书目名称 | Data-Driven Evolutionary Optimization | 副标题 | Integrating Evolutio | 编辑 | Yaochu Jin,Handing Wang,Chaoli Sun | 视频video | | 概述 | Includes a brief introduction to mathematical programming, metaheuristic algorithms, and machine learning techniques.Presents a systematic description of most recent research advances in data-driven e | 丛书名称 | Studies in Computational Intelligence | 图书封面 | 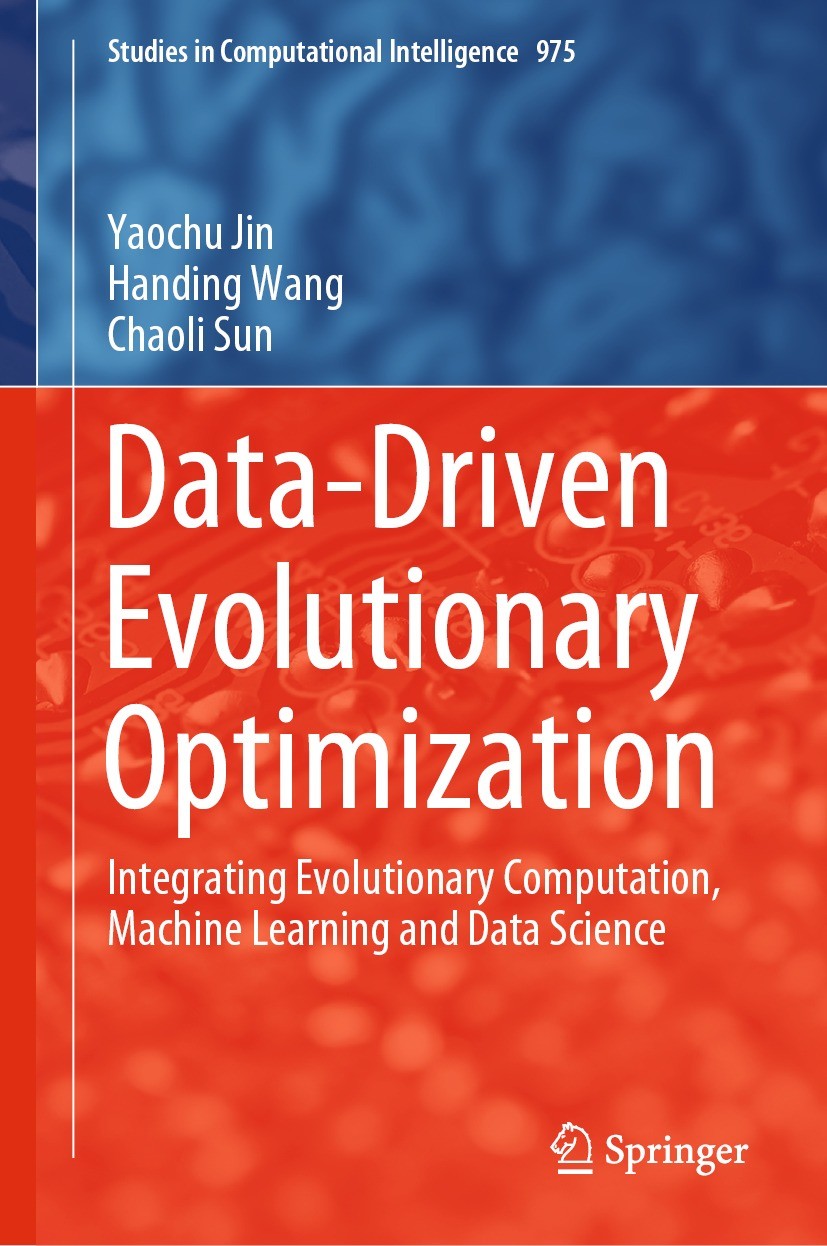 | 描述 | .Intended for researchers and practitioners alike, this book covers carefully selected yet broad topics in optimization, machine learning, and metaheuristics. Written by world-leading academic researchers who are extremely experienced in industrial applications, this self-contained book is the first of its kind that provides comprehensive background knowledge, particularly practical guidelines, and state-of-the-art techniques. New algorithms are carefully explained, further elaborated with pseudocode or flowcharts, and full working source code is made freely available. ..This is followed by a presentation of a variety of data-driven single- and multi-objective optimization algorithms that seamlessly integrate modern machine learning such as deep learning and transfer learning with evolutionary and swarm optimization algorithms. Applications of data-driven optimization ranging from aerodynamic design, optimization of industrial processes, to deep neural architecture search are included.. | 出版日期 | Book 2021 | 关键词 | Data-Driven Evolutionary Optimization; Evolutionary Optimization; Computational Intelligence; Metaheuri | 版次 | 1 | doi | https://doi.org/10.1007/978-3-030-74640-7 | isbn_softcover | 978-3-030-74642-1 | isbn_ebook | 978-3-030-74640-7Series ISSN 1860-949X Series E-ISSN 1860-9503 | issn_series | 1860-949X | copyright | The Editor(s) (if applicable) and The Author(s), under exclusive license to Springer Nature Switzerl |
The information of publication is updating
|
|