书目名称 | Data Mining |
副标题 | 15th Australasian Co |
编辑 | Yee Ling Boo,David Stirling,Graham Williams |
视频video | |
丛书名称 | Communications in Computer and Information Science |
图书封面 | 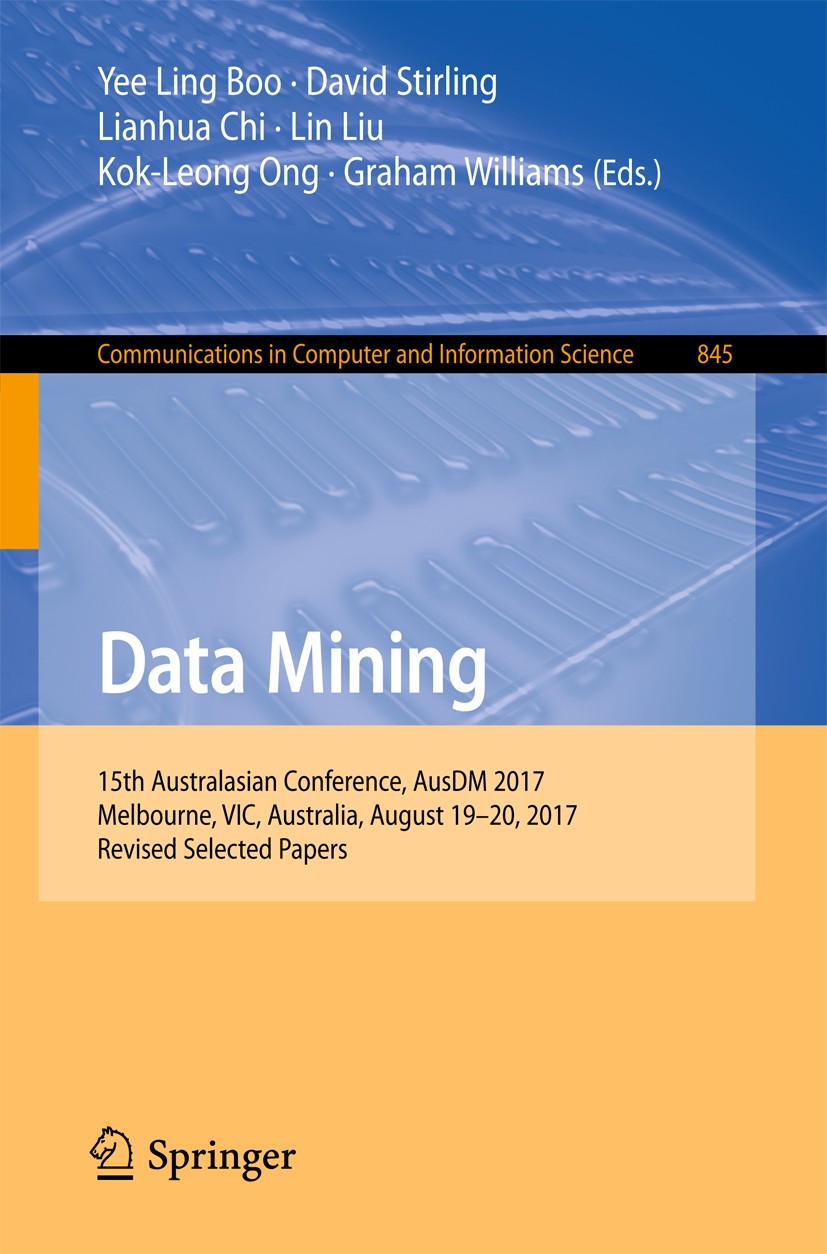 |
描述 | .This book constitutes the refereed proceedings of the 15th Australasian Conference on Data Mining, AusDM 2017, held in Melbourne, VIC, Australia, in August 2017..The 17 revised full papers presented together with 11 research track papers and 6 application track papers were carefully reviewed and selected from 31 submissions. The papers are organized in topical sections on clustering and classification; big data; time series; outlier detection and applications; social media and applications.. |
出版日期 | Conference proceedings 2018 |
关键词 | artificial intelligence; classification; data mining; decision trees; machine learning; signal processing |
版次 | 1 |
doi | https://doi.org/10.1007/978-981-13-0292-3 |
isbn_softcover | 978-981-13-0291-6 |
isbn_ebook | 978-981-13-0292-3Series ISSN 1865-0929 Series E-ISSN 1865-0937 |
issn_series | 1865-0929 |
copyright | Springer Nature Singapore Pte Ltd. 2018 |