书目名称 | Composing Fisher Kernels from Deep Neural Models |
副标题 | A Practitioner‘s App |
编辑 | Tayyaba Azim,Sarah Ahmed |
视频video | |
概述 | Presents a step-by-step approach to deriving a kernel from any probabilistic model belonging to the family of deep networks.Demonstrates the use of feature compression and selection techniques for red |
丛书名称 | SpringerBriefs in Computer Science |
图书封面 | 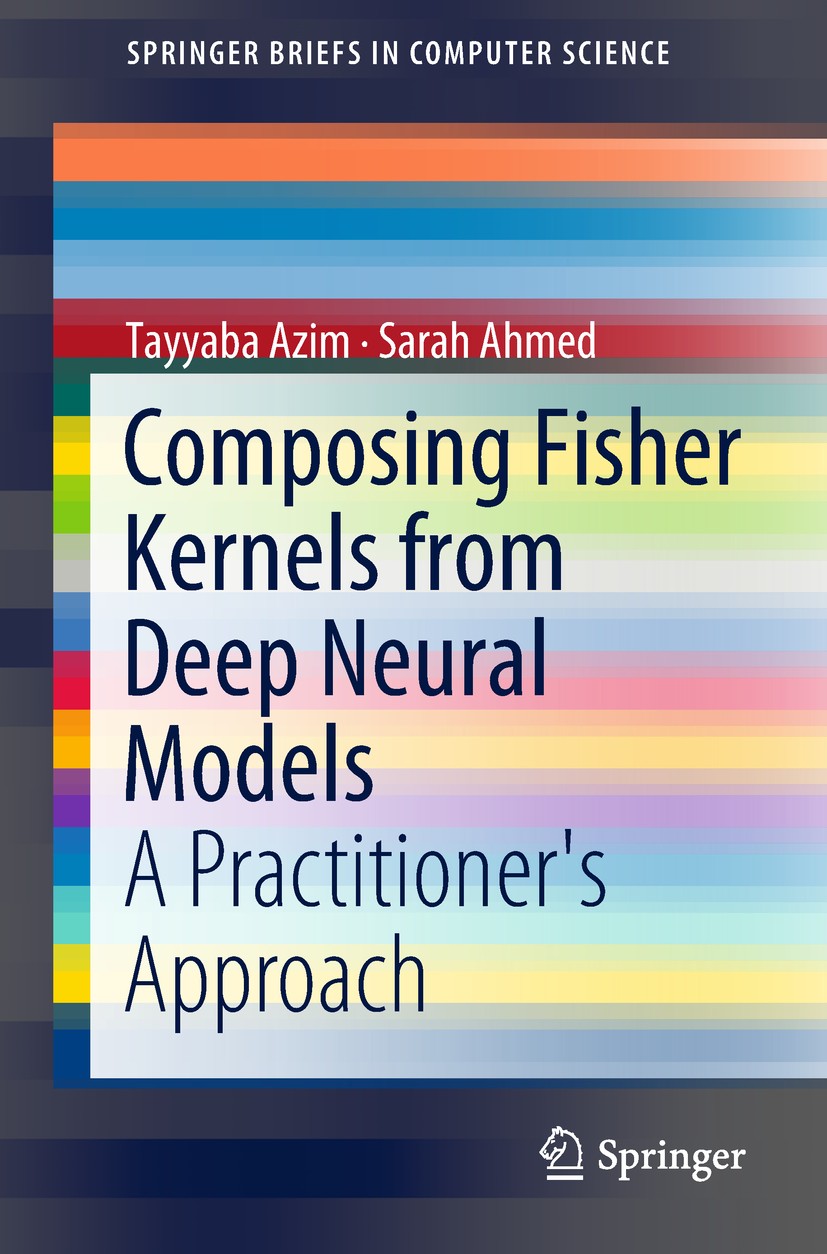 |
描述 | This book shows machine learning enthusiasts and practitioners how to get the best of both worlds by deriving Fisher kernels from deep learning models. In addition, the book shares insight on how to store and retrieve large-dimensional Fisher vectors using feature selection and compression techniques. Feature selection and feature compression are two of the most popular off-the-shelf methods for reducing data’s high-dimensional memory footprint and thus making it suitable for large-scale visual retrieval and classification. Kernel methods long remained the .de facto. standard for solving large-scale object classification tasks using low-level features, until the revival of deep models in 2006. Later, they made a comeback with improved Fisher vectors in 2010. However, their supremacy was always challenged by various versions of deep models, now considered to be the state of the art for solving various machine learning and computer vision tasks. Although the two research paradigms differ significantly, the excellent performance of Fisher kernels on the Image Net large-scale object classification dataset has caught the attention of numerous kernel practitioners, and many have drawn pa |
出版日期 | Book 2018 |
关键词 | Deep Models; Fisher Vectors; Large Scale Information Retrieval; Feature Compression Techniques; Feature |
版次 | 1 |
doi | https://doi.org/10.1007/978-3-319-98524-4 |
isbn_softcover | 978-3-319-98523-7 |
isbn_ebook | 978-3-319-98524-4Series ISSN 2191-5768 Series E-ISSN 2191-5776 |
issn_series | 2191-5768 |
copyright | The Author(s), under exclusive licence to Springer Nature Switzerland AG 2018 |