书目名称 | Data-Driven Fault Detection and Reasoning for Industrial Monitoring |
编辑 | Jing Wang,Jinglin Zhou,Xiaolu Chen |
视频video | |
概述 | Evaluates the practicality of data-driven methods in industrial process monitoring.Embeds manifold learning technology into multivariate statistical methods.Introduces partial least absolute technolog |
丛书名称 | Intelligent Control and Learning Systems |
图书封面 | 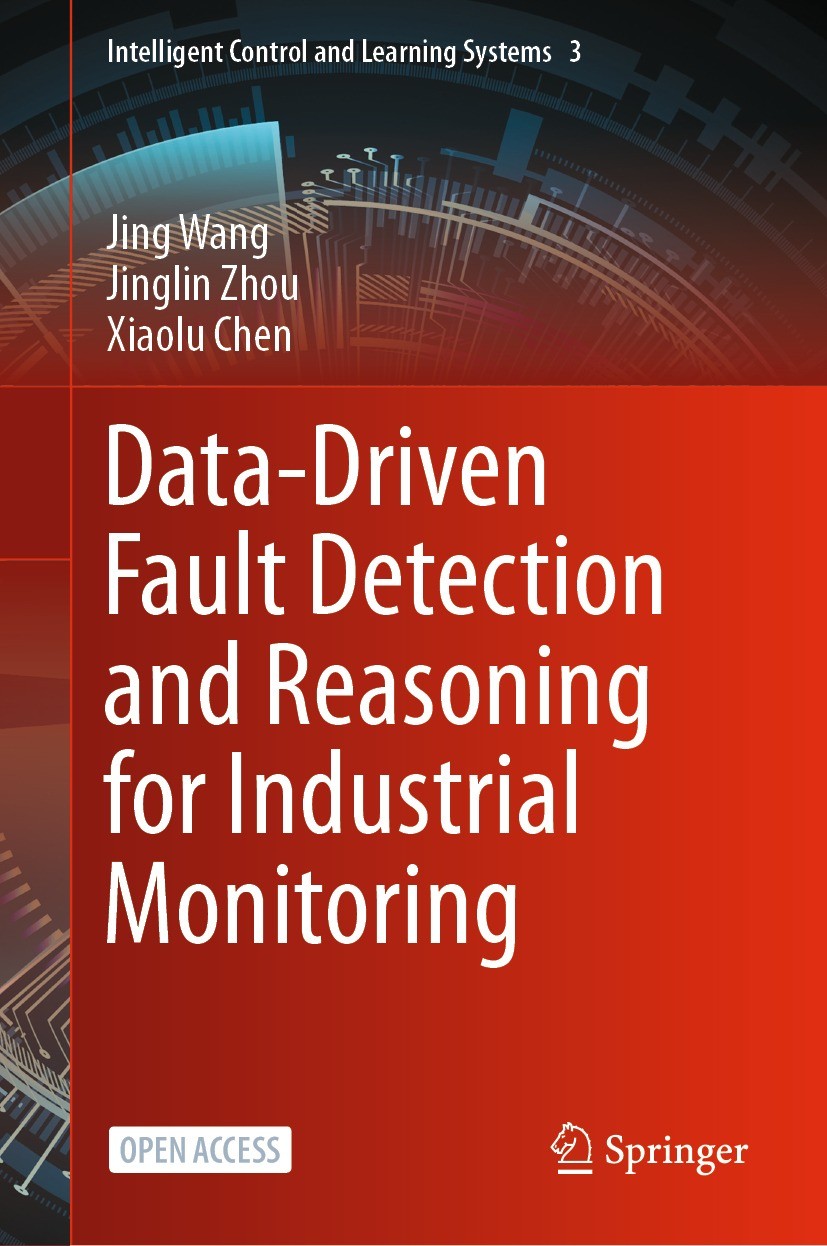 |
描述 | .This open access book assesses the potential of data-driven methods in industrial process monitoring engineering. The process modeling, fault detection, classification, isolation, and reasoning are studied in detail. These methods can be used to improve the safety and reliability of industrial processes. Fault diagnosis, including fault detection and reasoning, has attracted engineers and scientists from various fields such as control, machinery, mathematics, and automation engineering. Combining the diagnosis algorithms and application cases, this book establishes a basic framework for this topic and implements various statistical analysis methods for process monitoring. This book is intended for senior undergraduate and graduate students who are interested in fault diagnosis technology, researchers investigating automation and industrial security, professional practitioners and engineers working on engineering modeling and data processing applications. .This is an open access book.. |
出版日期 | Book‘‘‘‘‘‘‘‘ 2022 |
关键词 | Multivariate causality analysis; Process monitoring; Manifold learning; Fault diagnosis; Data modeling; F |
版次 | 1 |
doi | https://doi.org/10.1007/978-981-16-8044-1 |
isbn_softcover | 978-981-16-8046-5 |
isbn_ebook | 978-981-16-8044-1Series ISSN 2662-5458 Series E-ISSN 2662-5466 |
issn_series | 2662-5458 |
copyright | The Editor(s) (if applicable) and The Author(s) 2022 |