期刊全称 | Artificial Neural Networks and Machine Learning – ICANN 2019: Image Processing | 期刊简称 | 28th International C | 影响因子2023 | Igor V. Tetko,Věra Kůrková,Fabian Theis | 视频video | | 学科分类 | Lecture Notes in Computer Science | 图书封面 | 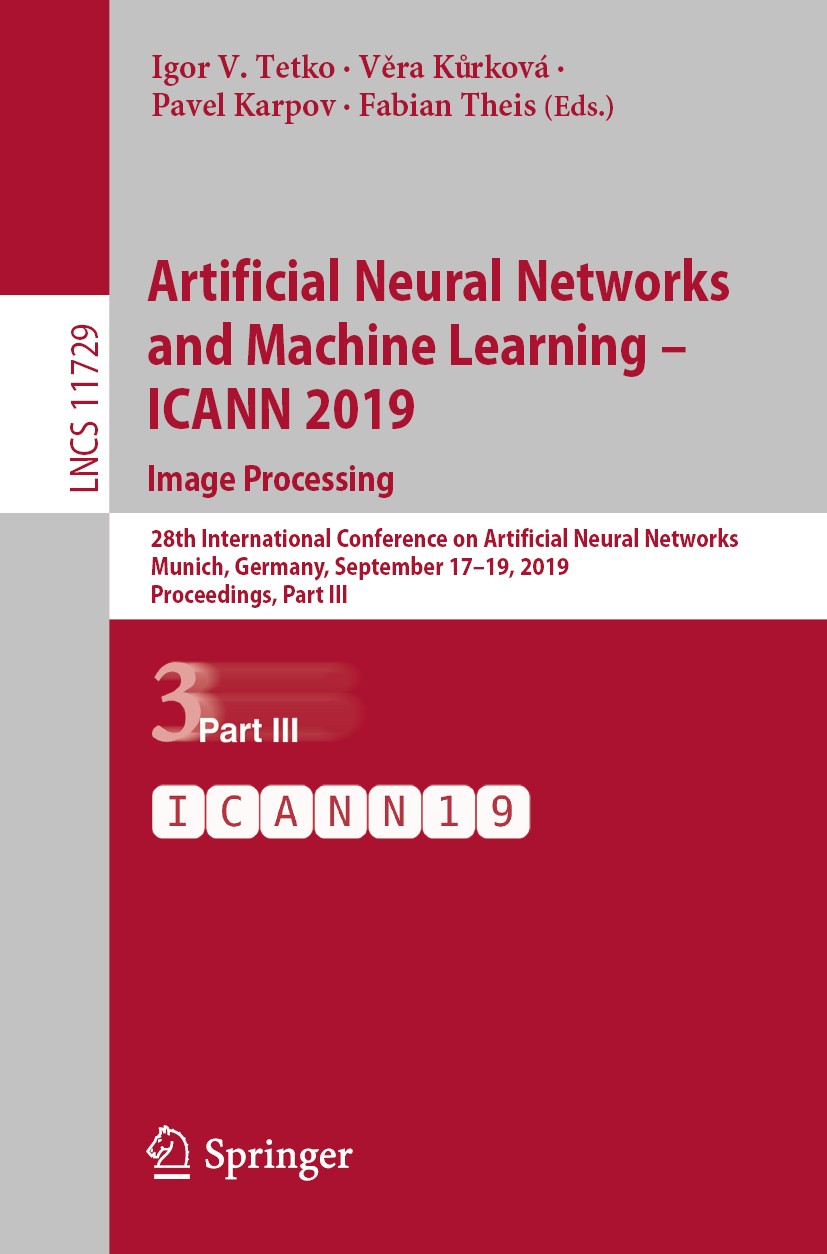 | 影响因子 | The proceedings set LNCS 11727, 11728, 11729, 11730, and 11731 constitute the proceedings of the 28th International Conference on Artificial Neural Networks, ICANN 2019, held in Munich, Germany, in September 2019. The total of 277 full papers and 43 short papers presented in these proceedings was carefully reviewed and selected from 494 submissions. They were organized in 5 volumes focusing on theoretical neural computation; deep learning; image processing; text and time series; and workshop and special sessions. . | Pindex | Conference proceedings 2019 |
The information of publication is updating
|
|