书目名称 | Data Wrangling with R | 编辑 | Bradley C. Boehmke, Ph.D. | 视频video | | 概述 | Presents techniques that allow users to spend less time obtaining, cleaning, manipulating, and preprocessing data and more time visualizing, analyzing, and presenting data via a step-by-step tutorial | 丛书名称 | Use R! | 图书封面 | 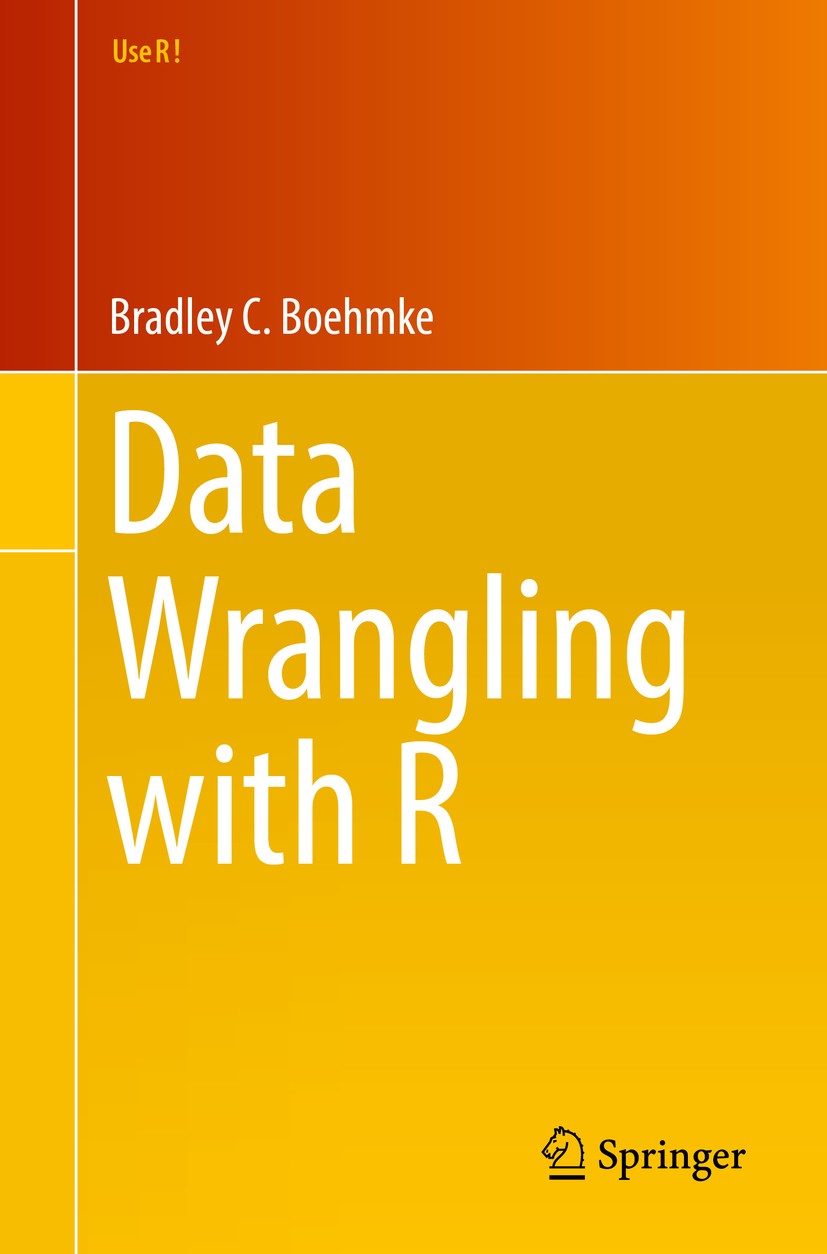 | 描述 | .This guide for practicing statisticians, data scientists, and R users and programmers will teach the essentials of preprocessing: data leveraging the R programming language to easily and quickly turn noisy data into usable pieces of information. Data wrangling, which is also commonly referred to as data munging, transformation, manipulation, janitor work, etc., can be a painstakingly laborious process. Roughly 80% of data analysis is spent on cleaning and preparing data; however, being a prerequisite to the rest of the data analysis workflow (visualization, analysis, reporting), it is essential that one become fluent and efficient in data wrangling techniques..This book will guide the user through the data wrangling process via a step-by-step tutorial approach and provide a solid foundation for working with data in R. The author‘s goal is to teach the user how to easily wrangle data in order to spend more time on understanding the content of the data. By the end of the book, the user will have learned: .How to work with different types of data such as numerics, characters, regular expressions, factors, and dates.The difference between different data structures and how to create, a | 出版日期 | Book 2016 | 关键词 | R; data wrangling; data structures; dplyr; tidyr; importing; scraping; exporting; coding; data frames; data ma | 版次 | 1 | doi | https://doi.org/10.1007/978-3-319-45599-0 | isbn_softcover | 978-3-319-45598-3 | isbn_ebook | 978-3-319-45599-0Series ISSN 2197-5736 Series E-ISSN 2197-5744 | issn_series | 2197-5736 | copyright | Springer International Publishing Switzerland 2016 |
The information of publication is updating
|
|