期刊全称 | Artificial Neural Networks and Machine Learning – ICANN 2022 | 期刊简称 | 31st International C | 影响因子2023 | Elias Pimenidis,Plamen Angelov,Mehmet Aydin | 视频video | | 学科分类 | Lecture Notes in Computer Science | 图书封面 | 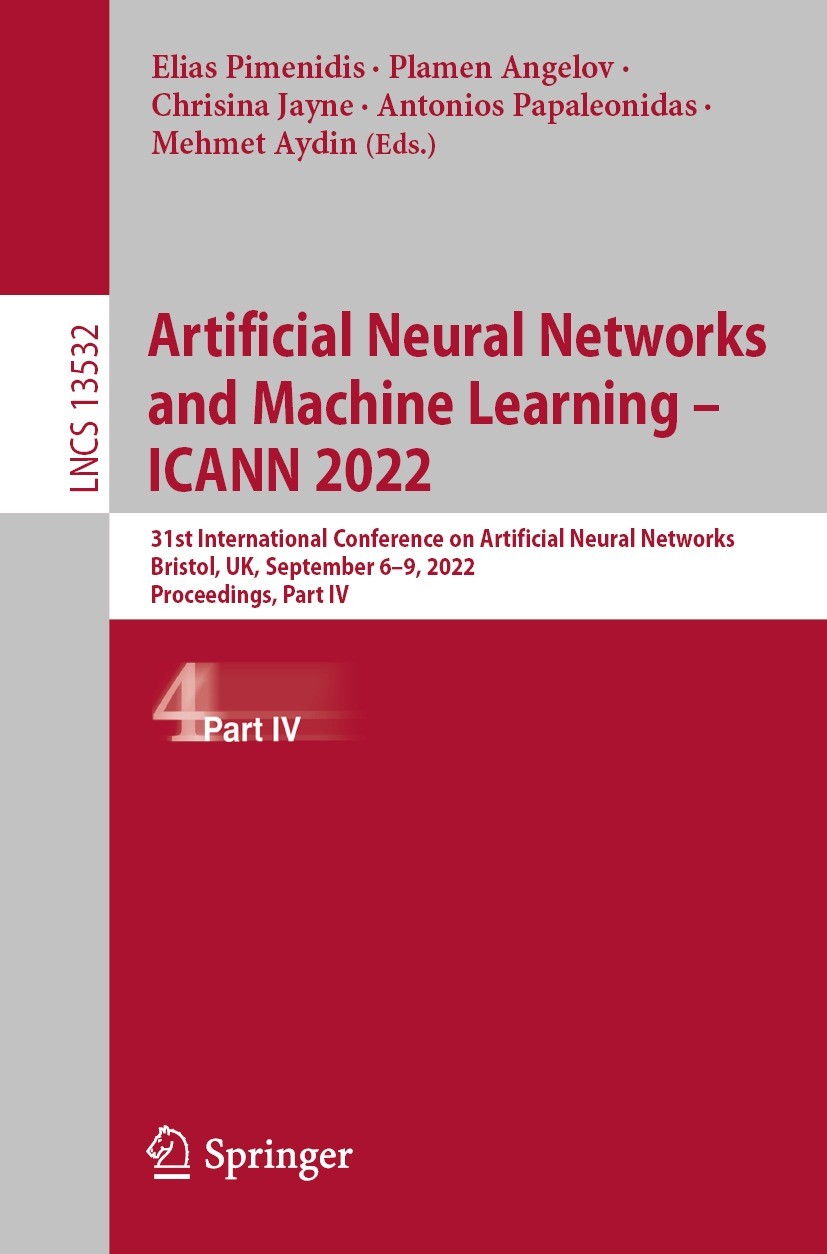 | 影响因子 | .The 4-volumes set of LNCS 13529, 13530, 13531, and 13532 constitutes the proceedings of the 31st International Conference on Artificial Neural Networks, ICANN 2022, held in Bristol, UK, in September 2022.. The total of 255 full papers presented in these proceedings was carefully reviewed and selected from 561 submissions. ICANN 2022 is a dual-track conference featuring tracks in brain inspired computing and machine learning and artificial neural networks, with strong cross-disciplinary interactions and applications.. | Pindex | Conference proceedings 2022 |
The information of publication is updating
|
|