书目名称 | Data Science and Artificial Intelligence |
副标题 | First International |
编辑 | Chutiporn Anutariya,Marcello M. Bonsangue |
视频video | |
丛书名称 | Communications in Computer and Information Science |
图书封面 | 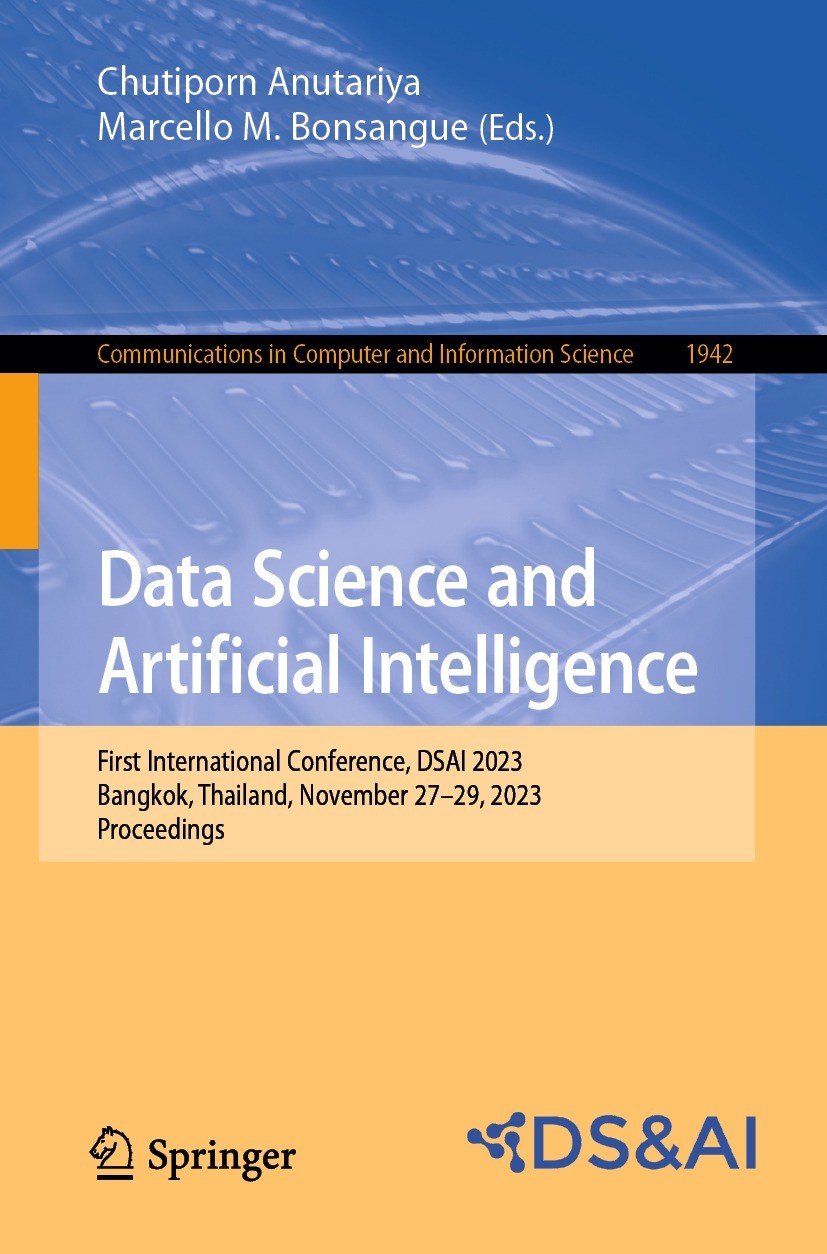 |
描述 | This book constitutes the proceedings of the First International Conference, DSAI 2023, held in Bangkok, Thailand, during November 27–30, 2023..The 22 full papers and the 4 short papers included in this volume were carefully reviewed and selected from 70 submissions. This volume focuses on ideas, methodologies, and cutting-edge research that can drive progress and foster interdisciplinary collaboration in the fields of data science and artificial intelligence. |
出版日期 | Conference proceedings 2023 |
关键词 | foundations of data science and artificial intelligence; data security; machine learning; deep learning |
版次 | 1 |
doi | https://doi.org/10.1007/978-981-99-7969-1 |
isbn_softcover | 978-981-99-7968-4 |
isbn_ebook | 978-981-99-7969-1Series ISSN 1865-0929 Series E-ISSN 1865-0937 |
issn_series | 1865-0929 |
copyright | The Editor(s) (if applicable) and The Author(s), under exclusive license to Springer Nature Singapor |