, rather than from academic categories. As such, readers will learn the corresponding approaches for resolving their industrial technical problems. Although the contents of this book and its case studies come from the steel industry, these techniques can be also used for other process industries. This book appeals to students, researchers, and professionals withinthe machine learning and data analysis and mining communities. |
出版日期 | Book 2018 |
关键词 | industrial time series prediction; prediction intervals for industrial data; long term prediction for |
版次 | 1 |
doi | https://doi.org/10.1007/978-3-319-94051-9 |
isbn_softcover | 978-3-030-06785-4 |
isbn_ebook | 978-3-319-94051-9Series ISSN 2510-1528 Series E-ISSN 2510-1536 |
issn_series | 2510-1528 |
copyright | Springer International Publishing AG, part of Springer Nature 2018 |