期刊全称 | Artificial Neural Networks - ICANN 2007 | 期刊简称 | 17th International C | 影响因子2023 | Joaquim Marques Sá,Luís A. Alexandre,Danilo Mandic | 视频video | | 学科分类 | Lecture Notes in Computer Science | 图书封面 | 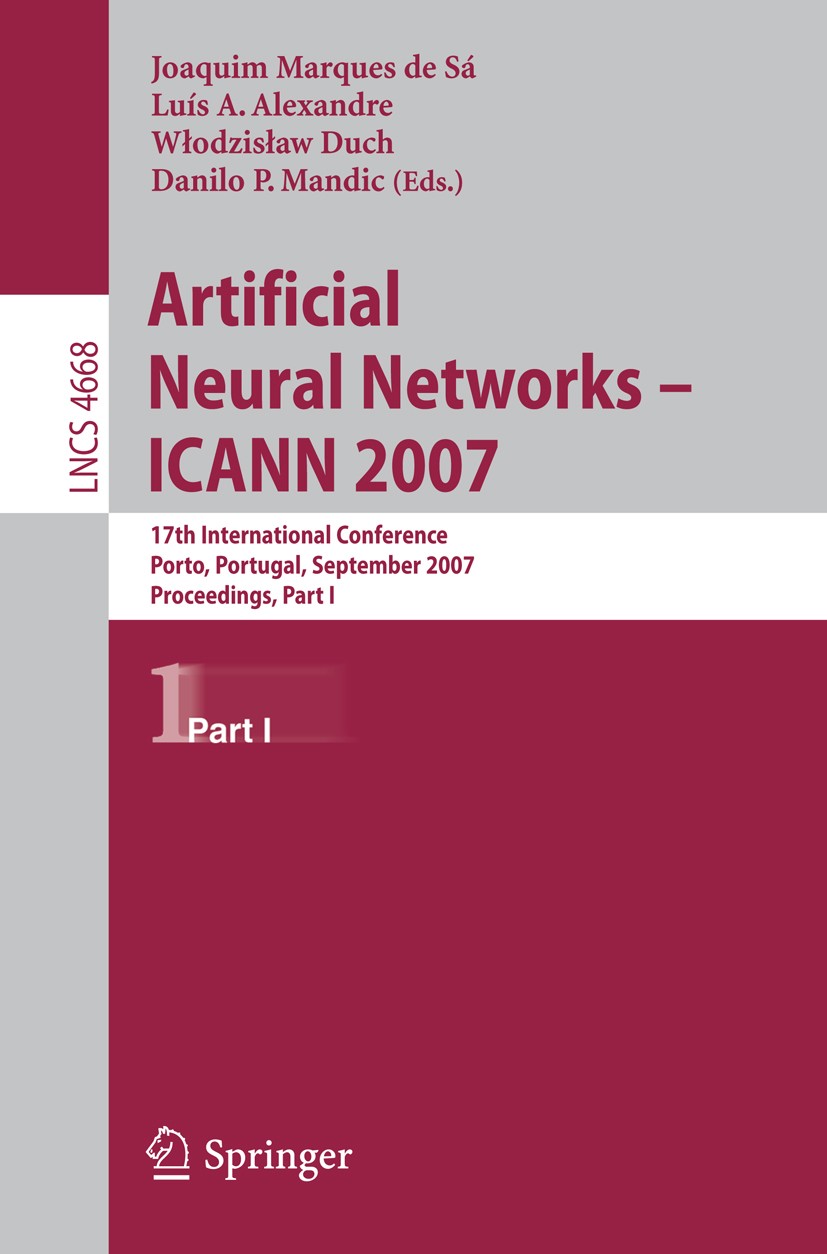 | 影响因子 | .This two volume set LNCS 4668 and LNCS 4669 constitutes the refereed proceedings of the 17th International Conference on Artificial Neural Networks, ICANN 2007, held in Porto, Portugal, in September 2007...The 197 revised full papers presented were carefully reviewed and selected from 376 submissions. The 98 papers of the first volume are organized in topical sections on learning theory, advances in neural network learning methods, ensemble learning, spiking neural networks, advances in neural network architectures neural network technologies, neural dynamics and complex systems, data analysis, estimation, spatial and spatio-temporal learning, evolutionary computing, meta learning, agents learning, complex-valued neural networks, as well as temporal synchronization and nonlinear dynamics in neural networks.. | Pindex | Conference proceedings 2007 |
The information of publication is updating
|
|