期刊全称 | Artificial Neural Networks and Machine Learning – ICANN 2024 | 期刊简称 | 33rd International C | 影响因子2023 | Michael Wand,Kristína Malinovská,Igor V. Tetko | 视频video | | 学科分类 | Lecture Notes in Computer Science | 图书封面 | 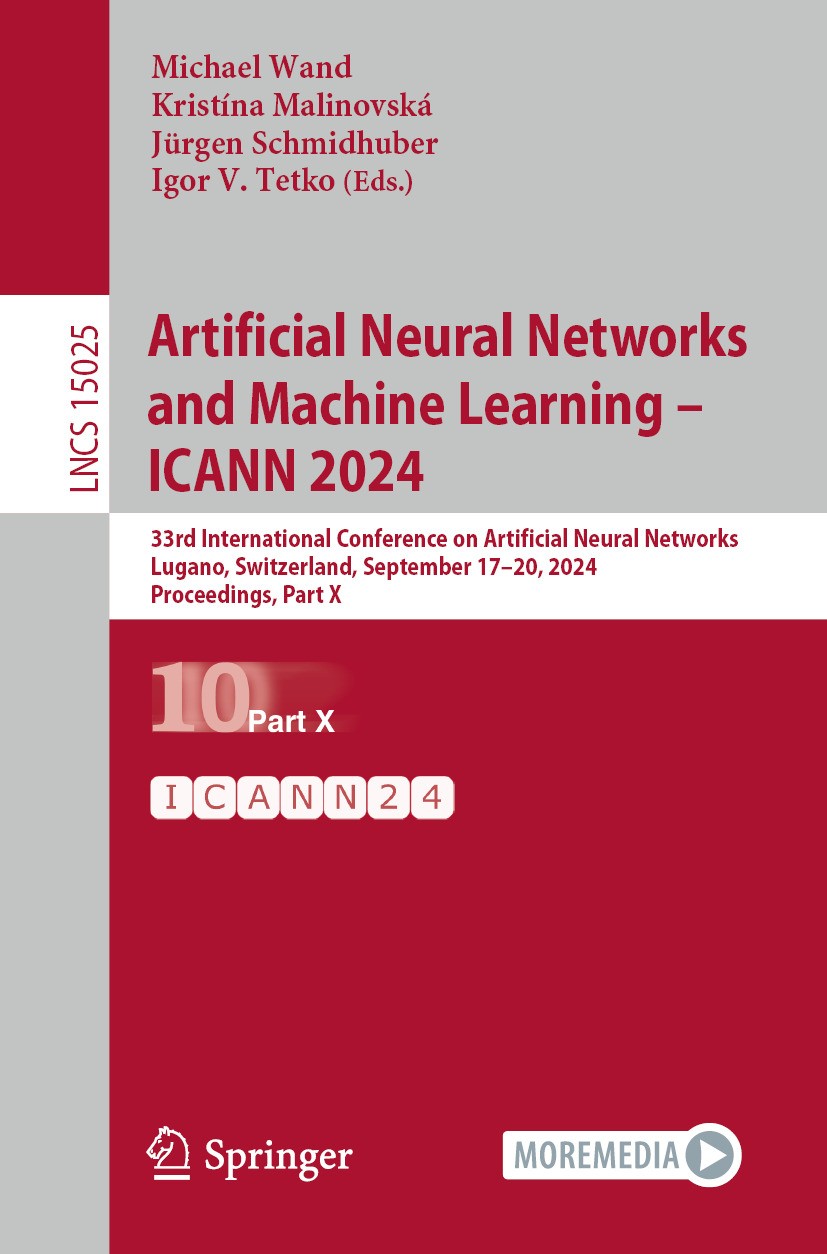 | 影响因子 | .The ten-volume set LNCS 15016-15025 constitutes the refereed proceedings of the 33rd International Conference on Artificial Neural Networks and Machine Learning, ICANN 2024, held in Lugano, Switzerland, during September 17–20, 2024...The 294 full papers and 16 short papers included in these proceedings were carefully reviewed and selected from 764 submissions. The papers cover the following topics: ..Part I - theory of neural networks and machine learning; novel methods in machine learning; novel neural architectures; neural architecture search; self-organization; neural processes; novel architectures for computer vision; and fairness in machine learning...Part II - computer vision: classification; computer vision: object detection; computer vision: security and adversarial attacks; computer vision: image enhancement; and computer vision: 3D methods...Part III - computer vision: anomaly detection; computer vision: segmentation; computer vision: pose estimation and tracking; computer vision: video processing; computer vision: generative methods; and topics in computer vision...Part IV - brain-inspired computing; cognitive and computational neuroscience; explainable artificial intel | Pindex | Conference proceedings 2024 |
The information of publication is updating
|
|