期刊全称 | Automated Deep Learning Using Neural Network Intelligence | 期刊简称 | Develop and Design P | 影响因子2023 | Ivan Gridin | 视频video | http://file.papertrans.cn/167/166283/166283.mp4 | 发行地址 | Covers application of the latest scientific advances in neural network design.Presents a clear and visual representation of neural architecture search concepts.Includes boosting of PyTorch and TensorF | 图书封面 | 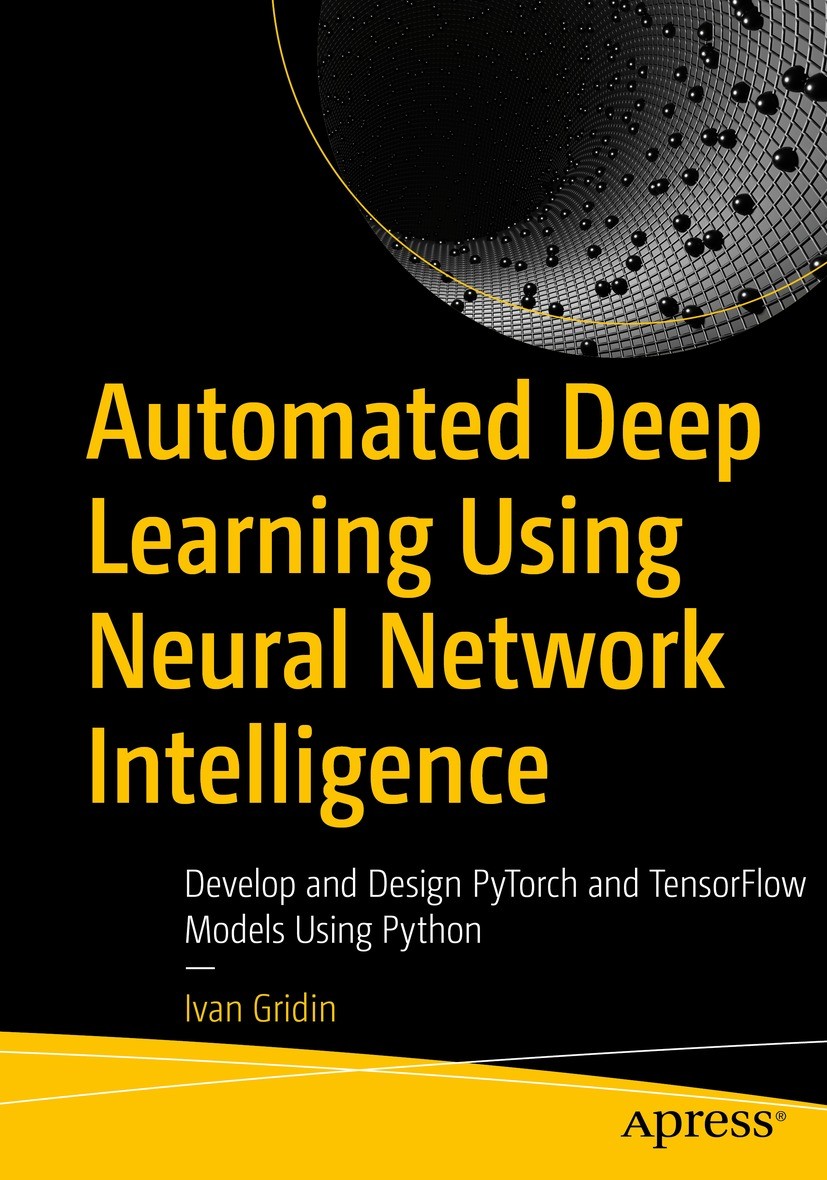 | 影响因子 | .Optimize, develop, and design PyTorch and TensorFlow models for a specific problem using the Microsoft Neural Network Intelligence (NNI) toolkit. This book includes practical examples illustrating automated deep learning approaches and provides techniques to facilitate your deep learning model development.. . The first chapters of this book cover the basics of NNI toolkit usage and methods for solving hyper-parameter optimization tasks. You will understand the black-box function maximization problem using NNI, and know how to prepare a TensorFlow or PyTorch model for hyper-parameter tuning, launch an experiment, and interpret the results. The book dives into optimization tuners and the search algorithms they are based on: Evolution search, Annealing search, and the Bayesian Optimization approach. The Neural Architecture Search is covered and you will learn how to develop deep learning models from scratch. Multi-trial and one-shot searching approaches of automatic neural network design are presented. The book teaches you how to construct a search space and launch an architecture search using the latest state-of-the-art exploration strategies: Efficient Neural Architecture Search (E | Pindex | Book 2022 |
The information of publication is updating
|
|