书目名称 | Data Mining in Crystallography | 编辑 | D. W. M. Hofmann,Liudmila N. Kuleshova | 视频video | | 概述 | This series presents critical reviews of the present position and future trends in modern chemical research concerned with chemical structure and bonding.Short and concise reports, each written by the | 丛书名称 | Structure and Bonding | 图书封面 | 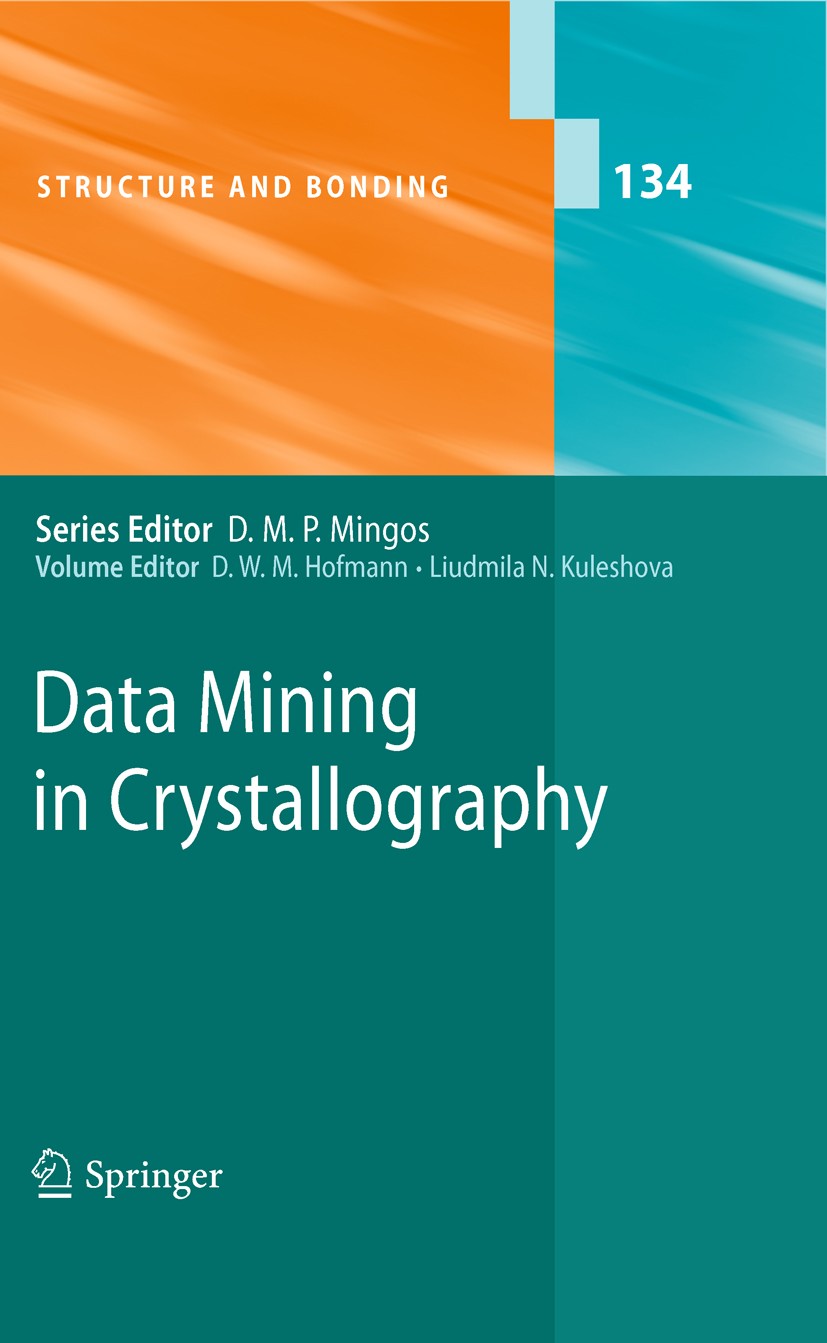 | 描述 | Humans have been “manually” extracting patterns from data for centuries, but the increasing volume of data in modern times has called for more automatic approaches. Early methods of identifying patterns in data include Bayes’ theorem (1700s) and Regression analysis (1800s). The proliferation, ubiquity and incre- ing power of computer technology has increased data collection and storage. As data sets have grown in size and complexity, direct hands-on data analysis has - creasingly been augmented with indirect, automatic data processing. Data mining has been developed as the tool for extracting hidden patterns from data, by using computing power and applying new techniques and methodologies for knowledge discovery. This has been aided by other discoveries in computer science, such as Neural networks, Clustering, Genetic algorithms (1950s), Decision trees (1960s) and Support vector machines (1980s). Data mining commonlyinvolves four classes of tasks: • Classi cation: Arranges the data into prede ned groups. For example, an e-mail program might attempt to classify an e-mail as legitimate or spam. Common algorithmsinclude Nearest neighbor,Naive Bayes classi er and Neural network. • Clus | 出版日期 | Book 2010 | 关键词 | Data Basis; Protein Structure; Secondary structure; clustering; crystallography; data analysis; data minin | 版次 | 1 | doi | https://doi.org/10.1007/978-3-642-04759-6 | isbn_softcover | 978-3-642-26161-9 | isbn_ebook | 978-3-642-04759-6Series ISSN 0081-5993 Series E-ISSN 1616-8550 | issn_series | 0081-5993 | copyright | Springer-Verlag Berlin Heidelberg 2010 |
The information of publication is updating
|
|