书目名称 | Data Stream Management | 副标题 | Processing High-Spee | 编辑 | Minos Garofalakis,Johannes Gehrke,Rajeev Rastogi | 视频video | | 概述 | Comprehensive introduction to the algorithmic and theoretical foundations of data stream processing – from basic mathematical models, algorithms, and analytics, and progressing to more advanced stream | 丛书名称 | Data-Centric Systems and Applications | 图书封面 | 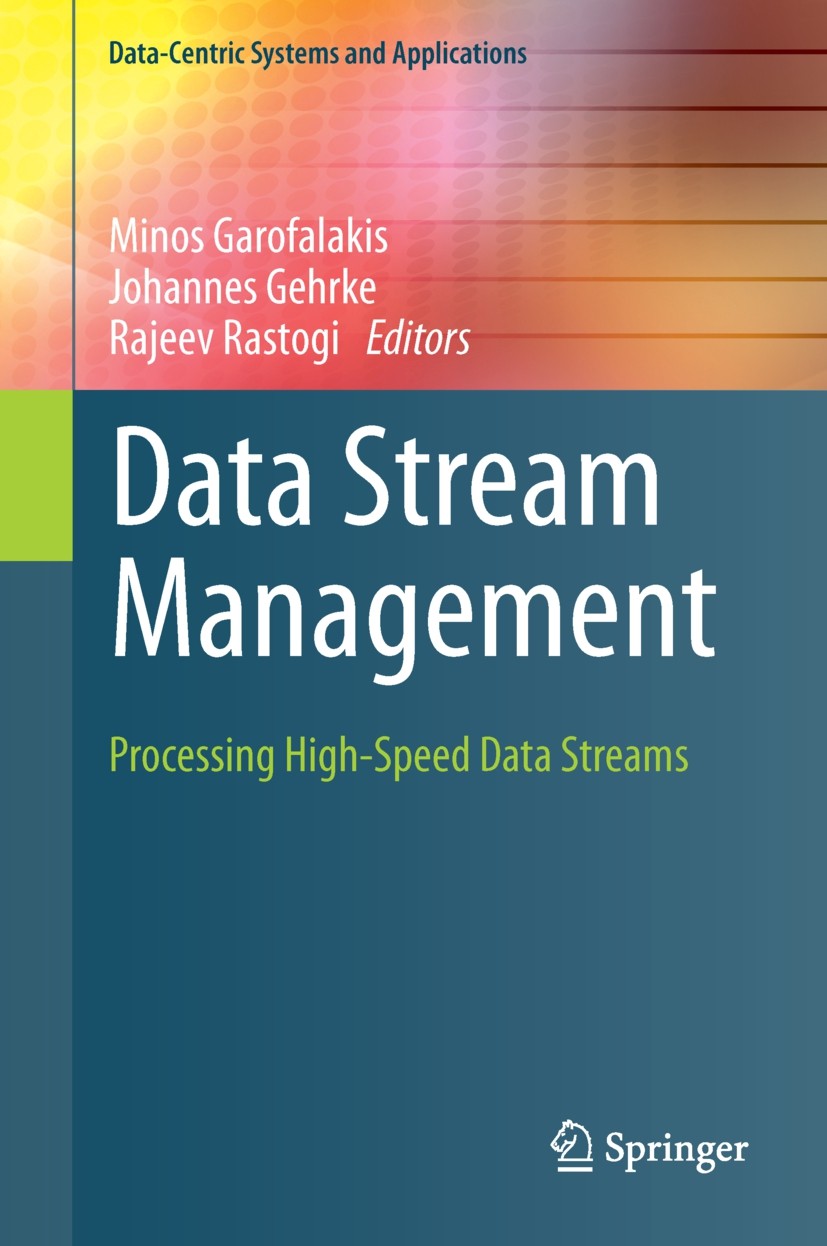 | 描述 | .This volume focuses on the theory and practice of .data stream management., and the novel challenges this emerging domain poses for data-management algorithms, systems, and applications. The collection of chapters, contributed by authorities in the field, offers a comprehensive introduction to both the algorithmic/theoretical foundations of data streams, as well as the streaming systems and applications built in different domains..A short introductory chapter provides a brief summary of some basic data streaming concepts and models, and discusses the key elements of a generic stream query processing architecture. Subsequently, Part I focuses on basic streaming algorithms for some key analytics functions (e.g., quantiles, norms, join aggregates, heavy hitters) over streaming data. Part II then examines important techniques for basic stream mining tasks (e.g., clustering, classification, frequent itemsets). Part III discusses a number of advanced topics on stream processingalgorithms, and Part IV focuses on system and language aspects of data stream processing with surveys of influential system prototypes and language designs. Part V then presents some representative applications of | 出版日期 | Textbook 2016 | 关键词 | Data streams; Data model extensions; Database management system engines; Data mining; Sensor networks; XM | 版次 | 1 | doi | https://doi.org/10.1007/978-3-540-28608-0 | isbn_softcover | 978-3-662-56837-8 | isbn_ebook | 978-3-540-28608-0Series ISSN 2197-9723 Series E-ISSN 2197-974X | issn_series | 2197-9723 | copyright | Springer-Verlag Berlin Heidelberg 2016 |
The information of publication is updating
|
|